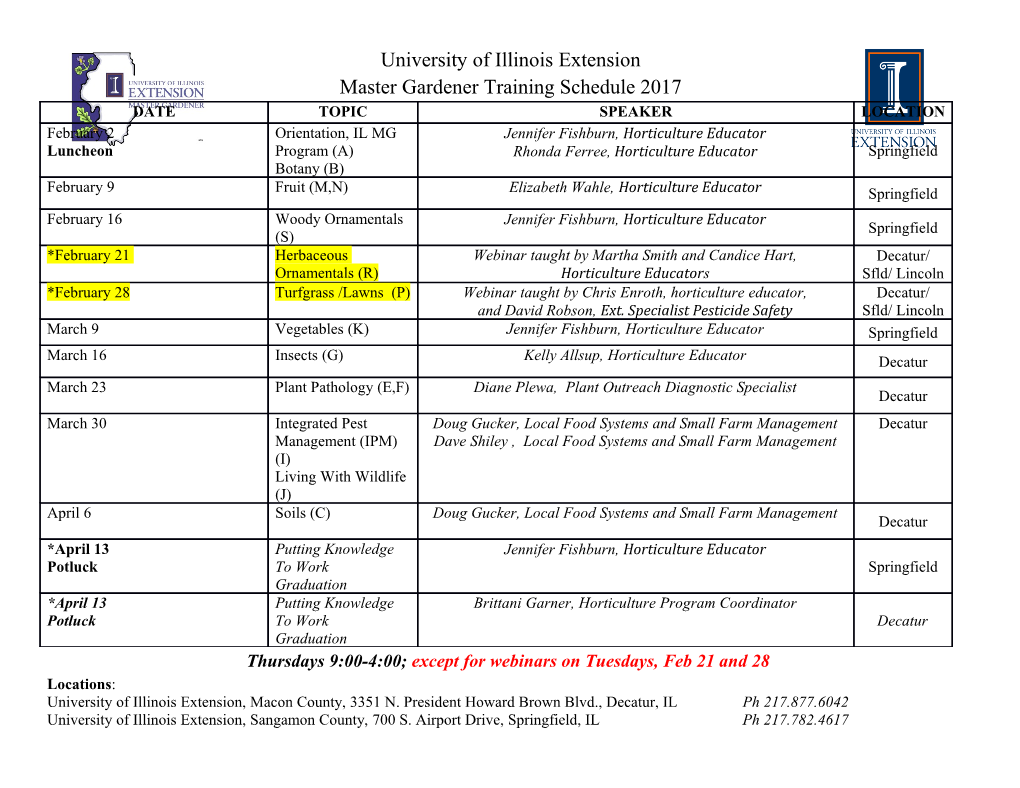
Automated Rip Current Detection with Region based Convolutional Neural Networks a a b a a Akila de Silva , Issei Mori , Gregory Dusek , James Davis and Alex Pang aUniversity of California, Santa Cruz, CA, United States bNOAA National Ocean Service, Silver Spring, MD, United States ARTICLEINFO ABSTRACT Keywords: This paper presents a machine learning approach for the automatic identification of rip currents with Rip Current Detection breaking waves. Rip currents are dangerous fast moving currents of water that result in many deaths Machine Learning by sweeping people out to sea. Most people do not know how to recognize rip currents in order to Image Processing avoid them. Furthermore, efforts to forecast rip currents are hindered by lack of observations to help Faster RCNN train and validate hazard models. The presence of web cams and smart phones have made video Temporal Smoothing and still imagery of the coast ubiquitous and provide a potential source of rip current observations. These same devices could aid public awareness of the presence of rip currents. What is lacking is a method to detect the presence or absence of rip currents from coastal imagery. This paper provides expert labeled training and test data sets for rip currents. We use Faster-RCNN and a custom temporal aggregation stage to make detections from still images or videos with higher measured accuracy than both humans and other methods of rip current detection previously reported in the literature. 1. Introduction Lifeguards are often trained to identify rip currents. However the majority of drownings occur on beaches with- Rip currents are the most significant safety risk to swim- out trained personnel [1,4]. Posted signs can provide a warn- mers along the coastlines of oceans, seas, and large lakes. [8, ing, but there is evidence that most people do not find exist- 7, 13]. The majority of beach goers do not know how to iden- ing signs helpful in actually identifying rip currents [6]. tify rip currents, and there is no robust and reliable location- Experts at the National Oceanic and Atmospheric Ad- independent method to identify them. Globally there are ministration (NOAA) use images and video to gather statis- thousands of drownings each year due to rip currents [23, tics about rip currents [19]. These data are supporting the 42]. A 20 year study by the US Lifesaving Association re- validation of a rip current forecast model to alert people to ports that 81.9% of the 37,000 beach rescues each year are potential hazards [20]. The most commonly used method to due to rip currents [7]. There has been no decline in the num- visualize rip currents from video is time averaging, summa- ber of associated drowning fatalities, despite warning signs rizing a video as a single image [28]. In [43] boosted cas- and educational material. cade of simple Haar like features, a machine learning tech- Rip currents are a well-studied ocean phenomenon [3, nique, was used to detect rip currents in time averaged im- 29, 31]. They are defined as strong and narrow channels of ages. However these time averages when manually assessed fast-moving water that flow towards the sea from beaches. can be misinterpreted. Furthermore, they are not readily When waves break, they form a “setup” or an increase in available nor interpretable by the average beachgoers, and mean water level. This setup can vary along a shoreline de- the process of averaging removes available information. pending on the amount or height of breaking waves. Rip cur- In recent years the coastal engineering community has rents form as water tends to flow alongshore from regions of successfully used deep neural networks to solve many prob- arXiv:2102.02902v1 [cs.CV] 4 Feb 2021 high setup (larger waves) to regions of lower setup (smaller lems. Classification problems such as classifying wave waves) where currents converge to form a seaward flowing breaking in infrared imagery [9], beach scene and other land- rip. The speed of seaward rips can be quite strong reaching scape classification [11], automated plankton image classifi- 2 m/s, faster than an Olympic swimmer. There are multiple cation [41] and ocean front recognition [36] were formulated factors that determine the location and strength of rips, such as deep learning problems using convolutional neural net- as bathymetry, wave height and direction, tide, and beach works. Furthermore, some regression problems such as opti- shape. Rip currents may either be transient or persistent in cal wave gauging [10], tracking remotely sensed waves [52], space and time. Rips that are frequently found at the same typhoon forecasting [33] were also solved using deep neu- location are usually indicative of a fairly stable bathymetric ral networks. In addition, generative adversarial networks, feature such as a sand bar or reef, or a hard structure such a type of deep neural networks, were used to improve the as rocky outcrop, jetty or pier. These bathymetric features quality of downscaling of ocean remote sensing data [18]. results in variations in wave breaking and setup leading to Object detection with deep neural networks is well channelized rip current flow. Transient or flash rips are inde- studied in the computer vision community. However most pendent of bathymetry and may move up or down the beach, benchmarks and research focus on detecting physical and may appear or disappear. objects with boundaries between what is and is not ORCID(s): an object [16, 22, 37]. Rip currents are ephemeral “objects” de Silva et al.: Preprint submitted to Elsevier Page 1 of 9 Automated rip current detection Figure 1: A collection of beach scenes, some of which contain dangerous rip currents. Unfortunately these “objects” do not have clear shape, and most people find them hard to identify. This paper describes a computer vision system with detection accuracy higher than both existing published methods and human observers. which are not observable in every frame, and amorphous released into the ocean and the dispersion observed [5, 14, without clearly defined boundaries even when observable. 15, 48]. Wave sensors, acoustic velocimeters, or current It is not obvious whether existing methods are applicable. profilers can be deployed at specific locations [21, 32, 34, Figure1 provides a set of examples, illustrating the difficulty 40]. Floating drifters with embedded GPS units have also of the problem. been used to measure currents [12, 13, 51]. These methods Our work is aimed at introducing this problem to the are costly, time consuming, require technical expertise and coastal engineering community, and showing that object de- are generally only applicable to highly localized instances tection methods are applicable. We gathered training data in time and space. These limitations severely hinder the ap- sets of rip currents and worked with experts at NOAA to plicability of such approaches to both public warnings and ensure that test data were labeled correctly. We use Faster- model validation. RCNN [50] with a custom temporal aggregation stage that Time averaged images are a routine method for analyzing allowed us to achieve detection accuracy that is higher than video in oceanic research, with 10 minutes being a common both humans and other methods of rip current detection pre- integration period [27, 28, 38, 47]. This method is popular viously reported in the literature. because averages often make identification of rip channels The contributions of this paper are: easier for the human eye. While these images are usually intended for human interpretation, Maryan et. al. apply ma- • Evidence that region based convolutional neural net- chine learning to recognize rip channels in time averaged im- works (CNN) approach for object detection is appli- ages [43]. Nelko also used time averaged images and noted cable to amorphous and ephemeral objects such as rip that prediction schemes developed at one beach location may currents not be directly applicable to another without some modifica- • Analysis showing rip current detection accuracy above tions [44]. In contrast to these works, the analysis in this pa- existing per suggests that object detection on individual frames out- published methods performs time averaged images. Dense optical flow [2, 30] has been used to detect rip • Data sets of rip current images and video for training currents in video [46]. This method is attractive since opti- and testing cal flow fields can be directly compared against ground truth The remainder of the paper is organized as follows. All flow fields obtained from in situ measurements [17]. Unfor- the related work is summarized in Section2. We discuss how tunately these methods are sensitive to camera perturbation, the data was collected in Section3. Our method is discussed and have difficulty in areas lacking textural information. The in4. Results are discussed in5. Limitations and discussion results in this paper suggests that object detection on individ- are in Section6. In Section7 we conclude our paper. And in ual frames outperforms previous optical flow based methods. AppendixA we provide the link to the supplementary mate- Certain kinds of rip currents are characterized by visible rials. sediment plumes. These can be segmented based on changes in coloration. For example, Liu et al, use thresholding in 2. Related Work HSV color space to detect rip currents [40]. Unfortunately, not all rip currents contain sediment plumes, and thus this Rip currents are most commonly studied using in situ method is not applicable to our data sets. techniques or instrumentation. Fluorescein dye is commonly Object detection in images is well studied in the com- de Silva et al.: Preprint submitted to Elsevier Page 2 of 9 Automated rip current detection With Rips Without Rips Figure 2: Examples drawn from the 2440 images we collected and labeled to build a training data set. Ground truth bounding boxes are shown in red.
Details
-
File Typepdf
-
Upload Time-
-
Content LanguagesEnglish
-
Upload UserAnonymous/Not logged-in
-
File Pages9 Page
-
File Size-