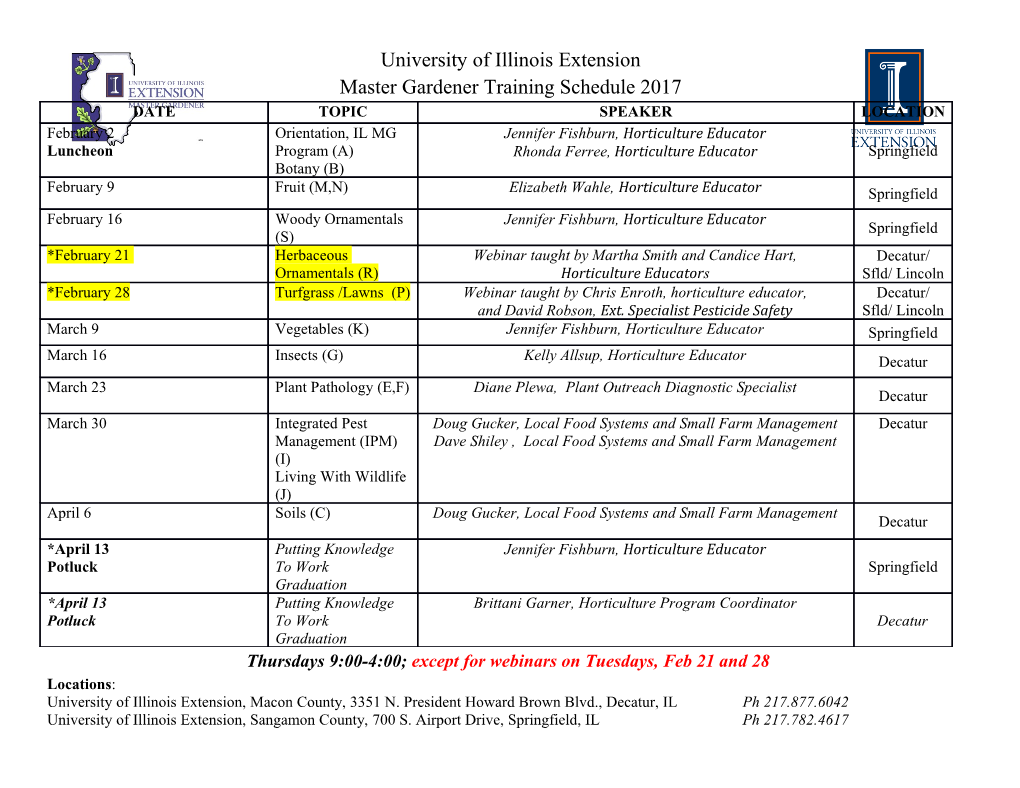
Nature Reviews Genetics | AOP, published online 15 June 2010; doi:10.1038/nrg2813 PROGRESS GENOME-WIDE ASSOCI ATI ON STUDIES considered benign; we note that inflation in λGC is proportional to sample size. New approaches to population If population stratification exists, it is important to distinguish between sub- stratification in genome-wide population differences that are due to recent genetic drift and those that arose from more association studies ancient population divergence11. In the case of genetic drift, dividing association statistics by λGC will provide a sufficient Alkes L. Price, Noah A. Zaitlen, David Reich and Nick Patterson correction for stratification. In the case of Abstract | Genome-wide association (GWA) studies are an effective approach ancient population divergence, markers with for identifying genetic variants associated with disease risk. GWA studies can unusual allele frequency differences that lie outside the expected distribution, which be confounded by population stratification — systematic ancestry differences could be caused by natural selection, make between cases and controls — which has previously been addressed by methods stratification a much more severe problem, that infer genetic ancestry. Those methods perform well in data sets in which and dividing association statistics by λGC is population structure is the only kind of structure present but are inadequate in likely to be inadequate. In the case of fam- data sets that also contain family structure or cryptic relatedness. Here, we ily structure or cryptic relatedness, dividing association statistics by λGC will generally review recent progress on methods that correct for stratification while produce the approximate null distribution, accounting for these additional complexities. although a refinement to the method may be needed when there is uncertainty in the (REF. 12) Genome-wide association (GWA) studies is a necessity in studies with family-based estimate of λGC . However, even if have identified hundreds of common vari- sample ascertainment, and there is increas- the appropriate null distribution is obtained, ants associated with disease risk or related ing evidence that cryptic relatedness may in general this approach will not maximize traits1 (see the National Human Genome occur in a wide range of data sets (see below). power to detect true associations. Other Research Institute (NHGRI) Catalog of Family-based association tests offer one poten- approaches to correcting for stratification, Published Genome-Wide Association tial solution for dealing with family structure. including approaches that also account for Studies). These studies have overcome the More recently, approaches using mixed models family structure and cryptic relatedness, are dangers of population stratification, which that incorporate the full covariance structure described below. can produce spurious associations if not across individuals have been proposed. properly corrected2–3. However, accounting Below, we review each of these methods, Inferring genetic ancestry for population structure is more challenging conduct simulations to evaluate their Structured association. Methods that explic- when family structure or cryptic relatedness performance, discuss stratification in the itly infer genetic ancestry generally provide is also present, and these limitations have specific context of low-frequency or rare an effective correction for population stratifi- motivated the development of new methods. variants and conclude with guidelines cation in data sets in which population struc- Spurious associations have occurred prima- and recommendations. ture is the only type of sample structure. In rily at markers with unusual allele frequency the structured association approach, samples differences among subpopulations2,4, so it is Detecting stratification are assigned to subpopulation clusters (pos- crucial that new methods aimed at correcting A widely used approach to evaluate whether sibly allowing fractional cluster membership) for stratification are evaluated at unusually confounding due to population stratifica- using a model-based clustering program such differentiated markers. tion exists is to compute the genomic con- as STRUCTURE13–14, and association statis- The prevailing paradigm in recent years trol λ (λGC), which is defined as the median tics are computed by stratifying by cluster has been to use genomic control to measure the χ2(1 degree of freedom) association statistic using a program such as STRAT15. The appli- extent of inflation due to population stratifi- across SNPs divided by its theoretical cability of this approach to large genome- cation or other confounders, and to correct median under the null distribution7–9. A wide data sets has historically been limited for stratification (if necessary) using methods value of λGC ≈ 1 indicates no stratification, by its high computational cost when allowing structured that infer genetic ancestry, such as whereas λGC > 1 indicates stratification or fractional cluster membership, but faster association or principal components analysis other confounders, such as family struc- model-based approaches for inferring popu- (PCA). A limitation of this strategy is that ture or cryptic relatedness (see below), or lation structure have recently been developed it fails to account for other types of sample differential bias10. P–P plots are a standard (such as the ADMIXTURE software)16. structure, such as family structure or cryptic tool for visualization of test statistics Thus, applying structured association to 5–6 (FIG. 1) relatedness . Modelling family structure . Values of λGC < 1.05 are generally both infer population structure and compute NATURE REVIEWS | GENETICS ADVANCE ONLINE PUBLICATION | 1 © 2010 Macmillan Publishers Limited. All rights reserved PROGRESS a No stratification b Stratification without unusually c Stratification with unusually differentiated markers differentiated markers 10 10 10 8 8 8 6 6 6 ed (–logP) ed (–logP) ed (–logP) 4 4 4 Observ Observ Observ 2 2 2 0 0 0 024602460246 Expected (–logP) Expected (–logP) Expected (–logP) Figure 1 | P–P plots for the visualization of stratification or other unusually differentiated markers: p-values exhibitNa modestture Revie genome-widews | Genetics confounders. The figure shows simulated P–P plots under three scenarios inflation. c | Stratification with unusually differentiated markers: p-values for genome-wide scans with no causal markers. a | No stratification: exhibit modest genome-wide inflation and severe inflation at a small p-values fit the expected distribution. b | Stratification without number of markers. association statistics in genome-wide data A common misconception is that AIMs information are immune to stratification31,32. sets is likely to become a practical approach. should be used to infer genetic ancestry even This approach performs favourably when genome-wide data are available, but in compared with previous family-based Principal components analysis. PCA is a fact the best ancestry estimates are obtained approaches31,32, but it places an upper bound tool that has been used to infer population using a large number of random markers. on the statistical power that can be extracted structure in genetic data for several decades, A limitation of the above methods is from the between-family component of the long before the era of GWA studies17–20. It that they do not model family structure or overall signal. This is because the trans- should be noted that top principal compo- cryptic relatedness. These factors may lead formed rank statistic cannot be more statis- nents do not always reflect population struc- to inflation in test statistics if they are not tically significant than one divided by the ture: they may reflect family relatedness19, explicitly modelled because samples that are number of samples. long-range linkage disequilibrium (LD) (due correlated are assumed to be uncorrelated. to, for example, inversion polymorphisms4) Although correcting for genetic ancestry and Mixed models 10 or assay artefacts . These effects can often then dividing by the residual λGC will restore Mixed models, which owe their roots to be eliminated by removing related samples, an appropriate null distribution, association applications in animal breeding, can model regions of long-range LD or low-quality statistics that explicitly account for family population structure, family structure and data, respectively, from the data used to structure or cryptic relatedness are likely to cryptic relatedness33,34. The basic approach compute principal components. In addition, achieve higher power owing to improved is to model phenotypes using a mixture PCA can highlight effects of differential bias weighting of the data. of fixed effects and random effects. Fixed that require additional quality control21. effects include the candidate SNP and Using top principal components as cov- Family-based association tests optional covariates, such as gender or age, ariates corrects for stratification in GWA Family-based studies, in which individuals whereas random effects are based on a studies21,22, and this can be done using are ascertained from family pedigrees, offer a phenotypic covariance matrix, which is software such as EIGENSTRAT. Like struc- unique solution to population stratification. modelled as a sum of heritable and non- tured association, PCA will appropriately Family-based association tests that focus heritable random variation (BOX 1). Mixed apply a greater
Details
-
File Typepdf
-
Upload Time-
-
Content LanguagesEnglish
-
Upload UserAnonymous/Not logged-in
-
File Pages5 Page
-
File Size-