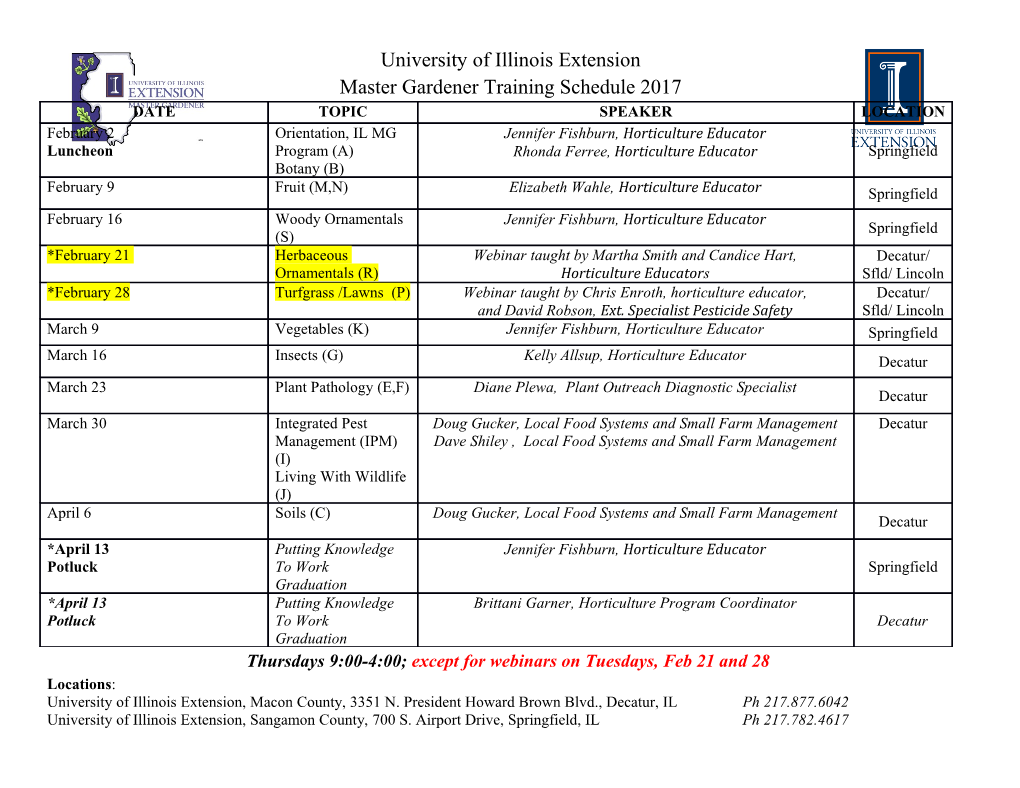
Vol. 7, No. 2, February 2011 “Can You Handle the Truth?” Making Sense of Biostatistics: Simple, Blocked and Stratified Randomization By William Irish A randomized clinical study tests the safety and efficacy of a healthcare intervention by randomly recruiting participants into either the treatment or the control group. Participants are placed into a study arm (e.g., active treatment) based solely on chance and not on the preference or influence of the physician, subject or study sponsor. Random assignment allows one to assume that all treatment arms contain participants with roughly the same characteristics (e.g., age and gender) and that measured outcomes are due to the intervention and not to differences in participant characteristics. For instance, if a study is not randomized, physicians might unconsciously assign participants with a more hopeful prognosis to the experimental group, thus making the new therapy seem more effective. Conversely, participants with a less hopeful prognosis might pick the experimental treatment, leading it to look less effective. The easiest and most popular form of randomization is simple randomization. With simple randomization, a participant is assigned to treatment without any regard to previous treatment assignments. For example, when a subject becomes eligible for randomization, a coin is tossed. If the coin turns up heads, the subject is assigned to Group A; if tails, to Group B. This process continues until all participants are randomized. Using this procedure, approximately one half of the subjects are assigned to Group A and one half to Group B. The randomization process is normally automated by creating a randomization schedule using a random number generator, available in most statistical software. Simple randomization is easy to implement, but it does have several disadvantages. First, it probably will not yield an exact 50:50 ratio. At any point in the randomization, including the end, there could be a substantial imbalance, particularly if the sample size is small. For example, if 20 subjects are randomized with equal probability to two treatment groups (Group A or Group B), the chance of a 12:8 split or worse is 50%. However, with 100 subjects, the chance of the same ratio (60:40 split) or worse is only 5%. Such imbalances do not invalidate the statistical tests, but they do reduce their accuracy. In addition, such imbalances can appear awkward and lead to loss of credibility for the trial. To avoid an imbalance in the number of subjects assigned to each treatment group, blocked randomization (also called permuted-block randomization) is often used. This approach guarantees that the imbalance be never be large and that, at certain points, the number of subjects in each group will be equal. Blocked randomization works in this way: Suppose subjects are to be randomly assigned to two treatment groups (Group A or Group B) and that each treatment has equal probability (i.e., 50:50). First, we need to decide on the block size. With two treatment groups, we could choose an even block size of, for example, four. Within each block, one half of the subjects will be assigned to Group A and the other half to Group B. The order in which the interventions are assigned in each block is randomized (e.g., ABBA). This process is repeated for consecutive blocks of subjects until all subjects are randomized (e.g., ABBA, AABB, BAAB, ABBA, and so on). Although blocked randomization is more likely to yield a 50:50 assignment of subjects, in some trials it can allow the investigator to guess which assignment is coming up next and enroll subjects accordingly. It also does not work well in multicenter trials that enroll a small number of subjects per research site, unless the assignment order is also randomized across research sites. Subscribe free at www.firstclinical.com © 2011 First Clinical Research and the Author(s) To maintain balanced assignments across prognostic factors, stratified randomization divides the subject population into two or more subgroups (“strata”), such as gender (male vs. female) or age (e.g., 18 to 39, 40 to 59, 60+ years). With two factors, the number of strata multiplies, e.g., males age 18 to 39, females age 18 to 39, etc.) Within the stratum, simple randomization or permuted block randomization is used, the latter being the most common method. Deciding which type of randomization to use may not be a simple decision. It depends on several factors: single center vs. multicenter, total sample size, number of potential strata, and stratum prevalence in the study population. To illustrate this, suppose there are three important prognostic factors on which to stratify the randomization: age with three subgroups (“levels”), sex with two levels, and co-morbidity with three levels. In this example, we would have a total of 3X2X3 = 18 strata. As factors are added and the levels within a factor refined (e.g., four age groups), the number of strata can increase rapidly. If there are 108 patients to be randomized with 18 strata, then only six patients would be expected per stratum, based on the unlikely assumption that the study population is evenly distributed across the levels. It is possible to manage enrollments to equalize the numbers, but at the cost of refusing enrollment to otherwise qualified subjects, an extra large challenge in multicenter studies. With increased stratification, the randomization procedure is more apt to be self-defeating (i.e., lose statistical efficiency), especially in small studies, due to sparseness of data within each stratum. Therefore, the number of strata should be kept to a minimum. Generally, in studies of 100 subjects or less, stratified randomization with no more than two or three prognostic factors should be used. In larger studies, simple permuted block randomization can be used, with important prognostic factors handled in the statistical analysis. Simple, blocked and stratified randomization are not the only approaches. Over the past few years, adaptive randomization has become more popular. In these randomization schemes, the probability of assignment to a treatment arm varies based on previous assignments, thereby addressing imbalances over time. A detailed discussion of these methods can be found in Kalish and Begg.1 Reference 1. Kalish LA and Begg CB. Treatment allocation methods in clinical trials: A review. Stat in Med 4:129-144, 1985. Author William Irish, PhD, is Vice President of Outcomes Research and Biostatistics at CTI Clinical Trial and Consulting Services. Contact him at 513.618.4057 or [email protected]. Subscribe free at www.firstclinical.com 2 © 2011 First Clinical Research and the Author(s) .
Details
-
File Typepdf
-
Upload Time-
-
Content LanguagesEnglish
-
Upload UserAnonymous/Not logged-in
-
File Pages2 Page
-
File Size-