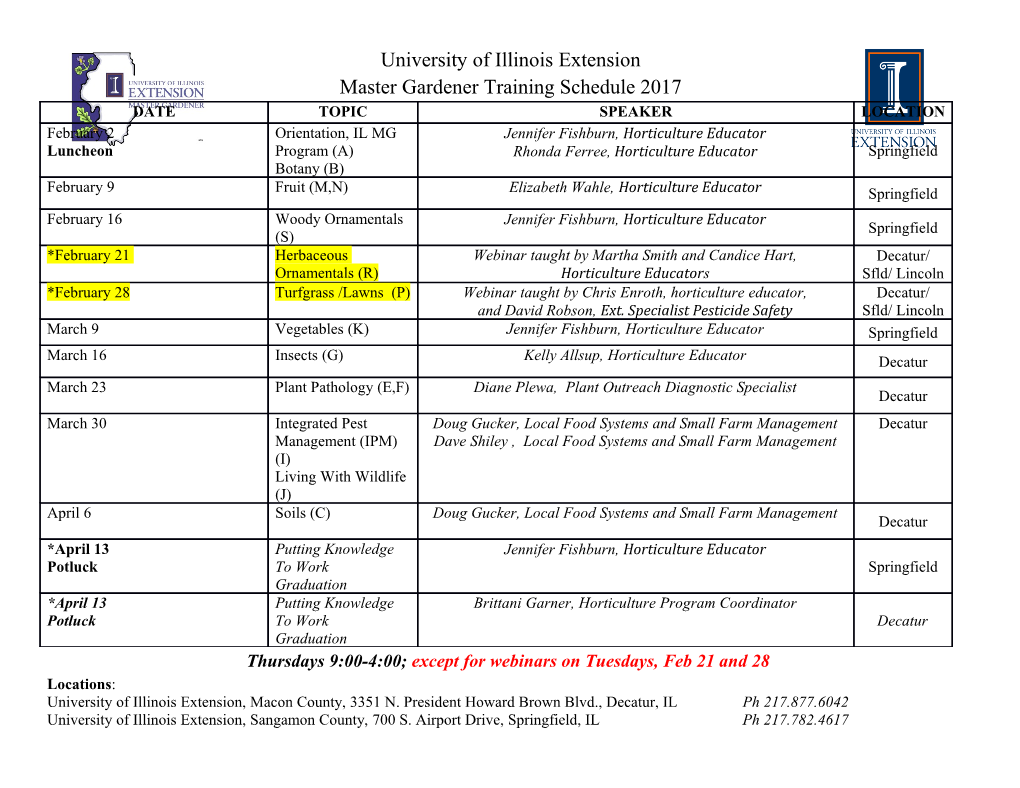
Uncorrected Proof Iran Red Crescent Med J. 2019 May; 21(5):e87526. doi: 10.5812/ircmj.87526. Published online 2019 June 11. Research Article Identification of a Novel Genetic Signature in Staging of Colorectal Cancer: A Bayesian Approach Fatemeh Mohammadzadeh 1, Ebrahim Hajizadeh 1, *, Aliakbar Rasekhi 1 and Sadegh Azimzadeh Jamalkandi 2 1Department of Biostatistics, Faculty of Medical Sciences, Tarbiat Modares University, Tehran, Iran 2Chemical Injuries Research Center, Systems Biology and Poisonings Institute, Baqiyatallah University of Medical Sciences, Tehran, Iran *Corresponding author: Department of Biostatistics, Faculty of Medical Sciences, Tarbiat Modares University, Tehran, Iran. Email: [email protected] Received 2018 December 14; Revised 2019 March 26; Accepted 2019 April 03. Abstract Background: Tumor stage is one of the most reliable prognostic factors in the clinical characterization of colorectal cancer. The identification of genes associated with tumor staging may facilitate the personalized molecular diagnosis and treatment along with better risk stratification in colorectal cancer. Objectives: The study aimed to identify genetic signatures associated with tumor staging and patients’ survival in colorectal cancer and recognize the patients’ risk category for clinical outcomes based on transcriptomic data. Methods: In this retrospective cohort study, two available transcriptomic datasets, including 232 patients with colorectal cancer under accession number GSE17537 and GSE17536 were used as discovery and validation sets, respectively. A Bayesian sparse group selection method in the discovery set was applied to identify the associated genes with the tumor staging. Then further screen- ing was performed using survival analysis, and significant genes were used to develop a gene signature model. Finally, the robust performance of the signature model was assessed in the validation set. Results: A total of 56 genes were significantly associated with the tumor staging in colorectal cancer. Survival analysis resulted in a shortlist of 19 genes, including ADH1B (P = 0.012), AHI (P = 0.006), AKAP12 (P = 0.018), BNIP3 (P = 0.015), CLDN11 (P = 0.015), CST9L (P = 0.028), DPP10 (P = 0.029), FBXO33 (P = 0.036), HEBP (P = 0.025), INTS4 (P = 0.003), LIPJ (P = 0.001), MMP21 (P = 0.006), NGRN (P = 0.014), PAFAH1B2 (P = 0.035), PCOLCE2 (P = 0.009), PIM1 (P = 0.007), TBKBP1 (P = 0.003), TCEB3B (P = 0.001), and TIPARP (P = 0.018), developing the signature model and validation. In both discovery and validation sets, the discrimination ability of the signature model to categorize patients with colorectal cancer into low- and high-risk subgroups for mortality and recurrence at 3- and 5-years showed good discrimination performances, with the area under the receiver operating characteristic curve (ROC) ranging from 0.64 to 0.88. It also had good sensitivity (discovery set 63.1%, validation set 61.7%) and specificity (discovery set 75.0%, validation set 59.3%) to discriminate between early- and late-stage groups. Conclusions: We identified a 19-gene signature associated with tumor staging and survival of colorectal cancer, which may repre- sent potential diagnosis and prognosis markers, and help to classify patients with colorectal cancer into low- or high-risk subgroups. Keywords: Bayesian Approach, Colorectal Cancer, Gene Expression Signatures, Microarray Analysis, Prognosis, Recurrence, Overall Survival, Tumor Staging, Classification,Archive Gene Ontology, Risk, Transcriptome of SID 1. Background sociated with the survival of patients with CRC and influ- ences the decision-making about the treatment plans (4). Colorectal cancer (CRC) is the third most frequent can- The tumor node metastasis (TNM) staging system is a tra- cer and the fourth leading cause of cancer-related death ditional approach to divide the cancer into four categories, worldwide (1). As a complex disease, CRC is affected by sev- namely I, II, III, and IV, based on the clinicopathologic fea- eral genetic and environmental factors (2). In recent years, tures, including tumor size, nodal spread, and metastasis. intensive studies have been conducted to provide more in- A higher stage is corresponding to a further progression sight into molecular alterations in the CRC. However, the of cancer and poorer clinical outcomes. Therefore, iden- molecular mechanisms underlying the CRC progression tification of genes associated with the TNM staging of the and tumor metastasis are still unclear. CRC can help to discover novel diagnostic and prognos- Identification of the tumor stage is currently the most tic biomarkers, and develop an improved prognostic tool reliable prognostic factor in the CRC (3). It is strongly as- Copyright © 2019, Author(s). This is an open-access article distributed under the terms of the Creative Commons Attribution-NonCommercial 4.0 International License (http://creativecommons.org/licenses/by-nc/4.0/) which permits copy and redistribute the material just in noncommercial usages, provided the original workwww.SID.ir is properly cited Uncorrected Proof Mohammadzadeh F et al. to optimize the personalized treatment and risk stratifica- tion of patients with CRC. Downloading mRNA expression data Currently, the systems biology approach in solving bi- and clinical information ological problems is a new approach that simultaneously considers all changes at different levels of the gene expres- sion. Meanwhile, there is a huge amount of transcrip- tomics data that can be explored using a variety of statis- Normalization and z-score transformation tical analysis methods or data mining techniques. SAM analysis with FDR<0.10 2. Objectives In this study, we attempted to identify a robust gene signature based on genes associated with the tumor stage Defining the group structure of genes for risk stratification of patients with CRC by taking into using K-means clustering and Gap statistic consideration the high correlation between genes in mi- croarray data through a Bayesian approach. Finally, the ro- bust performance of the signature model was validated in The Bayesian penalized ordered an independent cohort dataset. response model analysis 3. Methods Further screening using 3.1. Microarray Data and Data Preprocessing survival analysis In this retrospective cohort study, two previously published transcriptomics datasets, including 232 pa- Developing a gene signature model tients with colorectal cancer (5) under accession number GSE17537 and GSE17536 were used as discovery and vali- dation sets, respectively in the Gene Expression Omnibus (GEO) (www.ncbi.nlm.nih.gov/geo) for which met the fol- Validation lowing inclusion criteria: (a) being human gene expres- sion data, (b) profiled on the Affymetrix HG-U133_Plus_2 Figure 1. Flow chart of the analysis procedure, including data collection, prepro- platform, (c) information about age, sex, pathological tu- cessing, analysis, and validation mor staging (stage I-IV), death status, and survival time. De- tails of protocols and procedures of data collection, instru- ments, and variables measurement are available (5). Raw 3.3. Detection of Associated Genes with the TNM-Staging of CRC data of both datasets were downloaded and normalized us- in the Discovery Set ing the Robust Multi-array Average (RMA) algorithm (6). After differential gene expression analysis, the parallel The Z-score transformed was used to standardize the ex- line assumption was tested using a score test in a univari- pression value of each gene. Archiveate orderedof probit SID model for each gene. Then a multiple penalized ordinal probit model was used to discover the as- 3.2. Statistical Analysis sociated genes with the TNM-staging of patients with CRC. In this model, we used a Bayesian sparse group selection All analyses were performed using R programming (BSGS) method for variable selection, which took into ac- software version 3.5.0. Figure 1 shows the workflow of the count the group structures between genes in microarray study. At first, primary screening was performed using the data. The BSGS method was proposed by Xu and Ghosh (9) significance analysis of microarrays (SAM) algorithm (7) for a linear model. They showed the superior accuracy and available in “siggenes” package (8) to detect differentially excellent performance of this method for variable selec- expressed genes (DEG) between the different stages of CRC tion and prediction by simulation. In the BSGS method, it in the discovery set (GSE17537). A false discovery rate (FDR) is assumed that predictors to be partitioned into G groups < 0.1 was set for identification DEGs. and spike and slab priors are used to select variables at 2 Iran Red Crescent Med J. 2019;www.SID.ir 21(5):e87526. Uncorrected Proof Mohammadzadeh F et al. both group and within-group levels. The formulation of also investigated the association between the gene signa- the prior for the coefficient vector of the gth group can be ture model and tumor stages of patients with CRC. The ROC written as (9): curve analysis was applied to quantify how accurately the 1 gene signature model can discriminate between two early- 2 βg = Vg bg ; and late-stage groups (I and II stages vs. III and IV stages). The age- and gender-independent prognostic value of the 1 2 n o signature were also evaluated using multivariate Cox re- where Vg = diag τg1; : : : ; τgmg gression models. We validated the gene signature model τ gj ≥ 0, using the independent GSE17536 dataset. To this end, the g = 1, …, G, same gene signature model and the cut-off value were used j =
Details
-
File Typepdf
-
Upload Time-
-
Content LanguagesEnglish
-
Upload UserAnonymous/Not logged-in
-
File Pages9 Page
-
File Size-