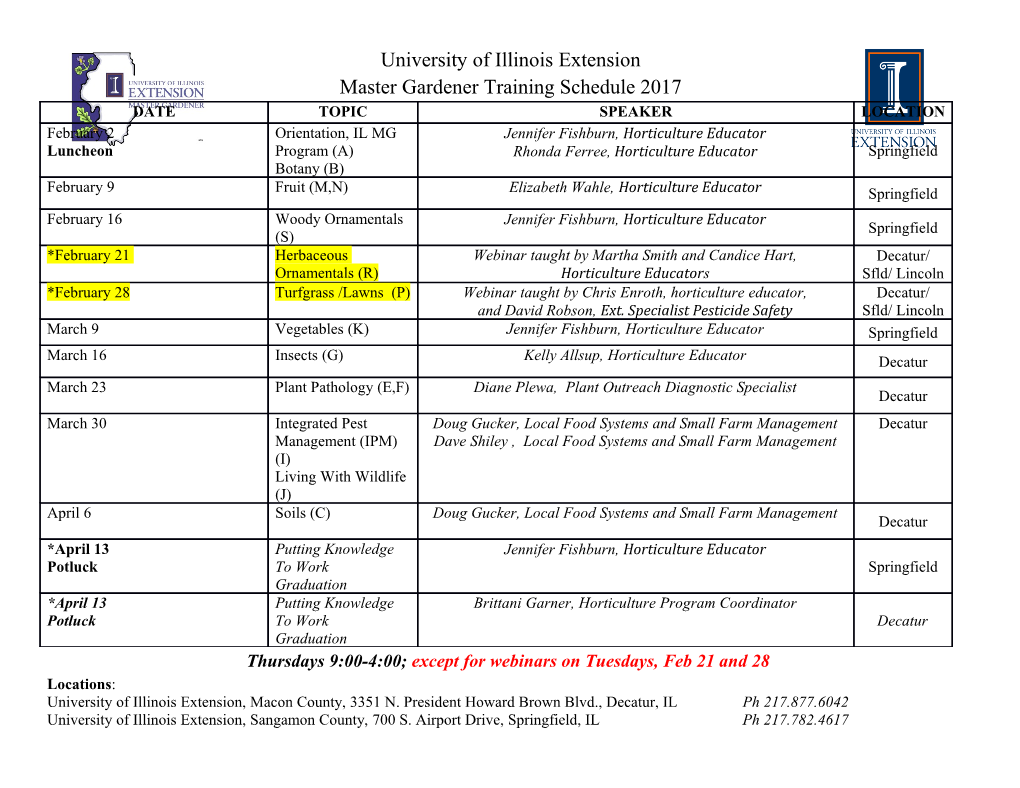
Lecture 2: ARMA(p,q) models (part 2) Florian Pelgrin University of Lausanne, Ecole´ des HEC Department of mathematics (IMEA-Nice) Sept. 2011 - Jan. 2012 Florian Pelgrin (HEC) Univariate time series Sept. 2011 - Jan. 2012 1 / 40 Introduction Motivation Characterize the main properties of MA(q) models. Estimation of MA(q) models Florian Pelgrin (HEC) Univariate time series Sept. 2011 - Jan. 2012 2 / 40 Introduction Road map 1 Introduction 2 MA(1) model 3 Application of a "counterfactual" MA(1) 4 Moving average model of order q, MA(q) 5 Application of a MA(q) model Florian Pelgrin (HEC) Univariate time series Sept. 2011 - Jan. 2012 3 / 40 MA(1) model Moving average models 2.1. Moving average model of order 1, MA(1) Definition A stochastic process (Xt )t2Z is said to be a moving average model of order 1 if it satisfies the following equation : Xt = µ + t − θt−1 8t where θ 6= 0, µ is a constant term, (t )t2Z is a weak white noise process 2 2 with expectation zero and variance σ (t ∼ WN(0; σ )). Florian Pelgrin (HEC) Univariate time series Sept. 2011 - Jan. 2012 4 / 40 MA(1) model Remarks : 1. In lag notation, one has : Xt = µ + Θ(L)t ≡ µ + (1 − θL)t 2. The previous process can be written in mean-deviation as follows : X~t = t − θt−1 where X~t = Xt − µ. Florian Pelgrin (HEC) Univariate time series Sept. 2011 - Jan. 2012 5 / 40 MA(1) model Remarks (cont'd) : 3. The properties of (Xt ) only depend on those of the weak white noise process (t ). To some extent, the behavior of (Xt ) is more noisy relative to an AR(1) process... 4. Iterating on the past infinite (and with some regularity conditions), the infinite autoregressive representation writes : 1 µ X X = + θk X + : t 1 − θ t−k t k=1 5. The infinite autoregressive representation illustrates the fact that a certain form of persistence is captured by a moving average model, especially when θ is close to one. Florian Pelgrin (HEC) Univariate time series Sept. 2011 - Jan. 2012 6 / 40 MA(1) model Simulation of a moving average process of order 1 (θ = 0.9) 4 3 2 1 0 -1 -2 -3 -4 -5 50 100 150 200 250 300 350 400 450 500 Florian Pelgrin (HEC) Univariate time series Sept. 2011 - Jan. 2012 7 / 40 MA(1) model Scatter plots of a moving average process of order 1: Left panel (X t-1 versus X t) and right panel (X t-2 versus X t) 4 4 3 3 2 2 1 1 0 0 -1 -1 X _(t-2) X _(t-1) -2 -2 -3 -3 -4 -4 -5 -5 -5 -4 -3 -2 -1 0 1 2 3 4 -5 -4 -3 -2 -1 0 1 2 3 4 X_t X_t Florian Pelgrin (HEC) Univariate time series Sept. 2011 - Jan. 2012 8 / 40 Figure: Scatter plots MA(1) model Stationarity and invertibility conditions Since (t ) is a weak noise process, (Xt ) is weakly stationary (by definition). The invertibility condition is the counterpart of the stability (stationary) condition of an AR(1) process : 1 If jθj < 1, then (Xt ) is invertible. 2 If jθj = 1, then (Xt ) is non invertible. 3 If jθj > 1, there exists a non-causal invertible representation of (Xt ) that we rule out. Florian Pelgrin (HEC) Univariate time series Sept. 2011 - Jan. 2012 9 / 40 MA(1) model Alternatively, if jθj < 1, then : 1 X (1 − θL)−1 = θk Lk k=0 and −1 t = (1 − θL) (Xt − µ) i.e. 1 µ X X = + θk X + t 1 − θ t−k t k=1 1 This is the infinite autoregressive representation of a MA(1) process. 2 The MA(1) representation is then called the fundamental or causal representation. Florian Pelgrin (HEC) Univariate time series Sept. 2011 - Jan. 2012 10 / 40 MA(1) model More formally... Definition The representation of the moving average process of order one defined by : Xt = µ + t − θt−1; is said to be causal or fundamental|(t ) is the innovation process|if the root of the characteristic equation zΘ(z−1) = 0 ≡ z − θ = 0 lies outside the unit circle : jzj < 1 , jθj < 1: Florian Pelgrin (HEC) Univariate time series Sept. 2011 - Jan. 2012 11 / 40 MA(1) model Remark : One can also use the inverse characteristic equation to find the invertibility condition : Θ(z) = 0 , 1 − θz = 0: The condition writes (for a MA(1) process) : jz∗j > 1 , jθj < 1: Florian Pelgrin (HEC) Univariate time series Sept. 2011 - Jan. 2012 12 / 40 MA(1) model Moments of a MA(1) Definition Let (Xt ) be a stationary stochastic process that satisfies a (fundamental) MA(1) representation, Xt = µ + t − θt−1. Then : E [Xt ] = µ 2 2 V [Xt ] = (1 + θ )σ 2 γX (1) = −θσ γX (h) = 0 for all jhj > 1 Florian Pelgrin (HEC) Univariate time series Sept. 2011 - Jan. 2012 13 / 40 MA(1) model Definition Let (Xt ) be a stationary stochastic process that satisfies a (fundamental) MA(1) representation, Xt = µ + t − θt−1. Then, the autocorrelation function is given by : 8 1 if h = 0 < θ ρX (h) = − 1+θ2 if h = ±1 : 0 otherwise. Florian Pelgrin (HEC) Univariate time series Sept. 2011 - Jan. 2012 14 / 40 MA(1) model The autocorrelation function of a moving average process of order 1, MA(1), is always zero for orders higher than 1 (jhj > 1) : MA(1) process has no memory beyond 1 period (see Scatter plots and autocorrelograms). This property generalizes to MA(p) processes. Partial autocorrelations : Nothing special, with the exception that it should decrease (possibly, with damped oscillations) ! The partial autocorrelation function cannot help for characterizing a MA(1). Florian Pelgrin (HEC) Univariate time series Sept. 2011 - Jan. 2012 15 / 40 MA(1) model Correlograms of a moving average process of order one ( θ = 0.9, 0.5, and 0.2) Florian Pelgrin (HEC) Univariate time series Sept. 2011 - Jan. 2012 16 / 40 Figure: (Partial) Autocorrelation function MA(1) model Correlogram of an AR(1) with phi=0.8 Correlogram of an AR(1) with phi=-0.8 0.8 1 0.6 0.5 0.4 0 0.2 -0.5 0 -0.2 -1 0 5 10 15 20 0 5 10 15 20 Correlogram of an MA(1) with theta=0.8 Correlogram of an MA(2) with theta=(0.4;0.3) 0.2 0.2 0.1 0 0 -0.2 -0.1 -0.4 -0.2 -0.6 -0.3 0 5 10 15 20 0 5 10 15 20 Florian Pelgrin (HEC) Univariate time series Sept. 2011 - Jan. 2012 17 / 40 Figure: AR(1) versus MA(1) and MA(2) MA(1) model Estimation Estimation is "more difficult” since the t terms are not observed ! Different techniques : 1 Conditional nonlinear least squares estimator 2 Maximum likelihood estimator 3 Generalized method of moments estimator. Without loss of generality, the constant term is omitted and the model is written as : Xt = t + θt−1: Florian Pelgrin (HEC) Univariate time series Sept. 2011 - Jan. 2012 18 / 40 MA(1) model Nonlinear conditional least squares estimator The "objective function" of the ordinary least squares estimator is : T X 2 θ^mco = argmin (xt − θt−1) θ t=2 Conditionally on 0, one has (backcasting procedure) : t−2 X j t−1 t−1 = (−θ) xt−1−j + (−θ) 0 j=0 Suppose that 0 = 0, the nonlinear objective function (with respect to θ) writes : 2 T 0 t−2 1 X X j @xt − θ (−θ) xt−1−j A t=2 j=0 Florian Pelgrin (HEC) Univariate time series Sept. 2011 - Jan. 2012 19 / 40 MA(1) model The conditional nonlinear least squares estimator of θ is defined by : 2 T 0 t−2 1 ^ X X j θcnls = argmin @xt − θ (−θ) xt−1−j A θ t=2 j=0 The asymptotic distribution is given by : p a:d: 2 T θ^cnls − θ !N (0; 1 − θ ) The effect of 0 = 0 dies out if T is sufficiently large. An alternative is to consider 0 as an unknown parameter. 2 An estimator of σ is : T 1 X σ^2 = (x − θ^ )2: T − 1 t cnls t−1 t=2 Florian Pelgrin (HEC) Univariate time series Sept. 2011 - Jan. 2012 20 / 40 MA(1) model Maximum likelihood estimator Two estimators : the conditional maximum likelihood estimator and the exact maximum likelihood estimator The conditional maximum likelihood estimator proceeds in the same way as the conditional nonlinear least squares estimator (backcasting procedure) : Suppose that t is a Gaussian White noise process For t = 1 : 1 = x1 − θ0 For t > 1 : t−1 X j t t = (−θ) xt−j + (−θ) 0 j=0 Write the conditional likelihood function (with 0 = 0) and maximize 2 with respect to θ and σ . Florian Pelgrin (HEC) Univariate time series Sept. 2011 - Jan. 2012 21 / 40 MA(1) model The exact maximum likelihood estimator can be calculated by two convenient algorithms : 1 The Kalman filter 2 The triangular factorization of the variance-covariance matrix of a MA(1) process In contrast to the conditional maximum likelihood estimator, the exact maximum likelihood estimator does not require that the moving average representation is invertible. Florian Pelgrin (HEC) Univariate time series Sept. 2011 - Jan. 2012 22 / 40 MA(1) model The (Generalized) method of moments estimator A simple method of moments estimator... Consider the first two moments of a MA(1) : 2 2 2 2 E Xt = (1 + θ )σ and E [Xt Xt−1] = θσ Using the empirical counterpart of these two moments conditions yields : T 2 2 2 2 −1 X xt − σ (1 + θ ) gT (x; θ; σ ) = T 2 xt xt−1 − σ θ t=1 −1 PT 2 2 2 ! T t=1 xt − σ (1 + θ ) = −1 PT 2 T t=2 xt xt−1 − σ θ ^ 2 Solving the exactly (just-) identified equation gT (x; θ; σ^ ) = 02×1 for ^ 2 θ andσ ^ (with sone regularity conditions...) gives the method of moment estimator.
Details
-
File Typepdf
-
Upload Time-
-
Content LanguagesEnglish
-
Upload UserAnonymous/Not logged-in
-
File Pages40 Page
-
File Size-