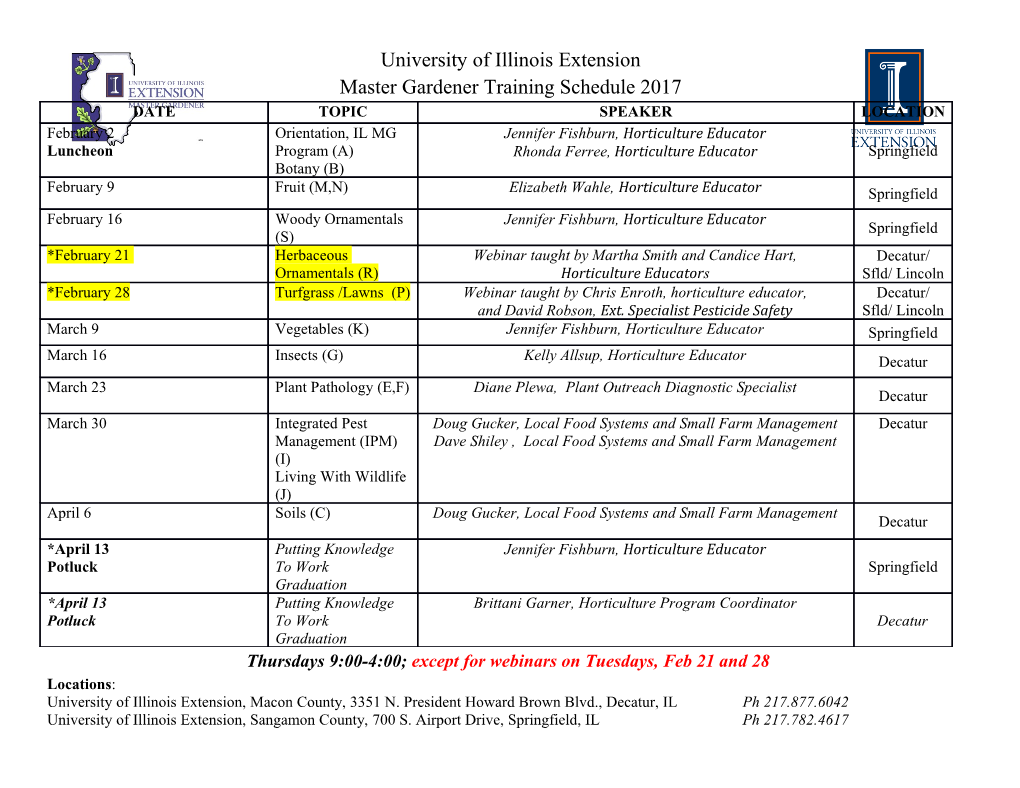
4/23/2020 Pathways to perception CISC 3250 in 3 (or fewer) synaptic steps 0 Input through sensory organ/tissue Types of percepts Systems Neuroscience in this lecture: 1 Synapse onto neurons in - Tactile (touch) Perception spinal cord/brain stem - Audition (sound) - Vision (sight) 2 Synapse onto neurons in thalamus 3 Synapse onto cortical neurons in Professor Daniel Leeds “primary ____ cortex” [email protected] 4+ Further cortical processing JMH 332 2 Touch/“Tactile” Touch: Inputs Mechanoreceptors in skin Skin Spinal • Pacinian corpuscles – vibrations cord • Meissner’s corpuscles – light touch Dorsal • Merkel’s discs – pressure and texture B horn Thalamus A C • Ruffini endings – stretch D Ventral F E postero- lateral (VPL) Cortex nucleus Post-central gyrus (SI) 3 4 1 4/23/2020 Thalamus – the “relay” station Hearing/“Auditory” Region names Cochlea Geniculate nuclei at largely based on most posterior location ventral spots in Cochlear Cochlear nucleus (-> thalamus VPL for nerve Superior olive) -> somatosensation Inferior colliculus Thalamus Brain stem Medial Recall: in cochlea geniculate VPL = have tonotopy nucleus Ventral (bottom) Cortex Neurons selective for (MGN) Posterior (back) Primary specific frequencies Lateral (side) Nucleus auditory 6 cortex (AI)7 http://en.wikipedia.org/wiki/File:Thalamus-schematic.svg Hearing and frequency decomposition Common patterns in speech i u ɑ • Vowels (a,e,i,o,u) Hz 5000 Sound consists of times and correspond to steady frequencies frequency combinations 3000 time 1000 Freq Time-bound wavelets: Top 2 freqs: i 300, 2500; u 300, 1000; ɑ 500, 1000 Similar to cochlear neurons • Consonants may be broad- 2 푡2 range frequencies, or 2 푡 − 1 − 푒 2휎2 time 1/4 sweeps 3휎휋 휎 Spectrogram 8 “Mexican hat” 9 S p a 2 4/23/2020 More speech pattern Spectro-temporal receptive fields Nagel 2008 Neuron Zebra Finch (field L) • Speech formant ranges by frequency AI (primary auditory cortex) neurons selective for patterns in space and time • ch, s – long high freq • d, k, t – broad freq burst • l, r, n, m – freq slide 10 11 Binaural hearing Math of sound localization Comparing sounds from left and right Speed of sound • Time shift and/or Volume Change c=343 m/s Human head 훼 Left Right listener b=0.2m speaker If Δ푡 =5ms 푐Δ푡 훼 = sin−1 횫풅=343x0.005 = 1.7m 푏 1.7 m Left Right 풐풑풑풐풔풊풕풆 Applications: 퐬퐢퐧 휶 = 0.2 m 풉풚풑풐풕풆풏풖풔풆 Jeffres ’48 횫풅 • Localize sound source = sound delay/ 풃 • Distinguish sounds from multiple sources 12 axon delay model 13 3 4/23/2020 Math of sound localization Math of sound localization Speed of sound 훼=58o Speed of sound c=343 m/s c=343 m/s 0.17 m Human head L 0.2 m R Human head b=0.2m b=0.2m If Δ푡 =0.5ms 푐Δ푡 푐Δ푡 훼 = sin−1 훼 = sin−1 횫풅=343x0.0005 = 0.17m 푏 푏 0.17 m ퟎ. ퟏퟕ 퐬퐢퐧 휶 = 휋 ퟎ. ퟐ 90o = 1.57 rad ( rad) 0.2 m = ퟎ. ퟖퟓ 2 Pick direction for comparison Sound gets R ear @ 휶 = 퐬퐢퐧−ퟏퟎ. ퟖퟓ x radians: > 0 rightSound earlier 1345.2 ms 휶 = ퟓퟖ퐨 90푥 360푥 Δ푡 = ≈ degrees14 < 0 leftSound earlier 15 to L ear @ 1345.7 ms 1.57 2휋 Math of sound localization Seeing/“Visual” Speed of sound x radians: c=343 m/s 90푥 360푥 ≈ degrees 1.57 2휋 Human head Retina b=0.2m 푐Δ푡 Thalamus 훼 = sin−1 푏 Optic Lateral nerve Sound gets R ear @ Which ear is sound closest to? geniculate nucleus 258.5 ms 285.5ms The ear that sound arrives at first (LGN) Get to L ear @ Ear with smaller time of arrival Cortex 285.3 ms ∆풅 = 풄∆풕 = ퟑퟒퟑ × (ퟎ. ퟐퟖퟓퟓ − ퟎ. ퟐퟖퟓퟑ) Primary ∆풅 ퟑퟒퟑ × ퟎ. ퟎퟎퟎퟐ . ퟎퟔퟖퟔ visual cortex 퐬퐢퐧 휶 = = = = ퟎ. ퟑퟓ (VI) What’s my 훼? 풃 ퟎ. ퟐ ퟎ. ퟐ Closer to L ear 휶 = 풂풔풊풏 ퟎ. ퟑퟓ = ퟐퟎo 16 19 4 4/23/2020 Sensitivity to perceptual variations Selectivity to perceptual variations • V1: Surround-suppression for shifted edges • PFC: Same object detected at diverse locations and scales • More complex percepts invariant to greater spatial transformations 21 22 HMAX – model of hierarchical vision HMAX – model of hierarchical vision 1. Gabor “filters” (edge detectors) V4 V2 V1 IT 2. Perform “Max pooling” (semi-invariance over space) 3. Weighted combination of space-invariant edges 4. Further max pooling • Higher cortical levels cover larger visual spans • Object recognition invariant to changes in location and orientation 23 24 5 4/23/2020 Higher HMAX layers cover more space Functions of HMAX layers • Odd layers (layer 1, 3, 5, …) look for specific combinations of lower-level features • Even layers (layer 2, 4, 6, …) provide invariance to some feature changes (e.g., shift in position) Example coverage layer 1 layer 2 layer 1 layer 2 layer 3 layer 4 + + for layer x neurons layer 3 25 Fire for 1 lines Fire for 1 Is 26 Functions of HMAX layers Detecting triangles: layer 2 • Odd layers (layer 1, 3, 5, …) look for specific Neuron outputs 1 if desired image viewed, otherwise 0 combinations of lower-level features Layer 1: Specific edge at specific location Radial basis function Layer 2: Specific edge at slightly varied locations 푖푛 표푢푡 푟푎푑 h= 푤푗푟 푟 = (ℎ) 푗 푗 ) ℎ ( 푟푎푑 h • Even layers (layer 2, 4, 6, …) provide invariance to some feature changes (e.g., shift in max max position) Layer 2 Layer 2 표푢푡 푖푛 푖푛 푖푛 Horizontal edge at Diagonal edge at 푟 = max( 푟1 푟2 ⋯ 푟퐽 ) approximate approximate Layer 1 location location 27 28 6 4/23/2020 Detecting triangles: layer 3 Detecting triangles: layer 4 Neuron outputs 1 if desired image viewed, otherwise 0 Neuron outputs 1 if desired image viewed, otherwise 0 Layer 2: Specific edge at slightly varied locations Layer 3: Combination of edges Layer 3: Combination of edges Layer 4: Triangle on the left Accepted stimuli in Weighted sum layer 3 neuron 푤푖푟푖 max Layer 2 푖 Layer 4 Layer 3 Left triangle Triangle centered Layer 3 at fixed location 29 30 7.
Details
-
File Typepdf
-
Upload Time-
-
Content LanguagesEnglish
-
Upload UserAnonymous/Not logged-in
-
File Pages7 Page
-
File Size-