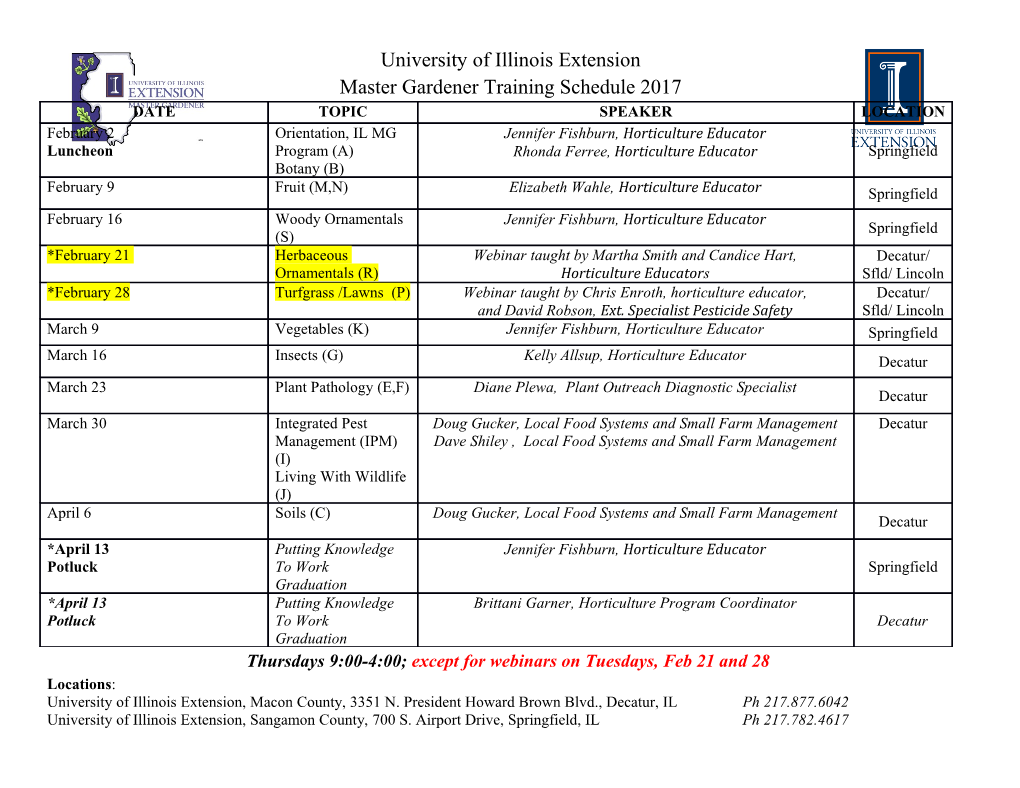
CLT Moments of Distributions Moments Lecture 12: Central Limit Theorem and CDFs Raw moment: 0 n µn = E(X ) Statistics 104 Central moment: 2 Colin Rundel µn = E[(X − µ) ] February 27, 2012 Normalized / Standardized moment: µn σn Statistics 104 (Colin Rundel) Lecture 12 February 27, 2012 1 / 22 CLT Moments of Distributions CLT Moments of Distributions Moment Generating Function Moment Generating Function - Properties The moment generating function of a random variable X is defined for all If X and Y are independent random variables then the moment generating real values of t by function for the distribution of X + Y is (P tx tX x e P(X = x) If X is discrete MX (t) = E[e ] = R tx t(X +Y ) tX tY tX tY x e P(X = x)dx If X is continuous MX +Y (t) = E[e ] = E[e e ] = E[e ]E[e ] = MX (t)MY (t) This is called the moment generating function because we can obtain the Similarly, the moment generating function for S , the sum of iid random raw moments of X by successively differentiating MX (t) and evaluating at n t = 0. variables X1; X2;:::; Xn is 0 0 MX (0) = E[e ] = 1 = µ0 n MS (t) = [MX (t)] d d n i M0 (t) = E[etX ] = E etX = E[XetX ] X dt dt 0 0 0 MX (0) = E[Xe ] = E[X ] = µ1 d d d M00(t) = M0 (t) = E[XetX ] = E (XetX ) = E[X 2etX ] X dt X dt dt 00 2 0 2 0 MX (0) = E[X e ] = E[X ] = µ2 Statistics 104 (Colin Rundel) Lecture 12 February 27, 2012 2 / 22 Statistics 104 (Colin Rundel) Lecture 12 February 27, 2012 3 / 22 CLT Moments of Distributions CLT Moments of Distributions Moment Generating Function - Unit Normal Moment Generating Function - Unit Normal, cont. Let Z ∼ N (0; 1) then 0 d t2=2 t2=2 Z 1 MZ (t) = e = te tZ tx 1 −x2=2 dt MZ (t) = E[e ] = e p e dx 0 0 −∞ 2π µ1 = MZ (0) = 0 Z 1 2 1 − x −tx = p e 2 dx 2 2 2π −∞ 00 d t =2 2 t =2 MZ (t) = te = (1 + t )e 1 2 dt 1 Z (x−t) t2 − 2 + 2 0 00 = p e dx µ2 = MZ (0) = 1 2π −∞ Z 1 2 t2=2 1 − (x−t) = e p e 2 dx d 2 2 M000(t) = (1 + t2)et =2 = (3t + t3)et =2 −∞ 2π Z dt t2=2 0 000 = e µ3 = MZ (0) = 0 d 2 2 M000(t) = (3t + t3)et =2 = et =2(3 + 6t2 + t4) Z dt 0 0000 µ4 = MZ (0) = 3 Statistics 104 (Colin Rundel) Lecture 12 February 27, 2012 4 / 22 Statistics 104 (Colin Rundel) Lecture 12 February 27, 2012 5 / 22 CLT Moments of Distributions CLT Moments of Distributions Sketch of Proof Central Limit Theorem Proposition Let X ; X ;::: be a sequence of independent and identically distributed 1 2 Let X1; X2;::: be a sequence of independent and identically distributed random 2 random variables each having mean µ and variance σ . Then the distribution variables and Sn = X1 +···+Xn. The distribution of Sn is given by the distribution of function fSn which has a moment generating function MSn with n ≥ 1. X + ··· + X − nµ 1 p n Let Z being a random variable with distribution function fZ and moment generat- σ n ing function MZ . tends to the unit normal as n ! 1. If MSn (t) ! MZ (t) for all t, then fSn (t) ! fZ (t) for all t at which fZ (t) is continuous. That is, for −∞ < a < 1, t2=2 We can prove the CLT by letting Z ∼ N (0; 1), MZ (t) = e and then Z a t2=2 X1 + ··· + Xn − nµ 1 −x2=2 showing for any S that M p ! e as n ! 1. P p ≤ a ! p e dx = Φ(a) as n ! 1 n Sn= n σ n 2π −∞ Statistics 104 (Colin Rundel) Lecture 12 February 27, 2012 6 / 22 Statistics 104 (Colin Rundel) Lecture 12 February 27, 2012 7 / 22 CLT Moments of Distributions CLT Moments of Distributions Proof of the CLT Proof of the CLT, cont. p Some simplifying assumptions and notation: The moment generating function of Xi = n is given by E(X ) = 0 i tX t M p (t) = E exp p i = M p Var(Xi ) = 1 Xi = n n Xi n MXi (t) exists and is finite p P n p L(t) = log M(t) and this the moment generating function of Sn= n = i = 1 Xi = n is L'Hospital's Rule: given by f (x) f 0(x) lim = lim t n x!1 g(x) x!1 g 0(x) M p (t) = M p Sn= n Xi n p Therefore in order to show MSn= n ! MZ (t) we need to show n t 2 M p ! et =2 Xi n Statistics 104 (Colin Rundel) Lecture 12 February 27, 2012 8 / 22 Statistics 104 (Colin Rundel) Lecture 12 February 27, 2012 9 / 22 CLT Moments of Distributions CLT Moments of Distributions Proof of the CLT, cont. Proof of the CLT, cont. 2 LXi (t) = log MXi (t) p n t =2 [MX (t= n)] ! e L (0) = log M (0) = log 1 = 0 i Xi Xi p 2 nLXi (t= n) ! t =2 0 d M (t) 0 Xi p p 1 L (t) = log MX (t) = 0 −3=2 Xi i L(t= n) L (t= n)(− 2 tn ) dt MXi (t) lim = lim by L'Hospital's rule n!1 −1 n!1 −2 0 n −n MX (0) µ p L0 (0) = i = = 0 L0(t= n)t Xi M (0) 1 = lim Xi n!1 2n−1=2 p L00(t= n)t(− 1 tn−3=2) = lim 2 by L'Hospital's rule 0 00 0 2 n!1 −3=2 d MX (t) MXi (t)MX (t) − [MX (t)] −n L00 (t) = i = i i Xi dt M (t) [M (t)]2 p t2 Xi Xi = lim L00(t= n) 00 0 2 n!1 2 MXi (0)MX (0) − [MX (0)] L00 (0) = i i 2 Xi 2 t [MX (0)] = i 2 0 2 2 E(X )E(X ) − E(Xi ) = i i = E(X 2) − E(X )2 = σ2 = 1 0 2 i i E(Xi ) Statistics 104 (Colin Rundel) Lecture 12 February 27, 2012 10 / 22 Statistics 104 (Colin Rundel) Lecture 12 February 27, 2012 11 / 22 CLT Moments of Distributions CLT Continuous Random Variables Proof of the CLT, Final Comments Cumulative Distribution Function The preceding proof assumes that E(Xi ) = 0 and Var(Xi ) = 1. We have already seen a variety of problems where we find P(X <= x) or P(X > x) etc. The former is given a special name - the cumulative We can generalize this result to any collection of random variables Yi by distribution function. considering the standardized form Y ∗ = (Y − µ)/σ. i i If X is discrete with probability mass function f (x) then x X Y + ··· + Y − nµ Y − µ Y − µ p P(X ≤ x) = F (x) = f (z) 1 p n = 1 + ··· + n n σ n σ σ z=−∞ ∗ ∗ p = (Y1 + ··· + Yn ) = n If X is continuous with probability density function f (x) then Z x P(X ≤ x) = F (x) = f (z)dz ∗ E(Yi ) = 0 −∞ ∗ Var(Yi ) = 1 CDF is defined for for all −∞ < x < 1 and follows the following rules: lim F (x) = 0 lim F (x) = 1 x < y ) F (x) < x→−∞ x!1 F (y) Statistics 104 (Colin Rundel) Lecture 12 February 27, 2012 12 / 22 Statistics 104 (Colin Rundel) Lecture 12 February 27, 2012 13 / 22 CLT Continuous Random Variables CLT Continuous Random Variables Binomial CDF Uniform CDF Let X ∼ Binom(n; p) then Let X ∼ Unif(a; b) then Probability Mass Function Cumulative Density Function Probability Mass Function Cumulative Density Function ( 8 1 0 for x ≤ a ! b−a for x 2 [a; b] > n bxc ! f (x) = < k n−k X n k n−k F (x) = x−a P(X = k) = f (k) = p (1 − p) P(X ≤ x) = F (x) = p (1 − p) 0 otherwise b−a for x 2 [a; b] k k > k=0 :1 for x ≥ b Statistics 104 (Colin Rundel) Lecture 12 February 27, 2012 14 / 22 Statistics 104 (Colin Rundel) Lecture 12 February 27, 2012 15 / 22 CLT Continuous Random Variables CLT Continuous Random Variables Normal CDF Exponential Distribution Let X ∼ N (µ, σ2) then In general terms, the Exponential distribution describes the time between events which occur continuously with a given rate λ (the expected number Probability Mass Function Cumulative Density Function of events in a given unit of time). 2 1 − (x−µ) f (x) = φ(x) = p e 2σ2 F (x) = Φ(x) Let X ∼ Exp(λ), we define one unit of time as 1/λ which we can 2πσ sub-divide into n sub-intervals. The probability that an event occurs during a particular sub-interval is approximately λ/n. The probability that we must wait b or fewer units of time between events is the same as the probability that an event does occur in one of the b · nth sub-intervals. Therefore, if we let Y ∼ Geo(λ/n) then bn−1 bn−1 k X X λ λ P(X ≤ b) ≈ P(Y ≤ nb) = P(Y = k) = 1 − n n k=0 k=0 Statistics 104 (Colin Rundel) Lecture 12 February 27, 2012 16 / 22 Statistics 104 (Colin Rundel) Lecture 12 February 27, 2012 17 / 22 CLT Continuous Random Variables CLT Continuous Random Variables Exponential Distribution, cont.
Details
-
File Typepdf
-
Upload Time-
-
Content LanguagesEnglish
-
Upload UserAnonymous/Not logged-in
-
File Pages6 Page
-
File Size-