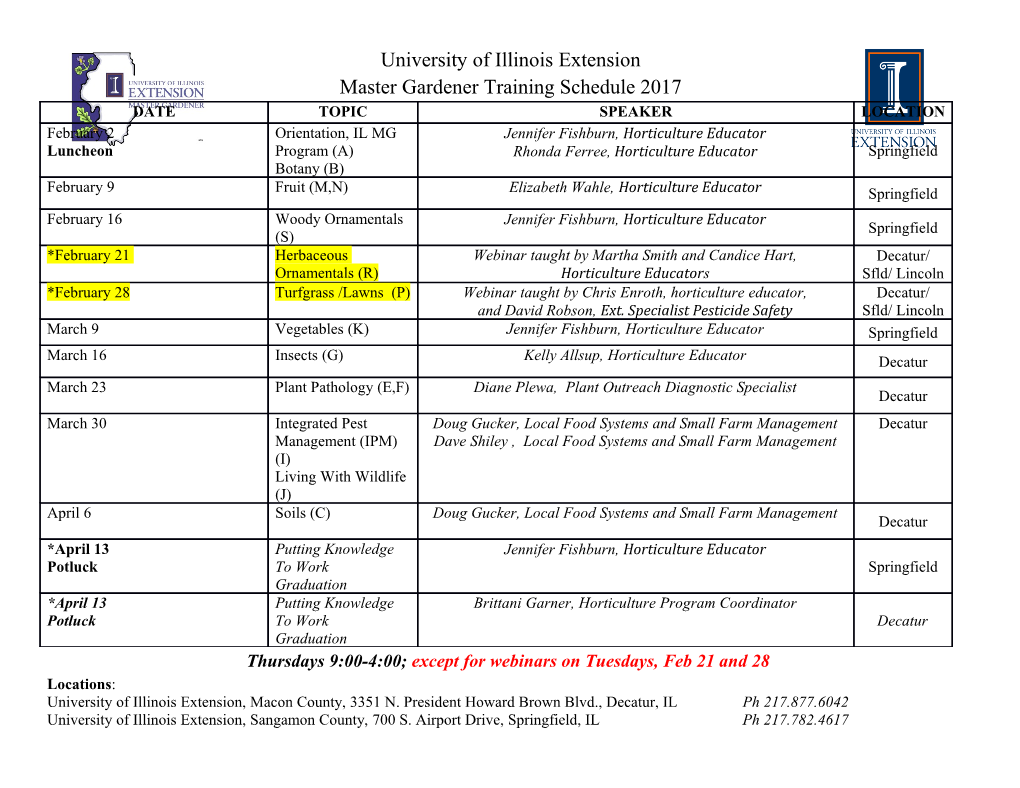
Sparse Optimization Lecture: Dual Methods, Part I Instructor: Wotao Yin July 2013 online discussions on piazza.com Those who complete this lecture will know dual (sub)gradient iteration • augmented ` iteration (linearzed Bregman iteration) • 1 dual smoothing minimization • augmented Lagrangian iteration • Bregman iteration and addback iteration • 1 / 39 Review Last two lectures studied explicit and implicit (proximal) gradient updates • derived the Lagrange dual problem • overviewed the following dual methods • 1. dual (sub)gradient method (a.k.a. Uzawa’s method) 2. dual proximal method (a.k.a., augmented Lagrangian method (ALM)) 3. operator splitting methods applied to the dual of min f(x) + g(z): Ax + Bz = b x,z { } The operator splitting methods studied includes forward-backward splitting • Peaceman-Rachford splitting • Douglas-Rachford splitting (giving rise to ADM or ADMM) • This lecture study these dual methods in more details and present their applications to sparse optimization models. 2 / 39 About sparse optimization During the lecture, keep in mind that sparse optimization models typically have one or more nonsmooth yet simple part(s) and one or more smooth parts with dense data. Common preferences: to the nonsmooth and simple part, proximal operation is preferred over • subgradient descent if smoothing is applied, exact smoothing is preferred over inexact • smoothing to the smooth part with dense data, simple (gradient) operator is preferred • over more complicated operators when applying divide-and-conquer, fewer divisions and simpler • subproblems are preferred In general, the dual methods appear to be more versatile than the primal-only methods (e.g., (sub)gradient and prox-linear methods) 3 / 39 Dual (sub)gradient ascent Primal problem min f(x), s.t. Ax = b. x Lagrangian relaxation: (x; y) = f(x) + yT (Ax b) L − Lagrangian dual problem min d(y) or max d(y) y y − If g is differentiable, you can apply yk+1 yk ck d(yk) ← − ∇ otherwise, apply yk+1 yk ckg, where g ∂d(yk). ← − ∈ 4 / 39 Dual (sub)gradient ascent Derive d or ∂d ∇ by hand, or • k k k k use (x; y ): compute x minx (x; y ), then b Axˉ ∂d(y ). • L ← L − ∈ Iteration: xk+1 min (x; yk), ← x L yk+1 yk + ck(Axk+1 b). ← − Application: augmented `1 minimization, a.k.a. linearized Bregman. 5 / 39 Augmented `1 minimization 2 Augment `1 by `2: 1 2 (L1+LS) min x 1 + x s.t. Ax = b k k 2α k k2 primal objective becomes strongly convex (but still non-differentiable) • hence, its dual is unconstrained and differentiable • a sufficiently large but finite α leads the exact ` solution • 1 related to: linearized Bregman algorithm, the elastic net model • a test with Gaussian A and sparse x with Gaussian entries: • min x : Ax = b min x 2 : Ax = b min x + 1 x 2 : Ax = b {k k1 } {k k2 } {k k1 25 k k2 } Exactly the same as `1 solution 6 / 39 Lagrangian dual of (L1+LS) Theorem (Convex Analysis, Rockafellar [1970]) If a convex program has a strictly convex objective, it has a unique solution and its Lagrangian dual program is differentiable. Lagrangian: (separable in x for fixed y) 1 2 T (x; y) = x 1 + x y (Ax b) L k k 2α k k2 − − Lagrange dual problem: α 2 min d(y) = b>y + A>y Proj[ 1,1]n (A>y) 2 y − 2 k − − k note: shrink(x, γ) = max x γ, 0 sign(x) = x Proj[ γ,γ](x). {| | − } − − Objective gradient: d(y) = b + αA shrink(A>y) ∇ − Dual gradient iteration: k+1 k x = α shrink(A>y ), yk+1 = yk + ck(b Axk+1). − 7 / 39 How to choose α 0 0 Exact smoothing: a finite α so that all α > α lead to `1 solution • ∃ 1 In practice, α = 10 xsol suffices , with recovery guarantees under RIP, • k k∞ NSP, and other conditions. 0 Although α > α lead to the same and unique primal solution x∗, the dual • solution set ∗ is a multi-set and it depends on α. Y Dynamically adjusting α may not be a good idea for the dual algorithms. • 1Lai and Yin [2012] 8 / 39 Exact regularization Theorem (Friedlander and Tseng [2007], Yin [2010]) There exists a finite α0 > 0 such that whenever α > α0, the solution to 1 2 (L1+LS) min x 1 + x s.t. Ax = b k k 2α k k2 is also a solution to (L1) min x 1 s.t. Ax = b. k k L1 L1+LS 9 / 39 L1+LS in compressive sensing If in some scenario, L1 gives exact or stable recovery provided that #measurements m C F (signal dim n, signal sparsity k). ≥ ∙ Then, adding 1 x 2, the condition becomes 2α k k2 1 #measurements m (C + O( )) F (signal dim n, signal sparsity k). ≥ 2α ∙ Theorem (exact recovery, Lai and Yin [2012]) Under the assumptions 0 1. x is k-sparse, and A satisfies RIP with δ2k 0.4404, and ≤ 2. α 10 x0 , ≥ k k∞ (L1+LS) uniquely recovers x0. The bound on δ2k is tighter than that for `1, and it depends on the bound on α. 10 / 39 Stable recovery For approximately sparse signals and/or noisy measurements, consider: 1 2 min x 1 + x 2 : Ax b 2 σ (1) x k k 2α k k k − k ≤ Theorem (stable recovery, Lai and Yin [2012]) Let x0 be an arbitrary vector, = largest k entries of x0 , and = C . Let 0 S { } Z S b := Ax + n, where n is arbitrary noisy. If A satisfies RIP with δ2k 0.3814 0 ≤ and α 10 x , then the solution x∗ of (1) with σ = n 2 satisfies ≥ k k∞ k k 0 0 x∗ x 2 Cˉ1 n 2 + Cˉ2 x 1/√k, k − k ≤ ∙ k k ∙ k Z k where Cˉ1, and Cˉ2 are constants depending on δ2k. 11 / 39 Implementation Since dual is C1 and unconstrained, various first-order techniques apply • accelerated gradient descent2 • Barzilai-Borwein step size3 / (non-monotone) line search4 • Quasi Newton method (with some cautions since it is not C2) • Fits the dual-decomposition framework, easy to parallelize (later lecture) • Results generalize to ` -like functions. • 1 Matlab codes and demos at • www.caam.rice.edu/~optimization/linearized_bregman/ 2Nesterov [1983] 3Barzilai and Borwein [1988] 4Zhang and Hager [2004] 12 / 39 Review: convex conjugate Recall convex conjugate (the Legendre transform): T f ∗(y) = sup y x f(x) x domf{ − } ∈ f ∗ is convex since it is point-wise maximum of linear functions if f is proper, closed, convex, then (f ∗)∗ = f, i.e., T f(x) = sup y x f ∗(y) . y domf { − } ∈ ∗ Examples: T f(x) = ι (x), indicator function, and f ∗(y) = supx y x, support • C ∈C function f(x) = ι 1 x 1 and f ∗(y) = y 1 • {− ≤ ≤ } k k f(x) = ι x 1 and f ∗(y) = y 2 • {k k2≤ } k k lots of smooth examples ...... • 13 / 39 Review: convex conjugate One can introduce an alternative representation via convex conjugacy T f(x) = sup y (Ax + b) h∗(y) = h(Ax + b). y domf { − } ∈ ∗ Example: let = y = [y1; y2]: y1 + y2 = 1, y1, y2 0 and • C { ≥ } 1 A = . 1 "− # T T Since x 1 = sup (y1 y2) x ι (y) = sup y Ax ι (y) , we k k y{ − − C } y{ − C } have x 1 = ι∗ (Ax) k k C where ι∗ ([x1; x2]) = max x1, x2 entry-wise. C { } 14 / 39 Dual smoothing Idea: strongly convexify h∗ = f becomes differentiable and has Lipschitz f ⇒ ∇ Represent f using h∗: T f(x) = sup y (Ax + b) h∗(y) y domh { − } ∈ ∗ Strongly convexify h∗ by adding strongly convex function d: hˆ∗(y) = h∗(y) + μd(y) Obtain a differentiable approximation: T fμ(x) = sup y (Ax + b) hˆ∗(y) y domh { − } ∈ ∗ fμ(x) is differentiable since h∗(y) + μd(y) is strongly convex. 15 / 39 Example: augmented `1 primal problem min x 1 : Ax = b • {k k } T T dual problem: max b y + ι[ 1,1]n (A y) • { − } f(y) = ι[ 1,1]n (y) is non-differentiable • − T let f ∗(x) = x 1 and represent f(y) = sup y x f ∗(x) , • k k x{ − } μ add x 2 to f ∗(x) and obtain • 2 k k T μ 2 1 2 fμ(y) = sup y x ( x 1 + x 2) = y Proj[ 1,1]n (y) 2 x { − k k 2 k k } 2μ k − − k 1 fμ(y) is differentiable; fμ(y) = shrink(y). • ∇ μ On the other hand, we can also smooth f ∗(x) = x 1 and obtain • k k differentiable fμ∗(x) by adding d(y) to f(y). (see the next slide ...) 16 / 39 Example: smoothed absolute value Recall f ∗(x) = x = sup yx ι[ 1,1](y) | | y { − − } Let d(y) = y2/2 2 2 x /(2μ), x μ, fμ∗ = sup yx (ι[ 1,1](y) + μy /2) = | | ≤ y { − − } x μ/2, x > μ, | | − | | which is the Huber function let d(y) = 1 1 y2 − − p 2 2 2 fμ∗ = sup yx (ι[ 1,1](y) μ 1 y ) μ = x + μ μ. y { − − − − } − − p p Recall x = sup (y1 y2)x ι (y) | | y { − − C } for = y : y1 + y2 = 1, y1, y2 0 . Let d(y) = y1 log y1 + y2 log y2 + log 2 C { ≥ } x/μ x/μ e + e− fμ∗(x) = sup (y1 y2)x (ι (y) + μd(y)) = μ log . y { − − C } 2 17 / 39 Compare three smoothed functions Courtesy of L. Vandenberghe 18 / 39 Example: smoothed maximum eigenvalue Let = Y n : trY = 1, Y 0 . Let X n C { ∈ S } ∈ S f(X) = λmax(X) = sup Y X ι (Y) Y { • − C } Negative entropy of λi(Y) : { } n d(Y) = λi(Y) log λi(Y) + log n i=1 X (Courtesy of L.
Details
-
File Typepdf
-
Upload Time-
-
Content LanguagesEnglish
-
Upload UserAnonymous/Not logged-in
-
File Pages41 Page
-
File Size-