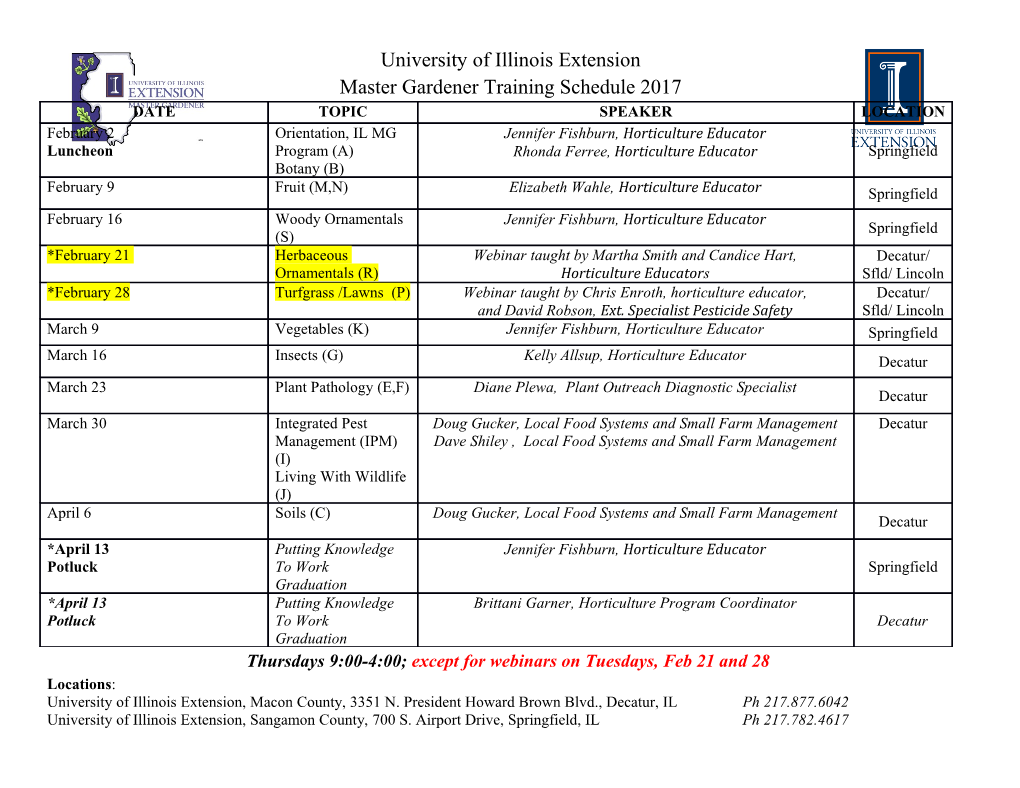
atmosphere Article Skill of Mesoscale Models in Forecasting Springtime Macrophysical Cloud Properties at the Savannah River Site in the Southeastern US Stephen Noble * , Brian Viner, Robert Buckley and Steven Chiswell Savannah River National Laboratory, Aiken, SC 29808, USA; [email protected] (B.V.); [email protected] (R.B.); [email protected] (S.C.) * Correspondence: [email protected] Received: 30 September 2020; Accepted: 3 November 2020; Published: 6 November 2020 Abstract: Predicting boundary layer clouds is important for the accurate modeling of pollutant dispersion. Higher resolution mesoscale models would be expected to produce better forecasts of cloud properties that affect dispersion. Using ceilometer observations, we assess the skill of two operational mesoscale models (RAMS and WRF) to forecast cloud base altitude and cloud fraction at the Savannah River Site in the southeastern US during the springtime. Verifications were performed at small spatial and temporal scales necessary for dispersion modeling. Both models were unreliable with a 50% (RAMS) and a 46% (WRF) rate of predicting clouds observed by the ceilometer which led to low cloud fraction predictions. Results indicated that WRF better predicted daytime cloud bases from convection that occurred frequently later in the period and RAMS better predicted nighttime cloud bases. Using root mean squared error (RMSE) to score the forecast periods also highlighted this diurnal dichotomy, with WRF scores better during the day and RAMS scores better at night. Analysis of forecast errors revealed divergent model cloud base biases—WRF low and RAMS high. A hybrid solution which weighs more heavily the RAMS nighttime forecasts and WRF daytime forecasts will likely provide the best prediction of cloud properties for dispersion. Keywords: clouds; cloud forecasting; RAMS; WRF; verification; cloud fraction; cloud base; dispersion 1. Introduction Scavenging and absorption of atmospheric particles and gases by boundary layer clouds affect the dispersion of pollutants, The processes of washout, chemical transformations, and sedimentation upon droplet evaporation of constituents all impact the rate of atmospheric removal or deposition. To predict the interactions between atmospheric aerosols and cloud droplets, models need to accurately predict clouds within the boundary layer. Large spatial or temporal variability in cloud field predictions will lead to incorrect localization of clouds that fail to correctly interact with the plume limiting dispersion predictions on small scales. The variable scale nature of clouds and cloud processes make them difficult to model. Small- scale interactions within clouds require state of the art microphysical and cumulus parameterizations [1] to estimate cloud properties for most mesoscale to global-scale meteorological simulations. Correctly parameterizing cloud processes is complicated by feedbacks by which clouds modify their own environment. For example, the formation of clouds modifies the local environment by moderating Earth’s energy balance and releasing latent heat to the atmosphere [2–4]. In dispersion modeling, clouds and precipitation drive wet deposition. Transformations of atmospheric aerosols and chemical constituents occur in clouds which, upon evaporation, leave altered aerosols [5] and modify airborne concentrations. Additionally, clouds moderate photochemistry Atmosphere 2020, 11, 1202; doi:10.3390/atmos11111202 www.mdpi.com/journal/atmosphere Atmosphere 2020, 11, 1202 2 of 16 Atmosphere 2020, 11, x FOR PEER REVIEW 2 of 16 photochemistryaffecting these constituentsaffecting these [6]. Theseconstituents interactions [6]. highlightThese interactions the importance highlight of accurate the importance cloud forecasts. of accurateRecent emphasiscloud forecasts. on high Recent frequency emphasis solar forecastingon high frequency to manage solar electricity forecasting loads to furthermanage demonstrates electricity loadsthe importantfurther demonstrates role of cloud the prediction important [role7]. Understandingof cloud prediction and [7] improving. Understanding cloudmodeling and improving aids to cloudimprove modeling functionality aids to improve for these functionality types of forecasts. for these types of forecasts. StudiesStudies that that assess assess cloud cloud forecasts forecasts are are primarily primarily performed performed for for cloud cloud cover cover (cloud (cloud fraction) fraction) and and cloudcloud ceiling ceiling (base) (base) height height necessary forfor aviation aviation safety safety [8 –[812–12]. Other]. Other studies studies focus focus on validatingon validatin cloudg cloudproperties properties from cloudfrom cloud parameterizations parameterizations for use infor large use scalein large climate scale models climate [13 models]. Most of[13] these. Most studies of theseuse modelsstudies withuse models grid spacing with grid greater spacing than 6greater km. Fewer than have6 km. used Fewer higher have resolution used higher models resolution(<2 km) modelsin these (<2 assessments km) in these [7 assessments,14], but note [7,14 that], higher but note resolution that higher models resolution performed models better performed at resolving better the atlower resolving boundary the lower layer boundary cloud base layer heights cloud (< base500 m)heights that might(<500 m) affect that dispersion. might affect dispersion. TheThe Savannah Savannah River NationalNational Laboratory Laboratory utilizes utilizes two two operational operational mesoscale mesoscale models, models, the Regional the RegionalAtmospheric Atmospheric Modeling Modeling System [System15] (RAMS) [15] (RAMS) and the Weatherand the ResearchWeather Research and Forecasting and Forecasting model [16 ] model(WRF), [16] to (WRF), produce to forecasts produce for forecasts the Savannah for the River Savannah Site (SRS) River located Site (SRS) in western located South in western Carolina South along Carolinathe Savannah along the River Savannah near Aiken, River SCnear (Figure Aiken1, ).SC These (Figure forecasts 1). These support forecasts a variety support of a sitevariety operations of site operationsincluding including identifying identifying weather patternsweather whichpatterns can which impact can site impact operations, site operations, assisting theassisting prescribed the prescribedburns conducted burns conducted by the US by Forest the US Service Forest at Service SRS and at drivingSRS and site driving dispersion site dispersion modeling modeling for emergency for emergencyresponse. response. Verification Verification studies of thestudies models of the used models for dispersion used for purposesdispersion [17 purposes] provide [17] confidence provide in confidenceconstituent in fateconstituent and transport fate and predictions. transport predictions. FigureFigure 1.1. LocationLocation and and size size of forecastforecast areaarea in in the the southeastern southeastern US US (state (state abbreviations abbreviations as noted). as noted) RAMS. RAMSinner inner domain, domain, red box; red WRF box; innerWRF domain,inner domai bluen, box; blue ceilometer box; ceilometer located located at black at cross black on cross the Savannah on the SavannahRiver Site River (SRS). Site (SRS). ObservationalObservational data data sets sets that that have have previously previously been been used used to to perform perform cloud cloud forecast forecast assessments assessments includeinclude satellite satellite data data (cloud (cloud fraction/mask fraction/mask and andbrightness brightness temperatures) temperatures) [8,13], [ 8c,loud13], cloudradar and radar lidar and networkslidar networks [9,11,18 [9], 11observ,18], observationation networks networks [14], [pyranometer14], pyranometer networks networks [7], [and7], and radiosondes radiosondes [12] [12. ]. TechniquesTechniques for for verifying verifying cloud cloud forecasts forecasts using satellite datadata includeinclude globalglobal analysis analysis to to compare compare with with 3 h 3 globalh global models models [8] and[8] gridand pointgrid point or area or comparisons area comparisons [7,13,19 ].[7,13,19 Verification]. Verification techniques techniques using observation using observationnetworks includenetworks area include averaging area averaging [11,14] and [11,14 probabilistic] and probabilistic comparisons comparisons [18]. Radiosonde [18]. Radiosonde relative relativehumidity humidity profiles profiles were also were used also to used verify to modelverify model cloud basecloud height base height [12]. [12]. InIn this this study study,, we we provide provide an an assessment assessment of of model model capabilities capabilities in inforecasting forecasting cloud cloud macrophysical macrophysical propertiesproperties that that affect affect atmospheric atmospheric constituent constituent dispersion dispersion at atfine fine spatial spatial and and temporal temporal scales scales.. W Wee use use 1515-min-min averaged averaged ceilometer ceilometer data data at atSRS SRS to to compare compare with with 15 15-min-min WRF WRF and and RAMS RAMS forecast forecast output output of of cloudcloud properties properties.. Comparisons Comparisons betweenbetweensingle single point point temporal temporal measurements measurements and instantaneousand instantaneous spatial spatialmeasurements measurements are highly are highly dependent dependent on resolution on resolution and advection and advection [14]. For [14] high-quality. For high dispersion-quality
Details
-
File Typepdf
-
Upload Time-
-
Content LanguagesEnglish
-
Upload UserAnonymous/Not logged-in
-
File Pages16 Page
-
File Size-