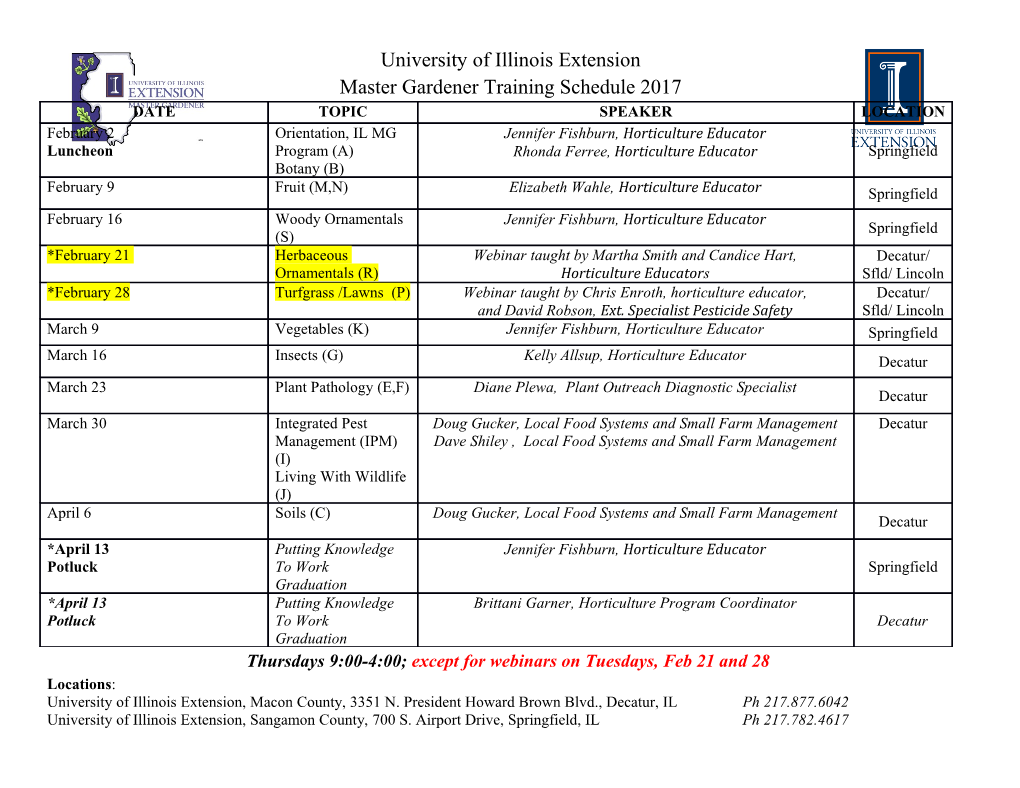
Generating sparse and selective third-order responses in the olfactory system of the fly Sean X. Luoa, Richard Axela,b,c,1, and L. F. Abbotta,d,1 aDepartment of Neuroscience, bDepartment of Biochemistry and Molecular Biophysics, cHoward Hughes Medical Institute, and dDepartment of Physiology and Cellular Biophysics, Columbia University, New York, NY 10032 Contributed by Richard Axel, April 28, 2010 (sent for review March 4, 2010) In the antennal lobe of Drosophila, information about odors is characteristics of LHNs and KCs lead us to consider the impli- transferred from olfactory receptor neurons (ORNs) to projection cations of the ORN-to-PN transformation with respect to two neurons (PNs), which then send axons to neurons in the lateral horn different downstream tasks: odor discrimination in the lateral of the protocerebrum (LHNs) and to Kenyon cells (KCs) in the mush- horn and general-purpose representation of a wide variety of room body. The transformation from ORN to PN responses can be odors in the mushroom body. We begin by discussing how model described by a normalization model similar to what has been used PN responses are constructed, then show how LHN and KC in modeling visually responsive neurons. We study the implications responses with the desired properties can be generated, and fi- of this transformation for the generation of LHN and KC responses nally analyze the roles played by different elements of the model. under the hypothesis that LHN responses are highly selective and therefore suitable for driving innate behaviors, whereas KCs pro- Results vide a more general sparse representation of odors suitable for We build our third-order neuron models by taking measured forming learned behavioral associations. Our results indicate that ORN firing rates (19, 20) (Fig. 1A) and then determining the the transformation from ORN to PN firing rates in the antennal lobe corresponding PN responses on the basis of an experimental equalizes the magnitudes of and decorrelates responses to differ- characterization of the transformations occurring within the an- ent odors through feedforward nonlinearities and lateral suppres- tennal lobe (see below) (21, 22). Finally, we use the computed PN sion within the circuitry of the antennal lobe, and we study how responses to drive model LHNs and KCs. To model the responses these two components affect LHN and KC responses. of LHNs or KCs, the computed firing rate for each PN in re- sponse to a particular odor is multiplied by a weight representing antennal lobe | decorrelation | lateral horn | normalization | olfaction the strength of a synapse from that PN onto the third-order neuron being modeled. The weighted PN rates are then summed, fi n the olfactory system, as in other sensory systems, signals from and the total is compared to a xed threshold (23) to determine Iprimary receptors are processed and transformed before being whether or not the third-order neuron generates a response to relayed to higher brain areas. In Drosophila melanogaster, olfactory that odor. Although selectivity is likely to arise from some form of receptor neurons (ORNs) located in the antennae and maxillary input summation and thresholding, the computations performed palps synapse onto projection neurons (PNs) within the glomeruli by neurons in the lateral horn and the mushroom body are un- of the antennal lobes (Fig. S1). ORNs expressing a given receptor doubtedly more complex than those of our model. Using a mini- converge on an anatomically invariant glomerulus. PNs innervate mal model allows us to identify the problems that more complex a single glomerulus and thus receive their primary input from cellular and circuit mechanisms must solve, and it allows us to ORNs expressing the same olfactory receptor (1), although cross- focus on the role of the antennal lobe circuitry. glomerular interactions mediated by interneurons are also present Transformation from ORN to PN Firing Rates. The transformation (2–5). The PNs send their axons to two distinct regions of the fly from ORN to PN responses consists of two functional compo- brain, the lateral horn and the mushroom body. By constructing nents: a feedforward nonlinearity and lateral suppression. The models of neurons in these regions, we study the effects of the relationship between the firing rate of an ORN and that of a PN transformations arising from circuitry within the antennal lobe on is nonlinear with strong saturation at high ORN rates (21), a re- the capacity of neurons in the lateral horn and the mushroom body sult due at least in part to short-term depression at ORN-PN to represent and discriminate odors. synapses (24). We refer to this transformation as a feedforward Any functional interpretation of the transformation from ORN nonlinearity, although lateral circuitry may also contribute to to PN responses depends on the nature of the processing being this effect. This nonlinearity was described using an exponential performed by the third-order neurons that receive PN input. The function, but it can also be fit by an equation of the form lateral horn and mushroom body appear to be involved in dif- r ¼ R r1:5 =ðσ1:5 þ r1:5 Þ, where r and r are the firing ferent forms of sensory processing. The lateral horn is believed to PN max ORN ORN PN ORN rates of a corresponding PN and ORN pair, and Rmax and σ are be important in generating innate behaviors (6), including those constants. The advantage of using this latter form is that it fits in elicited by pheromones (7). PN projections to lateral horn neu- well with more recent work in which the effects of lateral sup- rons (LHNs) are spatially stereotyped (8, 9) and clustered (10), pression have been incorporated (22). Lateral suppression, which “ ” suggesting that circuits in this region may be hardwired for the is the dominant effect of ORNs that do not drive a given PN di- fi detection of speci c odors that elicit innate behavioral responses. rectly, can be described by adding a term proportional to the sum The mushroom body is implicated in decision making (11) and in the formation of associative memories (12, 13). Both calcium imaging (14) and electrophysiology (15) indicate that the re- Author contributions: S.X.L., R.A., and L.F.A. designed research, performed research, con- sponses of Kenyon cell (KCs) in the mushroom body are sparse, tributed analytic tools, analyzed data, and wrote the paper. with each odorant eliciting responses in a few percent of the KCs The authors declare no conflict of interest. and individual KCs responding to a small number of tested Freely available online through the PNAS open access option. odorants. Electrophysiological experiments suggest that con- 1To whom correspondence may be addressed. E-mail: [email protected] or lfa2103@ nections from PNs to Kenyon cells may be random (16, 17), al- columbia.edu. fi NEUROSCIENCE though zonal speci city of PN projections and KC dendrites in This article contains supporting information online at www.pnas.org/lookup/suppl/doi:10. the mushroom bodies has been reported (10, 18). The distinct 1073/pnas.1005635107/-/DCSupplemental. www.pnas.org/cgi/doi/10.1073/pnas.1005635107 PNAS | June 8, 2010 | vol. 107 | no. 23 | 10713–10718 Downloaded by guest on September 25, 2021 A B odors that elicit stronger total responses (large sPN). This prob- 300 lem can be avoided if the magnitudes of PN responses are 2 2 4 250 4 ring Fi te Ra (H z) equalized, meaning that the total activity across the population 6 6 8 8 200 of PNs (i.e., sPN) is roughly the same for each odor. We use the 10 10 12 12 150 term equalization, rather than normalization, because normali- PN Index 14 14 16 16 100 zation has been used to refer to the entire transformation of ORN Receptor 18 18 Eq. 1 in the visual system literature where such a transformation 20 20 50 20 40 60 80 100 20 40 60 80 100 was first proposed and has been used extensively (25, 26). The 0 Odor Index Odor Index transformation of Eq. 1 has a strong magnitude-equalizing effect; C D E the sum of ORN rates (sORN, Fig. 1C) is much more variable across odors than the sum over PN rates (sPN, Fig. 1E). To iso- 2.5 ORN NL PN late the origin of this equalization of the response magnitude, we 2 also computed sPN when lateral suppression was eliminated by 1.5 setting the parameter m in Eq. 1 to zero. The results indicate that 1 much, but not all, of the response magnitude equalization arises from the feedforward nonlinearity (Fig. 1D). 0.5 firing rates/avg Selectivity is also difficult to achieve if the patterns of PN ac- 0 0 50 100 0 50 100 0 50 100 tivation across odors are too similar, that is, too highly correlated. Odor We performed a principal component analysis (PCA) of the ORN and computed PN firing rates to compare their degree of correlation. For totally correlated data, a single principal compo- F 50 G H nent accounts for 100% of the data variance, whereas for com- 40 pletely uncorrelated data, all of the components account for an 30 equal percentage of the variance. For the olfactory responses, >40% 20 of the ORN response variance is accounted for by a single prin- % Variance cipal component projection, indicating a high degree of correla- 10 tion (Fig. 1F). The maximal principal component projection for 0 0 10 20 0 10 20 0 10 20 the PN responses accounts for only 15% of the total variance PC component (Fig. 1H), so PNs are significantly decorrelated by the trans- formation of Eq. 1.
Details
-
File Typepdf
-
Upload Time-
-
Content LanguagesEnglish
-
Upload UserAnonymous/Not logged-in
-
File Pages6 Page
-
File Size-