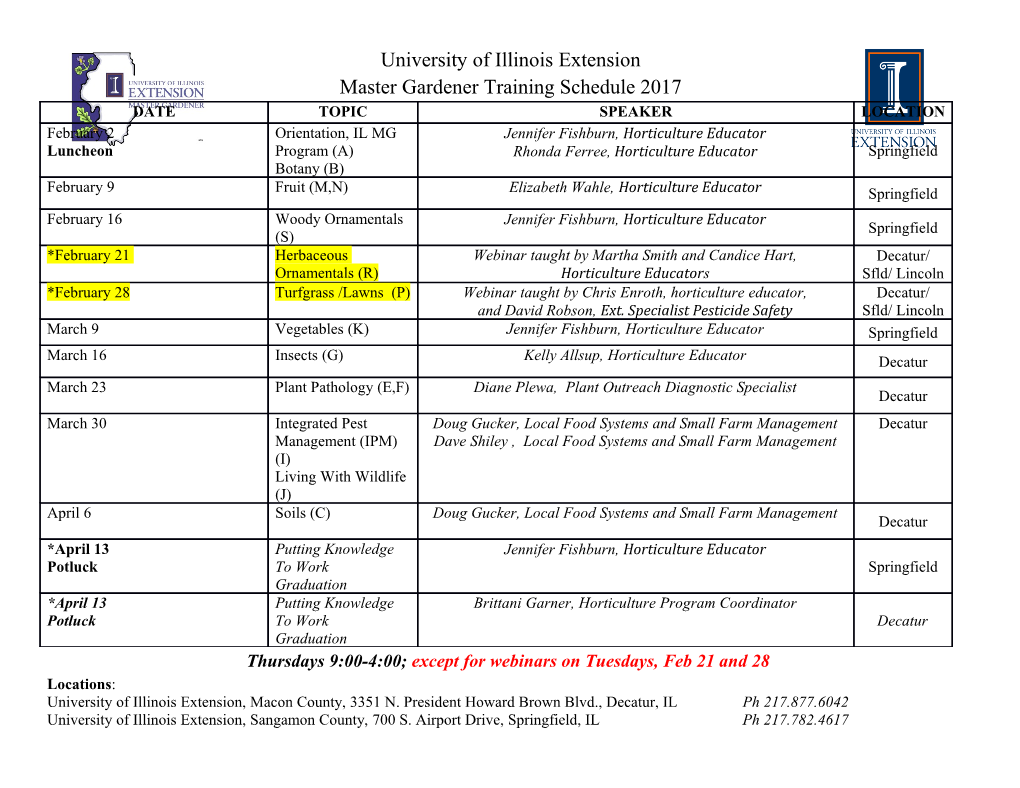
Reprod Dev Biol 37(4) : 185-192 (2013) ISSN : 1738-2432 (P r i n t) http://dx.doi.org/10.12749/RDB.2013.37.4.185 ISSN : 2288-0151 (Online) Gene Co-Expression Network Analysis of Reproductive Traits in Bovine Genome Dajeong Lim1,†, Yong-Min Cho1, Seung-Hwan Lee2, Han-Ha Chai1 and Tae-Hun Kim1 1Division of Animal Genomics and Bioinformatics, National Institute of Animal Science, RDA, Suwon 441-706, Korea 2Hanwoo Experiment Station, National Institute of Animal Science, RDA, Pyungchang, 232-950, Korea ABSTRACT Many countries have implemented genetic evaluation for fertility traits in recent years. In particular, reproductive trait is a complex trait and need to require a system-level approach for identifying candidate genes related to the trait. To find the candidate gene associated with reproductive trait, we applied a weighted gene co-expression network ana- lysis from expression value of bovine genes. We identified three co-expressed modules associated with reproductive trait from bovine microarray data. Hub genes (ZP4, FHL2 and EGR4) were determined in each module; they were topologically centered with statistically significant value in the gene co-expression network. We were able to find the highly co-expressed gene pairs with a correlation coefficient. Finally, the crucial functions of co-expressed modules were reported from functional enrichment analysis. We suggest that the network-based approach in livestock may an important method for analyzing the complex effects of candidate genes associated with economic traits like repro- duction. (Key words : Reproduction, Gene Co-expression Network (GCN), Cattle) INTRODUCTION These networks provide information on the genetic con- trol of complex traits and can help identify causal ge- nes that affect gene function rather than gene expre- A good reproductive performance is crucial for eco- ssion (Haley and de Koning, 2006). System-oriented app- nomic as well as ethical reasons. Without reproduction roaches have been applied by animal geneticists to in- there will be no animal production. Therefore, In recent vestigate livestock traits (Nobis et al., 2003; Donaldson years many other countries have also implemented ge- et al., 2005; Smith and Rosa, 2007), resulting in the iden- netic evaluation for reproductive traits. The cattle bree- tification and characterization of economically impor- ding objectives has gradually shifted from production tant causal trans-acting genes within QTL regions. The- to functional traits such as pregnancy. From this point se trans-QTL regions share a common biological func- of view it is important to increase knowledge of genes tion (e.g., similar gene ontology function, metabolic pa- and their regulation. Reproduction trait is also a com- thway, transcriptional co-regulation) (Schadt et al., 2003; plex trait, which is contributed from many genes. There- Gibson and Weir, 2005; Subramanian et al., 2005). In fore, it is the agreement that no single factor deter- the case of bovines, several countries identify quality cha- mines a large proportion of the trait variation in the llenges. Three genes were identified as being significan- population (Hwang et al., 2008). From this reason, sys- tly correlated with bovine skeletal muscle based on mi- tems biology approach has been useful to identify ge- croarray data from a gene network (Reverter et al., 2006). nes that underlie complex trait from network of genetic Jiang et al. (2009) (Jiang et al., 2009) reported that the interactions among all possible genes. Furthermore, pat- genetic network was associated with 19 economically terns of covariation in the expression of multiple loci important beef traits. This report suggested the three can- can be used to build networks that show relationships didate gene approach as targets. Therefore, we need to between genes, and between genes and functional traits. systemic approach in order to identify candidate genes * To whom correspondence should be addressed. * This work was supported by Agenda (PJ906956) of the National Institute of Animal Science, Rural Development Administration (RDA), Republic of Korea. †Corresponding author : Phone: +82-31-290-1606, E-mail: [email protected] 185 186 Lim et al. in the network analysis among many genes related to used 221 genes associated with pregnancy rate for net- reproduction within QTL intervals. A gene co-express- work construction. ion network (GCN) is a gene correlation network crea- ted from expression profiling, with each gene having Gene Co-Expression Network Construction and Net- several neighbors and is useful for identifying genes that work Module Identification control quantitative phenotypes. In this study, we in- In co-expression networks, we refer to nodes as ge- troduce a systemic approach involving network analy- nes whose degrees indicate the number of links con- sis of reproduction-related genes and suggest co-expre- nected by the node. We extracted expression values for ssed pattern of hub genes in the network. 221 genes and evaluated pairwise correlations between the gene expression profiles of each pair of genes us- ing Pearson’s correlation coefficients (denoted as r). The MATERIALS AND METHODS unweighted network encoded gene co-expression as bi- nary information (connected=1, unconnected=0) using a “hard” threshold. In contrast, the weighted network re- Our analysis involved three main steps: finding can- presented “soft” thresholding that weighed each con- didate genes in the Animal QTL database and analyz- nection as a continuous number [0, 1]. The power ad- ing the results of microarray experiments from the Ge- β jacency function α =|cor(x , x ) was used to construct ne Expression Omnibus (GEO) database, using these ge- i,j i j a weighted network as the connection strength between nes and co-expression information to construct co-ex- two genes. We investigated soft thresholding with the pression networks related to the “pregnancy rate” trait power adjacency function and selected a power of beta and analyzing the network topology and functional en- (β)=7. A major aim of co-expression network analysis richment for modules using DAVID tool and CytoSca- is to determine subsets of nodes (modules) that are ti- pe. ghtly connected to each other. To organize genes into modules, we used a module identification method ba- Identification of Candidate Genes Associated with the sed on a topological overlap dissimilarity measure (Ra- Pregnancy Rate vasz et al., 2002) in conjunction with a clustering me- To determine candidate genes associated with the re- thod, which detected biologically meaningful modules. production within QTL intervals, we obtained genomic The topological overlap of two nodes refers to their re- positions of the “pregnancy rate” trait using “QTL lo- lative interconnectedness. The topological overlap ma- cation by bp” information from the Animal QTL data- trix (TOM) Ω=[ωij] provides a similarity measure, whi- base (http://www.genome.iastate.edu/cgi-bin/QTLdb/BT/ ch has proven useful in biological networks (Ye and index). Most of QTLs are identified in the different re- Godzik, 2004), where lij=uαiu αuj and ki=uαiu is the gions in a chromosome. There are rare regions of over- node connectivity as follows: lap. Therefore, we select the genes associated with pre- gnancy rate from Animal QTL database with QTL IDs that have marker information in term of ‘pregnancy rate’ within Animal Trait Ontology (ATO) category. In In the case of our network, l equals the number of the GEO database (http://www.ncbi.nlm.nih.gov/geo/), all ij nodes to which both i and j are connected. To identify data from microarray experiments related to bovines — were used: GEO series (GSE) 15544, GSE 15342, GSE 13- modules, we used TOM-based dissimilarity ( =1 725, GSE 6918, GSE 10695, GSE 12327, GSE 9256, GSE in a hierarchical cluster analysis. Each module rep- 12688, GSE 11495, GSE 11312, GSE 7360, GSE 9344, and resents a group of genes with similar expression pro- GSE 8442. All arrays were processed to determine the files across the samples and the expression profile pa- robust multiarray average (RMA) (Irizarry et al., 2003) ttern is distinct from those of other modules. The wei- using the “affy” software package (Gautier et al., 2004). ghted gene co-expression analysis (WGCNA) software Expression values were computed in detail from raw packages for R were used to identify co-expression val- CEL files by applying the RMA model of probe-specific ues associated with pregnancy rate (Peter and Steve, correction for perfect-match probes. These corrected 2008). The degree distribution of a network has a ge- probe values were then subjected to quantile normal- neralized power-law form p(k)~k— r, which is the defin- ization, and a median polish was applied to compute one ing property of a scale-free network (Barabasi and Al- expression measure from all probe values. Resulting bert, 1999). The genes of highly connected nodes to no- RMA expression values were log2-transformed. We de- des with few connections (hubs) play an important role termined mean intensity for an expression intensity of as a local property in a network (Barabasi and Oltvai, each gene matching to at least two probes. Finally, we 2004). A node with high BC has great influence over Co-Expression Network of Reproductive Trait in Cattle 187 Scale independence Mean connectivity Scale independence Mean connectivity what flows in the network; BC may play a major role Scale independence Mean connectivity as a global property since it is a useful indicator for 7 8 1 8 50 1 0.8 7 detecting bottlenecks in a network. For node k, BC is 6 50 0.8 7 8 1 5 6 50 the fraction of the number of shortest paths that pass 0.8 5 6 40 through each node (Brandes, 2001) and is defined as 0.6 5 4 40 0.6 4 40 0.6 4 30 , 0.4 30 0.4 ScaleTopologysignedFree R^2 ModelFit, connectivity Mean 30 ScaleTopologysignedFree R^2 ModelFit, connectivity Mean 0.4 3 10 12 14 16 18 20 → ScaleTopologysignedFree R^2 ModelFit, 9 10 16 connectivity Mean where g is the number of the shortest geodesic paths 0.2 12 14 18 20 20 i j 3 9 0.2 10 12 14 16 18 20 20 2 from node i to node j, is the number of geodesic 3 9 2 0.2 20 2 → paths among gi j from node i to node j that pass th- 0.0 2 10 0.0 rough node k.
Details
-
File Typepdf
-
Upload Time-
-
Content LanguagesEnglish
-
Upload UserAnonymous/Not logged-in
-
File Pages8 Page
-
File Size-