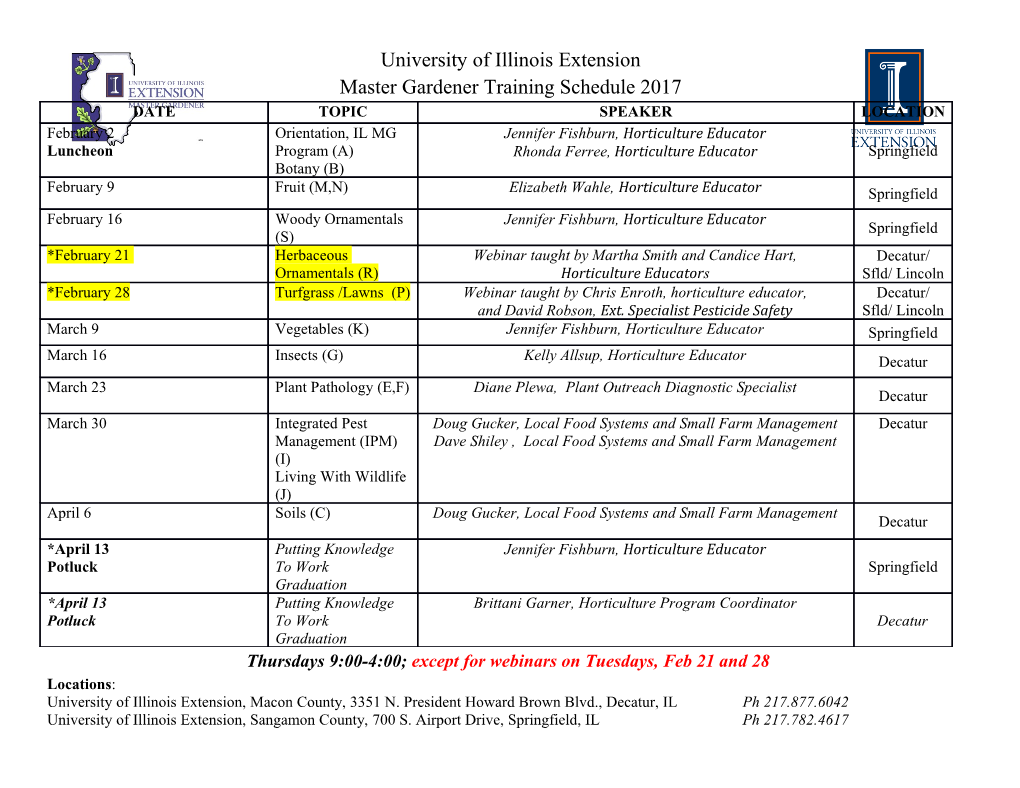
MACHINE LEARNING FOR INTELLIGENT CONTROL: APPLICATION OF REINFORCEMENT LEARNING TECHNIQUES TO THE DEVELOPMENT OF FLIGHT CONTROL SYSTEMS FOR MINIATURE UAV ROTORCRAFT A thesis submitted in partial fulfilment of the requirements for the Degree of Master of Engineering in Mechanical Engineering in the University of Canterbury by Edwin Hayes University of Canterbury 2013 Abstract This thesis investigates the possibility of using reinforcement learning (RL) techniques to create a flight controller for a quadrotor Micro Aerial Vehicle (MAV). A capable flight control system is a core requirement of any unmanned aerial vehicle. The challenging and diverse applications in which MAVs are destined to be used, mean that consider- able time and effort need to be put into designing and commissioning suitable flight controllers. It is proposed that reinforcement learning, a subset of machine learning, could be used to address some of the practical difficulties. While much research has delved into RL in unmanned aerial vehicle applications, this work has tended to ignore low level motion control, or been concerned only in off-line learning regimes. This thesis addresses an area in which accessible information is scarce: the performance of RL when used for on-policy motion control. Trying out a candidate algorithm on a real MAV is a simple but expensive proposition. In place of such an approach, this research details the development of a suitable simulator environment, in which a prototype controller might be evaluated. Then inquiry then proposes a possible RL-based control system, utilising the Q-learning algorithm, with an adaptive RBF-network providing function approximation. The operation of this prototypical control system is then tested in detail, to determine both the absolute level of performance which can be expected, and the effect which tuning critical parameters of the algorithm has on the functioning of the controller. Performance is compared against a conventional PID controller to maximise the usability of the results by a wide audience. Testing considers behaviour in the presence of disturbances, and run-time changes in plant dynamics. Results show that given sufficient learning opportunity, a RL-based control system performs as well as a simple PID controller. However, unstable behaviour during learning is an issue for future analysis. Additionally, preliminary testing is performed to evaluate the feasibility of implementing RL algorithms in an embedded computing environment, as a general requirement for a MAV flight controller. Whilst the algorithm runs successfully in an embedded context, observation reveals further development would be necessary to reduce computation time to a level where a controller was able to update sufficiently quickly for a real-time motion control application. In summary, the study provides a critical assessment of the feasibility of using RL algorithms for motion control tasks, such as MAV flight control. Advantages which merit interest are exposed, though practical considerations suggest at this stage, that such a control system is not a realistic proposition. There is a discussion of avenues which may uncover possibilities to surmount these challenges. This investigation will prove useful for engineers interested in the opportunities which reinforcement learning techniques represent. i ii Contents 1 Introduction 1 1.1 Motivation . 1 1.1.1 UASs, MAVs & Autopilots . 1 1.1.2 Issues with Traditional Control Systems . 1 1.1.3 Possible Application of Machine Learning . 2 1.1.4 Similar Research & Objectives . 3 1.2 Outline . 3 1.2.1 Problem Domain . 3 1.2.2 Thesis Summary . 4 1.2.3 Limitations . 4 2 Background & Literature 5 2.1 Background of Machine Learning in General . 5 2.2 Background of Reinforcement Learning . 6 2.2.1 About Reinforcement Learning . 6 2.2.2 About Q-Learning . 7 2.2.3 About Eligibility Traces . 8 2.2.4 Q-Learning Function Estimation via ARBFN . 8 2.3 Reinforcement Learning for UAV Platforms . 9 2.3.1 Remaining Questions . 11 2.4 A Note on Terminology . 11 3 Design of Micro Aerial Vehicles 13 3.1 About Quadrotor MAVs . 13 3.1.1 General Scheme . 13 3.1.2 Basic Dynamics . 14 3.1.3 A Typical Quadrotor UAV . 15 3.1.4 Test Stand . 15 4 Software Design 19 4.1 Computing Software Technologies . 19 4.1.1 Java . 19 4.1.2 Reinforcement Learning Library (Teachingbox) . 20 4.1.3 J3D Robot Simulator (Simbad) . 21 4.2 Quadrotor UAV Simulator . 22 4.2.1 Encoding of Simulator State . 22 4.2.2 Freedom During Testing . 23 4.2.3 Encoding of Controller State . 24 4.2.4 Throttle Signal Mixing . 25 4.2.5 Simulator Implementation . 26 4.3 Controllers . 29 iii 4.3.1 Traditional PID Controller . 30 4.3.2 Q-Learning Controller . 31 4.4 Integration of Components . 35 4.4.1 Modifications Required . 35 4.4.2 Typical Call Chain . 37 5 Performance of PID Controller 39 5.1 Experimental Details . 39 5.1.1 Test Procedure . 39 5.1.2 Performance Metrics . 40 5.2 Results . 40 5.2.1 A First Attempt . 40 5.2.2 Other Controller Gains . 42 5.2.3 Effects of Disturbance . 45 5.2.4 Handling Changes in Dynamics . 46 6 Performance of Q-Learning Based Controller 53 6.1 Experimental Details . 53 6.1.1 Test Procedure . 53 6.1.2 Performance Metrics . 54 6.1.3 The Term `Stability' . 54 6.2 Tuning Parameters . 55 6.2.1 Initial Baseline . 55 6.2.2 Varying Learning Rate α ........................... 60 6.2.3 Varying Discount Rate γ ........................... 66 6.2.4 Varying Eligibility Trace Decay Rate λ .................... 73 6.2.5 Varying Velocity Reward Gain Kθ_ ...................... 81 6.2.6 Varying Displacement ARBFN Resolution σθ . 92 6.2.7 Varying Velocity ARBFN Resolution σθ_ . 100 6.2.8 Varying Greediness ..............................107 6.2.9 Final Baseline . 112 6.3 Effects of Disturbance . 118 6.4 Handling Changes in Dynamics . 121 6.4.1 Without Disturbances . 121 6.4.2 With Disturbances . 127 6.5 Inspection of Q-Functions and Policies . 133 6.6 Assessment of Results . 137 7 Computational Considerations 139 7.1 Introduction . 139 7.1.1 Importance of Computational Performance . 139 7.1.2 Practical Considerations . 140 7.2 Computing Hardware Technologies . 140 7.2.1 Desktop Computer . 140 7.2.2 Embedded ARM Computer . 141 7.3 Performance Comparison . 142 7.3.1 Performance Metrics . 142 7.3.2 Experimental Procedure . 143 7.3.3 Expectations . 143 7.3.4 Results . 144 8 Conclusions 147 iv 8.1 Considerations for Parameter Selection . 147 8.2 Viability for Practical Use . 148 8.3 Further Expansion & Future Work . 149 8.3.1 Longer Simulations . 150 8.3.2 More Comprehensive Test Regime . 150 8.3.3 Testing on Test Platform . 150 8.3.4 Free Rotation . 151 8.3.5 Incorporating Translational Control . 151 8.3.6 Adding Multiple Actions & Continuous Actions . 151 8.3.7 Hybrid Approaches . 152 8.4 Closing Remarks . 153 A Additional Q-Function and Policy Plots 155 Bibliography 165 v vi List of Figures 3.1 Illustrative diagram of simplified model of forces on quadrotor MAV. 14 3.2 Illustrative diagram of principles for movement of a quadrotor MAV. 16 3.3 The Droidworx CX-4, a fairly typical quadrotor MAV intended for industrial applications. 16 3.4 The UAV test stand. The X/Y translational arm is denoted Details A and B. The translational Z slide is denoted Detail C, and the rotational gimbal frame is denoted Detail D. 18 4.1 Diagram showing the global/earth reference frame (denoted E) and the base/vehicle reference.
Details
-
File Typepdf
-
Upload Time-
-
Content LanguagesEnglish
-
Upload UserAnonymous/Not logged-in
-
File Pages185 Page
-
File Size-