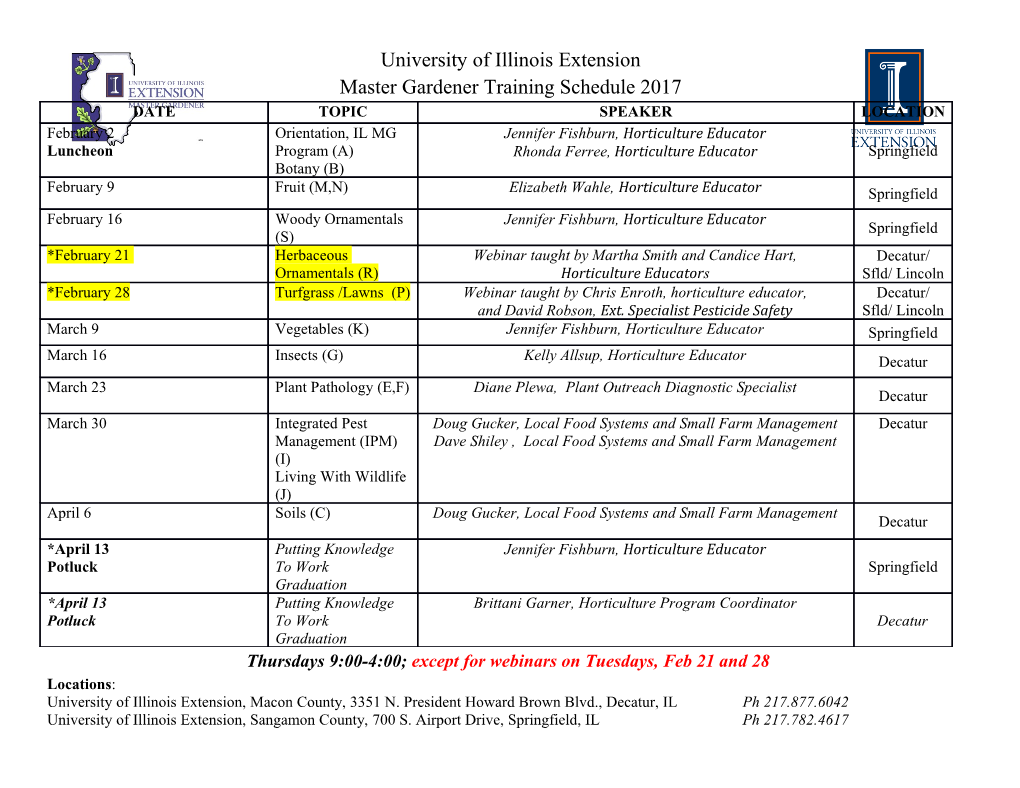
How to Not Get Caught When You Launder Money on Blockchain? Cuneyt G. Akcora1, Sudhanva Purusotham2, Yulia R. Gel2 Mitchell Krawiec-Thayer3, Murat Kantarcioglu2 1University of Manitoba, 2 UT Dallas, 3 Monero Research Lab 65 Chancellor Circle Winnipeg, MB, Canada R3T 2N2 [email protected], fSudhanva.Purushotham, ygl, [email protected], [email protected] Abstract transactions and circumvent existing AI-based money laun- dering techniques. Our insights, however, are intended not to The number of blockchain users has tremendously grown in assist criminals to obfuscate their malicious activities but to recent years. As an unintended consequence, e-crime trans- motivate the AI community for further research in this area actions on blockchains has been on the rise. Consequently, public blockchains have become a hotbed of research for de- of critical societal importance and, as a result, to advance veloping AI tools to detect and trace users and transactions the state of art methodology in the AI-based cryptocurrency that are related to e-crime. money laundering detection. We argue that following a few select strategies can make In the remainder of this paper, we first identify tech- money laundering on blockchain virtually undetectable with niques that can be used by criminals to launder money on most of the currently existing tools and algorithms. As a re- blockchain and then empirically show why these techniques sult, the effective combating of e-crime activities involving would be effective in hiding patterns used by potential AI cryptocurrencies requires the development of novel analytic models. methodology in AI. 2 Related Work 1 Introduction The success of Bitcoin (Nakamoto 2008) has encouraged Cryptocurrencies have emerged as an important rapidly hundreds of similar digital coins (Tschorsch and Scheuer- evolving financial tool that allows for cross-border transac- mann 2016). The underlying Blockchain technology has tions without requiring a central trusted party. Unfortunately, been adopted in many applications. With this rapidly in- the pseudo-anonymity of cryptocurrencies also continues creasing activity, there have been numerous studies analyz- to facilitate various forms of fraudulent activities. Sellers ing the blockchain technology from different perspectives. and buyers of illicit goods are matched with ease, and pay- The earliest results aimed at tracking the transaction net- ments are quickly executed. However, contrary to fiat cur- work to locate coins used in illegal activities, such as money rencies, where law enforcement agencies have access to a laundering and blackmailing (Androulaki et al. 2013; Ober, wide arsenal of analytic methods for investigation of vari- Katzenbeisser, and Hamacher 2013). These findings are ous criminal activities, from money laundering to drug deal- known as the taint analysis (Di Battista et al. 2015). ership to human trafficking, methods for detecting crime on Bitcoin provides pseudo-anonymity: although all transac- the blockchain are yet in their infancy (Foley, Karlsen, and tions are public by nature, user identification is not required Putnin¸sˇ 2019; Zhou et al. 2020). As a result, large amounts to join the network. Mixing schemes (Maxwell 2013; Ruff- of illegal transactions with cryptocurrencies remain uniden- ing, Moreno-Sanchez, and Kate 2014) exist to hide the flow tified. Given the complexity of the transaction patterns used of coins in the network. However, as shown in (Meiklejohn arXiv:2010.15082v1 [cs.CR] 22 Sep 2020 by illicit networks, simple heuristic-based techniques do not et al. 2013), some Bitcoin payments can be traced. As a allow for reliable detection of suspicious blockchain activi- result, obfuscation efforts (Narayanan and Moser¨ 2017) by ties and efficient implementation of Anti-Money Laundering malicious users have become increasingly sophisticated. (AML) rules. In ransomware analysis, Montreal (Paquet-Clouston, To address this challenge, there have been recently pro- Haslhofer, and Dupont 2019), Princeton (Huang and McCoy posed various AI techniques, e.g., (Deng et al. 2019; Ak- 2018) and Padua (Conti, Gangwal, and Ruj 2018) studies cora et al. 2020; Lee et al. 2019). In addition, in September have analyzed networks of cryptocurrency ransomware and 2020 the U.S. Government solicited proposals for AI solu- found that hacker behavior can aid identification of undis- tions in detecting money laundering activities on cryptocur- closed ransomware payments. Datasets of these three studies rencies (Franceschi-Bicchierai 2020). are publicly available. In this paper, we identify multiple techniques that can be Early studies in ransomware detection use decision rules used by the money launderers to hide illicit cryptocurrency on amounts and times of known ransomware transac- tions to locate undisclosed ransomware (CryptoLocker) pay- Copyright c 2021, All rights reserved. ments (Liao et al. 2016). More recent studies are joint ef- forts between researchers and blockchain analytics compa- them. Once the ransomware is installed, it communicates nies (Huang and McCoy 2018; Weber et al. 2019). However, with a command and control center. Although earlier ran- these studies do not disclose features used in their AI mod- somware used hard-coded IPs and domain names, newer els. variants may use anonymity networks, such as TOR, to Darknet market vendor identification has been considered reach a hidden command and control server. In the case of by Tai, Soska, and Christin (2019) where features, such as asymmetric encryption, the encryption key is delivered to shared email addresses, are extracted from market profiles the victim’s machine. In some variants, the malware cre- and used in address classifiers. Lee et al. (2019) perform a ates the encryption key on the victim’s machine and deliv- taint analysis for addresses and track cryptocurrency pay- ers it to the command center. Once resources are locked or ments to online exchanges. We glean insights from both ar- encrypted, the ransomware displays a message that asks a ticles and create a set of rules that militate against such anal- certain amount of cryptocurrency to be sent to an address. ysis. This amount may depend on the number and size of the encrypted resources. 3 Sources of Dark Coins After payment, a decryption tool is delivered to the vic- 3.1 Darknet Markets tim. However, in some cases, such as with WannaCry, the Darknet markets are online sites that can only be accessed by ransomware contained a bug that made it impossible to iden- privacy-enhancing tools such as TOR and I2P (Lamy et al. tify who paid a ransomware amount. 2020). Markets allow vendors to create online stores and match buyers to the vendors. The merchandise range from 3.3 Undisclosed Transactions real passports to heroin and guns. The market employs an Dark coins may be received from undisclosed sales of goods escrow service where a buyer is given a one-time-use ad- or services. Typically, the coins are accrued to be spent on dress to pay for a good. After the payment, the market in- the blockchain without exchanging them for fiat currency. structs the vendor to mail the goods physically. Upon the Traditionally, a notable source of undisclosed finan- delivery (from abroad as well), the market releases coins to cial transactions has been the Hawala network which al- the vendor’s account, which can cash out the coins. The es- lows transferring money through personal connections glob- crow process can take around 6 days, and researchers found ally (El Qorchi et al. 2003). Bitcoin can be used to facilitate that in the early days (i.e., 2014) the release of coins could transfers between trustless hawalas as follows. John gives affect Bitcoin price (Janze 2017). The market needs to foster $100k USD to a hawala to transfer the equivalent amount trust between vendors and buyers to convince buyers to part to a location. A second hawala in the location receives bit- with their coins. However, occasionally, the market defrauds coins in its address and pays several dollars to John’s friend vendors and disappears with coins, or similarly, vendors de- Jim. Both hawala take a fee to allow the dollar transfer. John fraud buyers. pays both bitcoin and hawala transaction fees but avoids ver- Cryptocurrencies are ideal venues for Darknet markets ifying his identity or paying tax for the transferred amount. because payments can be made anonymously and received Hawalas undertake the risk of being discovered by financial anywhere in the world. Starting with the Silk Road in 2011, authorities. Darknet markets have been gathering ever-growing amounts where illicit goods, such as fake passports and guns, are 4 Cryptocurrency Transaction Models transacted for cryptocurrencies. Law enforcement has seized multiple Darknet markets, but the ease of setting up a new We follow the adversarial model in (Androulaki et al. market has turned this into a cat-and-mouse game. Typi- 2013) and observe the Bitcoin blockchain, denoted by tx- cally, Bitcoin and Monero are used for Darknet payments. Chain, for a period of time ∆t. In this period ∆t, n en- In multiple cases, the seized markets’ data have been made tities, U = a1; a2; : : : ; anu, send or receive transaction public (Paquet-Clouston, Decary-H´ etu,´ and Morselli 2018; outputs in txChain through a set of nα addresses: A = Branwen et al. 2015) (updated regularly) where each good a1; a2; : : : ; anα where nα ≥ nu. We assume that within is recorded with its buyer, price, and time information. Iden- ∆t, nT transactions have taken place as follows: T = tifying these payments on the Bitcoin blockchain is known T1(I1 ! O1);:::; Tn(InT ! OnT ) denotes a transaction as transaction fingerprinting (Fleder and Kester 2015). Dark- with sets of outputs and inputs, respectively. We will use net market vendors accrue big amounts of coins in multiple A(oi) to denote the bitcoin amount in an output oi 2 O. Set addresses (Tai, Soska, and Christin 2019). of addresses in an output is denoted with O:A.
Details
-
File Typepdf
-
Upload Time-
-
Content LanguagesEnglish
-
Upload UserAnonymous/Not logged-in
-
File Pages7 Page
-
File Size-