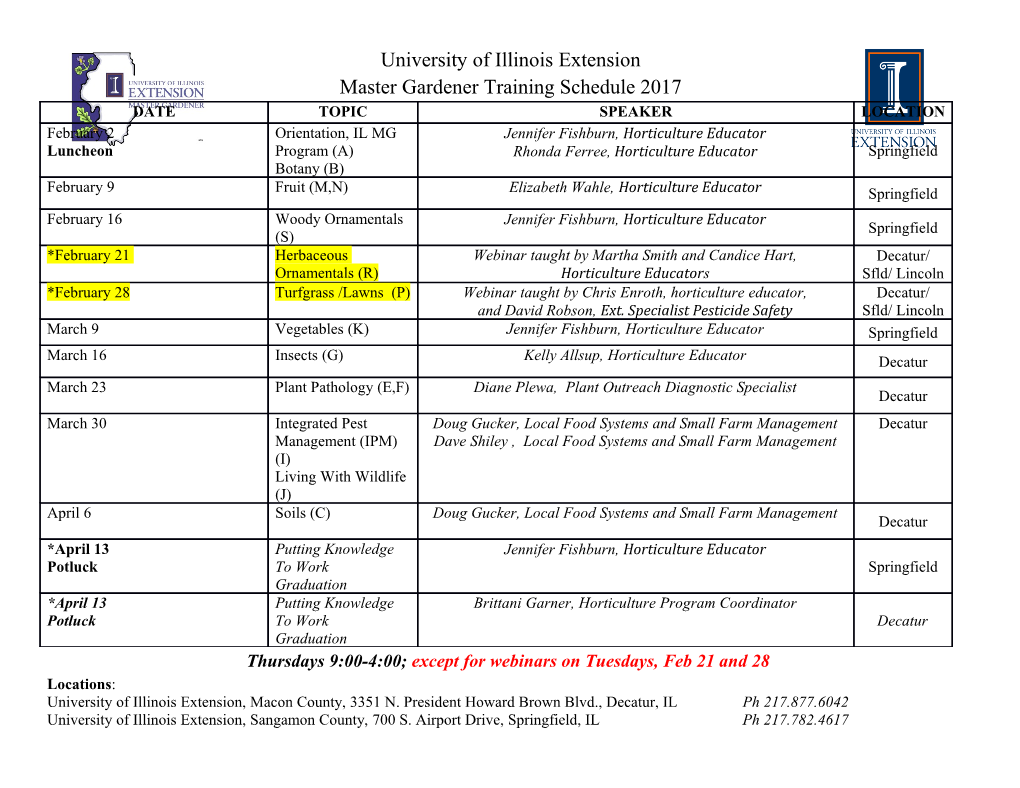
H OH metabolites OH Article Circulating Metabolites Associated with Body Fat and Lean Mass in Adults with Overweight/Obesity Christopher Papandreou 1,2,3,† , Jesús García-Gavilán 1,2,3,† , Lucía Camacho-Barcia 3,4 , Thea T. Hansen 5 , Anders Sjödin 5, Joanne A. Harrold 6, Jason C. G. Halford 6,7 and Mònica Bulló 1,2,3,* 1 Department of Biochemistry and Biotechnology, Faculty of Medicine and Health Sciences, University Rovira i Virgili (URV), 43201 Reus, Spain; [email protected] (C.P.); [email protected] (J.G.-G.) 2 Institute of Health Pere Virgili—IISPV, University Hospital Sant Joan, 43201 Reus, Spain 3 Consorcio CIBER, M.P. Fisiopatología de la Obesidad y Nutrición (CIBEROBN), Instituto de Salud Carlos III, 28029 Madrid, Spain; [email protected] 4 Department of Psychiatry, University Hospital of Bellvitge-IDIBELL, Hospitalet de Llobregat, 08908 Barcelona, Spain 5 Section for Obesity Research, Department of Nutrition, Exercise and Sports, University of Copenhagen, 2200 Copenhagen, Denmark; [email protected] (T.T.H.); [email protected] (A.S.) 6 Department of Psychology, Institute of Population Health, University of Liverpool, Liverpool L69 3GL, UK; [email protected] (J.A.H.); [email protected] (J.C.G.H.) 7 Appetite Control & Energy Balance Research Group, School of Psychology, Faculty of Medicine & Health, University of Leeds, Leeds LS2 9JT, UK * Correspondence: [email protected] † These authors contributed equally to this work. Citation: Papandreou, C.; Abstract: The interplay between fat mass and lean mass within human metabolism is not completely García-Gavilán, J.; Camacho-Barcia, understood. We aimed to identify specific circulating metabolomic profiles associated with these L.; Hansen, T.T.; Sjödin, A.; Harrold, body composition compartments. Cross-sectional analyses were conducted over 236 adults with J.A.; Halford, J.C.G.; Bulló, M. overweight/obesity from the Satiety Innovation (SATIN) study. Body composition was assessed Circulating Metabolites Associated by dual-energy X-ray absorptiometry. A targeted multiplatform metabolite profiling approach was with Body Fat and Lean Mass in applied. Associations between 168 circulating metabolites and the body composition measures were Adults with Overweight/Obesity. assessed using elastic net regression analyses. The accuracy of the multimetabolite weighted models Metabolites 2021, 11, 317. https:// was evaluated using a 10-fold cross-validation approach and the Pearson’s correlation coefficients doi.org/10.3390/metabo11050317 between metabolomic profiles and body compartments were estimated. Two different profiles Academic Editor: Thusitha including 86 and 65 metabolites were selected for % body fat and lean mass. These metabolites mainly W. Rupasinghe consisted of lipids (sphingomyelins, phosphatidylcholines, lysophosphatidylcholines), acylcarnitines, and amino acids. Several metabolites overlapped between these body composition measures but none Received: 17 April 2021 of them towards the same direction. The Pearson correlation coefficients between the metabolomic Accepted: 12 May 2021 profiles and % body fat or lean mass were 0.80 and 0.79, respectively. Our findings suggest alterations Published: 13 May 2021 in lipid metabolism, fatty acid oxidation, and protein degradation with increased adiposity and decreased lean body mass. These findings could help us to better understand the interplay between Publisher’s Note: MDPI stays neutral body composition compartments with human metabolic processes. with regard to jurisdictional claims in published maps and institutional affil- Keywords: metabolomics; body composition; fat mass; lean mass; SATIN iations. 1. Introduction Copyright: © 2021 by the authors. Increased prevalence of obesity, assessed by body mass index (BMI), is one of the Licensee MDPI, Basel, Switzerland. largest health concerns globally being a major risk factor for a number of prevalent chronic This article is an open access article diseases [1]. However, BMI is an indirect estimate of adiposity as it does not distinguish distributed under the terms and between fat mass and lean mass. An abundance of evidence indicates an increased risk of conditions of the Creative Commons Attribution (CC BY) license (https:// cardiometabolic diseases [2,3] and mortality [4] for those who have an increased body fat creativecommons.org/licenses/by/ and/or reduced lean mass. However, the underlying mechanisms linking these two com- 4.0/). partments of body composition with health outcomes are not fully understood. It has been Metabolites 2021, 11, 317. https://doi.org/10.3390/metabo11050317 https://www.mdpi.com/journal/metabolites Metabolites 2021, 11, 317 2 of 11 suggested that several factors including genetic, physiologic, metabolic, and behavioral may explain this link [5]. Prior studies have identified different circulating metabolites such as amino acids, acylcarnitines, or lipid species associated with body fat [6–8], lean mass [7,9–11], and metabolic risk [6,12,13]. However, to date, limited metabolomic-analysis has been conducted using combinations of different metabolomic platforms to cover a wide range of metabolites and examine their association with these body composition com- partments. A comprehensive metabolite profiling (metabolomics) may provide a deeper understanding of the interplay between fat mass and lean mass with human metabolism. The exclusion of participants with manifestation ofeither cardiometabolic diseases also reduces unwanted confounding when investigating body composition measures in relation to metabolomic profiles [14]. Therefore, we used a multiplatform metabolomics approach to identify circulating metabolomics profiles associated with body fat and lean mass in participants with over- weight/obesity included in the EU project Satiety Innovation (SATIN) study. 2. Results The general characteristics of the 236 participants are summarized in Table1. The mean age was 46.4 years, with a mean body mass index (BMI) of 31.1kg/m2. The majority of women were pre-menopausal (>90%). The mean % body fat was 42.0, and the lean mass was 47.2 kg. Pearson’s correlation analysis revealed that % body fat was significantly correlated with lean mass (r = −0.71, p-value: <0.001). Table 1. Characteristics of study participants. Characteristics (n = 236) Age, years 46.4 ± 10.7 Women sex, N (%) 184 (78) Weight, kg 87.5 ± 11.2 BMI, kg/m2 31.1 ± 2.2 Body fat, % 42.0 ± 5.6 Lean mass, kg 47.2 ± 9.2 Glucose, mg/dL 93.3 ± 11.0 Total cholesterol, mg/dL 196.0 ± 34.9 HDL-C, mg/dL 55.7 ± 15.3 LDL-C, mg/dL 119.9 ± 30.5 Triglycerides, mg/dL 102.3 ± 48.9 Data shows mean ± SD or number (%); Abbreviations: BMI, body mass index; HDL-C, high-density lipoprotein cholesterol; LDL-C, low-density lipoprotein. 2.1. Circulating Metabolites Associated with Body Composition Measures Of the 168 metabolites used in the analyses, the elastic net regression model selected 86 and 65 metabolites for % body fat and lean mass, respectively (Figures1 and2). The selected metabolites shown in the respective Figures1 and2 were ranked from the highest to the lowest elastic net positive and negative regression coefficients. Mean and SD of the set of 86 metabolites selected 9–10 times in the 10-fold CV elastic lin- ear regression procedure (using lambda.min). Metabolites with negative coefficients (m = 43) are plotted in the left part, whereas those with positive coefficients (m = 43) are shown in the right part. Abbreviations: ARA + EPA, Arachidonic acid + Eicosapentaenoic acid; LPC, Lysophosphatidylcholine; PC, Phosphatidylcholine; PE, Phosphatidylethanolamine; SM, Sphingomyelin; TG, Triacylglycerides; TMAO, Trimethylamine N-oxide. Mean and SD of the set of 65 metabolites selected 9–10 times in the 10-fold CV elastic lin- ear regression procedure (using lambda.min). Metabolites with negative coefficients (m = 32) are plotted in the left part, whereas those with positive coefficients (m = 33) are shown in the right part. Abbreviations: ARA + EPA, Arachidonic acid + Eicosapentaenoic acid; LPC, Lysophosphatidylcholine; PC, Phosphatidylcholine; PE, Phosphatidylethanolamine; PUFA, polyunsaturated fatty acids; SM, Sphingomyelin; TG, Triacylglycerides. Metabolites 2021, 11, 317 3 of 11 Figure 1. Coefficients (mean ± SD) for the metabolites selected 9–10 times in the 10-fold CV linear elastic regression and associated with % body fat. Figure 2. Coefficients (mean ± SD) for the metabolites selected 9–10 times in the 10-fold CV linear elastic regression and associated with lean mass. 2.2. Metabolomic Profile of Body Fat Forty-three metabolites were positively associated with % body fat, and 43 were neg- atively associated. High positive regression coefficients were found for sphingomyelins (SMs: C32:2, C34:2, C38:2, C34:0), linoleic acid, serine, threonine, alanine, six carnitines (methylglutaryl-, tiglyl-, hexanoyl-, pimelyl-, decenoyl-, hexadecenyl-), phosphatidyl- Metabolites 2021, 11, 317 4 of 11 choline (PC: C38:3), total lysophosphatidylcholine (LPC), TG C54:2, sucrose, glycolic acid, followed by other carnitines, several PCs, SMs, and phosphatidylethanolamines (PEs), oleic acid, palmitic acid, glycerol and phenylalanine.The highest negative regression coefficient was found for tryptophan followed by SM C42:1, octadecanoyl-carnitine, TG C50:3, LPC C18:2, SM C35:1, 3-hydroxybutanoic acid, methionine, PCs (C38:6, C40:4), eight other carnitines (decadienoyl-, dodecanoyl-, C16 OH, free, glutaryl-, tridecanoyl-, octenoyl-, methyl-malonyl-), LPC C16:0, SM C34:1, TMAO, leucine, other carnitines, several
Details
-
File Typepdf
-
Upload Time-
-
Content LanguagesEnglish
-
Upload UserAnonymous/Not logged-in
-
File Pages11 Page
-
File Size-