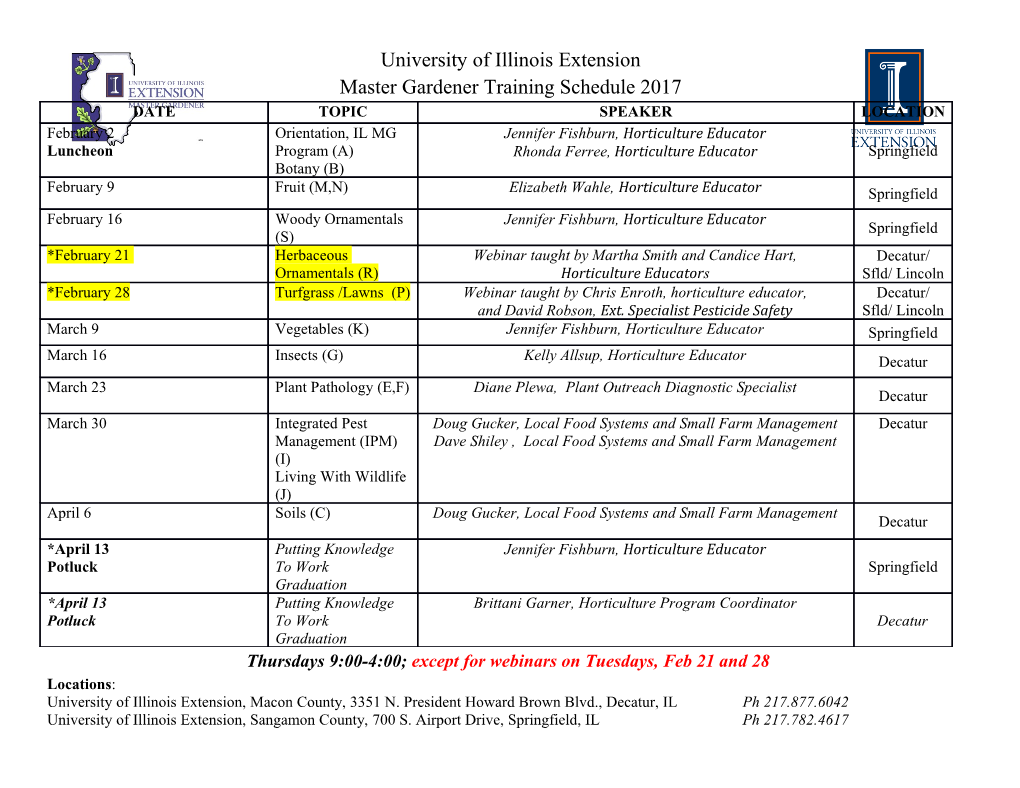
Lecture Notes for 1st Year Ph.D. Game Theory∗ Navin Kartik† 1 Introduction Game theory is a formal methodology and a set of techniques to study the interaction of rational agents in strategic settings. ‘Rational’ here means the standard thing in economics: maximizing over well-defined objectives; ‘strategic’ means that agents care not only about their own actions, but also about the actions taken by other agents. Note that decision theory — which you should have seen at least a bit of last term — is the study of how an individual makes decisions in non-strategic settings; hence game theory is sometimes also referred to as multi-person decision theory. The common terminology for the field comes from its putative applications to games such as poker, chess, etc.1 However, the applications we are usually interested in have little directly to do with such games. In particular, these are what we call “zero-sum” games in the sense that one player’s loss is another player’s gain; they are games of pure conflict. In economic applications, there is typically a mixture of conflict and cooperation motives. 1.1 A (Very!) Brief History Modern game theory as a field owes much to the work of John von Neumann. In 1928, he wrote an important paper on two-person zero-sum games that contained the famous Min- imax Theorem, which we’ll see later on. In 1944, von Neumann and Oscar Morgenstern published their classic book, Theory of Games and Strategic Behavior, that extended the work on zero-sum games, and also started cooperative game theory. In the early 1950’s, John Nash made his seminal contributions to non-zero-sum games and started bargaining ∗Last updated: March 11, 2009. These notes draw upon various published and unpublished sources, including notes by Vince Crawford, David Miller, and particularly those by Doug Bernheim. Thanks to David Miller and former and current students for comments. †[email protected]. Please feel free to send me suggestions, corrections, typos, etc. 1Ironically, game theory actually has limited prescriptive advice to offer on how to play either chess or poker. For example, we know that chess is “solvable” in a sense to be made precise later, but nobody actually knows what the solution is! This stems from the fact that chess is simply too complicated to “solve” (at present); this is of course why the best players are said to rely just as much on their intuition or feel as much as logic, and can defeat powerful computers. 1 theory. In 1957, Robert Luce and Howard Raiffa published their book, Games and De- cisions: Introduction and Critical Survey, popularizing game theory. In 1967–1968, John Harsanyi formalized methods to study games of incomplete information, which was crucial for widening the scope of applications. In the 1970s, there was an explosion of theoretical and applied work in game theory, and the methodology was well along its way to its current status as a preeminent tool in not only economics, but other social sciences too. 1.2 Non-cooperative Game Theory Throughout this course, we will focus on noncooperative game theory, as opposed to co- operative game theory. All of game theory describes strategic settings by starting with the set of players, i.e. the decision-makers. The difference between noncooperative and cooperative game theory is that the former takes each player’s individual actions as primi- tives, whereas the latter takes joint actions as primitives. That is, cooperative game theory assumes that binding agreements can be made by players within various groups and players can communicate freely in order to do so. We will take the noncooperative viewpoint that each player acts as an individual, and the possibilities for agreements and communication must be explicitly modeled. Except for brief discussions in Appendix A of Chapter 18 and parts of Chapter 22, Mas-Colell, Whinston, and Green (1995, hereafter, MWG) does not deal with cooperative game theory either. For an excellent introduction, see Chapters 13-15 in Osborne and Rubinstein (1994). 2 Strategic Settings A game is a description of a strategic environment. Informally, the description must specify who is playing, what the rules are, what the outcomes are depending on any set of actions, and how players value the various outcomes. Example 1. (Matching Pennies version A) Two players, Anne and Bob. Simultane- ously, each picks Heads or Tails. If they pick the same, Bob pays Anne $2; if they pick different, Anne pays Bob $2. Example 2. (Matching Pennies version B) Two players, Anne and Bob. First, Anne picks either Heads or Tails. Upon observing her choice, Bob then picks either Heads or Tails. If they pick the same, Bob pays Anne $2; if they pick different, Anne pays Bob $2. Example 3. (Matching Pennies version N) Two players, Anne and Bob. Simultane- ously, they pick either Heads or Tails. If they pick different, then they each receive $0. If they pick the same, they wait for 15 minutes to see if it rains outside in that time. If it does, they each receive $2 (from God); if it does not rain, they each receive $0. Assume it rains with 50% chance. 2 In all the above examples, implicitly, players value money in the canonical way and are risk-neutral. Notice that Examples 1 and 2 are zero-sum games — whatever Anne wins, Bob loses, and vice-versa. Example 3 is not zero-sum, since they could both win $2. In fact, it is a particular kind of coordination game. Moreover, it is also a game that involves an action taken by “nature” (who decides whether it rains or not). 2.1 Extensive Form Representation • Work through extensive form representation of the examples first. Let us now be more precise about the description of a game. Definition 1. An extensive form game is defined by a tuple ΓE = {X , A,I,p,α, H, H, ι, ρ, u} as follows: 1. A finite set of I players. Denote the set of players as I = {0, 1,...,I}. Players 1,...,I are the “real” players; player 0 is used as an “auxiliary” player, nature. 2. A set of nodes, X .2 3. A function p : X → X ∪ {∅} specifying a unique immediate predecessor of each node 3 x such that p(x) is the empty-set for exactly one node, called the root node, x0. (a) The immediate successors of node x are defined as s(x)= {y ∈X : p(y)= x}. (b) By iterating the functions p and s, we can find all predecessors and successors of any node, x, which we denote P (x) and S(x) respectively. We require that that P (x) ∩ S(x)= ∅, i.e. no node is both a predecessor and a successor to any other node. (c) The set of terminal nodes is T = {x ∈X : s(x)= ∅}. 4. A set of actions, A, and a function α : X \ {x0}→A that specifies for each node x 6= x0, the action which leads to x from p(x). We require that α be such that if distinct x′,x′′ ∈ s(x), then α(x′) 6= α(x′′). That is, from any node, each action leads to a unique successor. The set of available actions at any node, x, is denoted ′ c(x)= {α(x )}x′∈s(x). 5. A collection of information sets, H, that forms a partition of X ,4 and a function H : X →H that assigns each decision node into an information set. We require that if y ∈ P (x) then H(x) 6= H(y); that is, no node can be in the same information set 2Nodes are typically drawn as small solid circles, but note fn. 3. 3 The root node is typically drawn as a small hollow circle. 4Recall that a partition is a set of mutually exclusive and exhaustive subsets. 3 as any of its predecessors. We also require that c(x) = c(x′) if H(x) = H(x′); that is, two nodes in the same information set have the same set of available actions. It is therefore meaningful to write C(H)= {a ∈A : a ∈ c(x) ∀x ∈ H} for any information set H ∈H as the set of choices available at H. 6. A function ι : H→ I assigning the player (possibly nature) to move at all the decision nodes in any information set. This defines a collection of information sets that any player i moves at, Hi ≡ {H ∈H : i = ι(H)}. 5 7. For each H ∈ H0, a probability distribution ρ(H) on the set C(H). This dictates nature’s moves at each of its information sets. 8. u = (u1,...,uI ) is a vector of utility functions such that for each i = 1,...,I, ui : T → R is a (vNM) payoff function that represents (expected utility) preferences for i over terminal nodes. Keep in mind that when drawing game trees, we use dotted lines between nodes (Kreps) or ellipses around nodes (MWG) to indicate nodes that fall into the same informa- tion set. • Work through examples of what the definition of an extensive form game rules out. To avoid technical complications, we restrict attention the formal definition above to finite games which satisfy the following property. Assumption 1. The set of nodes, X , is finite. Remark 1. If X is finite, then even if the set of actions, A, is infinite, there are only a finite number of relevant actions; hence without loss of generality, we can take A as finite if X is finite. At various points, we will study infinite games (where the number of nodes is in- finite); the extension of the formal concept of a game to such cases will be intuitive and straightforward.
Details
-
File Typepdf
-
Upload Time-
-
Content LanguagesEnglish
-
Upload UserAnonymous/Not logged-in
-
File Pages89 Page
-
File Size-