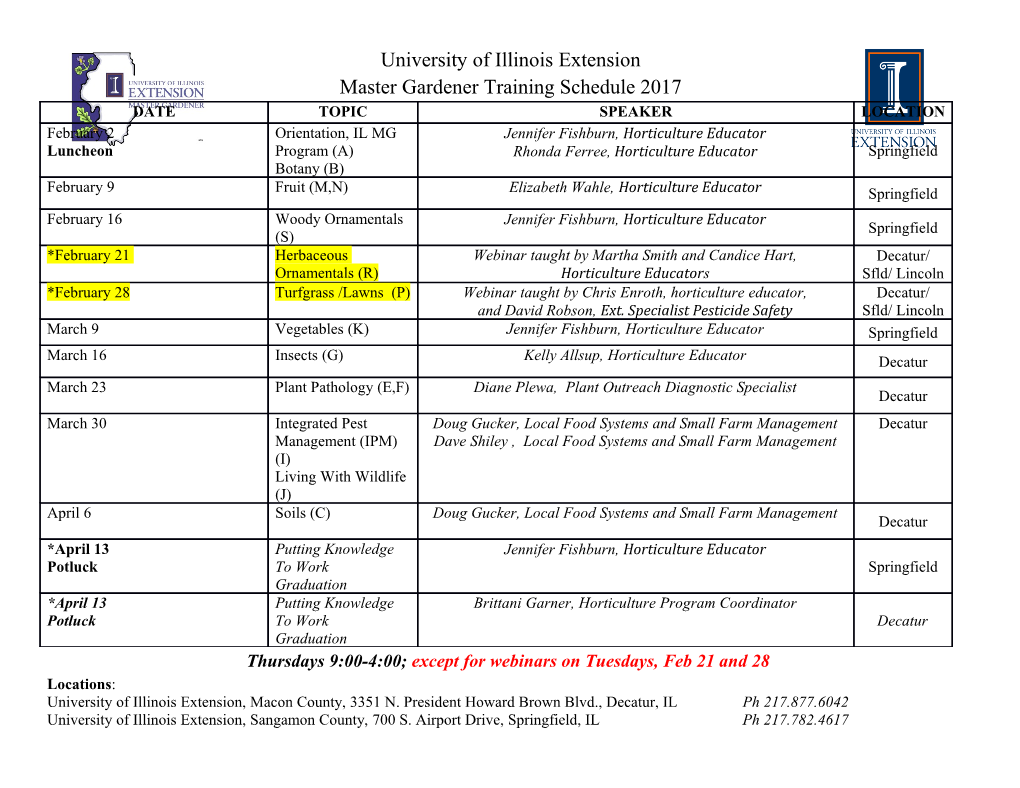
City University of New York (CUNY) CUNY Academic Works Publications and Research Kingsborough Community College 2016 Generalized Least-Powers Regressions I: Bivariate Regressions Nataniel Greene CUNY Kingsborough Community College How does access to this work benefit ou?y Let us know! More information about this work at: https://academicworks.cuny.edu/kb_pubs/105 Discover additional works at: https://academicworks.cuny.edu This work is made publicly available by the City University of New York (CUNY). Contact: [email protected] INTERNATIONAL JOURNAL OF MATHEMATICAL MODELS AND METHODS IN APPLIED SCIENCES Volume 10, 2016 Generalized Least-Powers Regressions I: Bivariate Regressions Nataniel Greene of this paper is on even values of p, since this case allows Abstract— The bivariate theory of generalized least-squares is for the derivation of analytic formulas to nd the regression extended here to least-powers. The bivariate generalized least- coefcients, analogous to what was done for least-squares. powers problem of order p seeks a line which minimizes the average generalized mean of the absolute pth power deviations between the The numerical example presented illustrates that bivariate data and the line. Least-squares regressions utilize second order generalized least-powers methods perform comparably to gen- moments of the data to construct the regression line whereas least- eralized least-squares methods but have a greater range of powers regressions use moments of order p to construct the line. slope values. The focus is on even values of p, since this case admits analytic so- lution methods for the regression coefcients. A numerical example shows generalized least-powers methods performing comparably to II. BIVARIATE ORDINARY AND GENERALIZED generalized least-squares methods, but with a wider range of slope LEAST-POWERS REGRESSION values. A. Bivariate Ordinary Least-Powers Regression OLP Keywords—Least-powers, generalized least-powers, least-squares, p generalized least-squares, geometric mean regression, orthogonal The generalization of ordinary least-squares regression regression, least-quartic regression. (OLS) to arbitrary powers is called ordinary least-powers regression of order p and is denoted here by OLPp. OLS I. OVERVIEW is the same thing as OLP2. The case of OLP4, called least- quartic regression, is described in a paper by Arbia [1]. OR two variables x and y ordinary least-squares y x j Denition 1: (The Ordinary Least-Powers Problem) Values F regression suffers from a fundamental lack of symmetry. of a and b are sought which minimize an error function dened It minimizes the distance between the data and the regression by line in the dependent variable y alone. To predict the value N of the independent variable x one cannot simply solve for 1 p E = a + bxi yi : (1) N j j this variable using the regression equation. Instead one must i=1 derive a new regression equation treating x as the dependent X variable. This is called ordinary least-squares x y regression. The resulting line y = a + bx is called the ordinary least- j powers y x regression line. The fact that there are two ordinary least-squares lines to j model a single set of data is problematic. One wishes to The explicit bivariate formula for the ordinary least-squares have a single linear model for the data, for which it is valid error described by Ehrenberg [3] is generalized now to higher- to solve for either variable for prediction purposes. A theory order regressions using generalized product-moments. of generalized least-squares was developed by this author to Denition 2: Dene the generalized bivariate product- overcome this problem by minimizing the average generalized moment of order p = m + n as mean of the square deviations in both x and y variables [5]– N 1 m n [8]. For the resulting regression equation, one can solve for = (xi ) yi (2) m;n N x y x in terms of y in order to predict the value of x: This theory i=1 was subsequently extended to multiple variables [9]. X for whole numbers m and n. In this paper, the extension of the bivariate theory of gen- Theorem 3: (Explicit Bivariate Error Formula) Let p be an eralized least-squares to least-powers is begun. The bivariate even whole number and let F = E a= b : Then generalized least-powers problem of order p seeks a line which j y x minimizes the average generalized mean of the absolute pth p t power deviations between the data and the line. Unlike least- E = ( 1)s br a b (3) r; s; t r;s y x squares regressions which utilize second order moments of r+s+t=p X the data to construct the regression line, least-pth powers or regressions utilize moments of order p to construct the line. p t In the interest of generality, the denitions here are for- E = ( 1)s br a b + F r; s; t r;s y x mulated using arbitrary powers p. Nevertheless, the focus r+s+t=p;t=0 X 6 N. Greene is with the Department of Mathematics and Computer Science, where Kingsborough Community College, City University of New York, 2001 p p r p r Oriental Boulevard, Brooklyn, NY 11235 USA (phone: 718-368-5929; e-mail: F = ( 1) r;p rb : (4) r [email protected]). r=0 X ISSN: 1998-0140 352 INTERNATIONAL JOURNAL OF MATHEMATICAL MODELS AND METHODS IN APPLIED SCIENCES Volume 10, 2016 Proof: Assume p is even and omit absolute values. Begin For p = 4, with the error expression and manipulate as follows: 4 3 2 F = 4;0b 43;1b + 62;2b 41;3b + 0;4: (7) N 1 p E = (a + bxi yi) For p = 6, N i=1 F = b6 6 b5 + 15 b4 20 b3 XN 6;0 5;1 4;2 3;3 1 p 2 = b (xi ) yi + a b +152;4b 61;5b + 0;6: N x y y x i=1 (8) XN 1 p Theorem 5: (Bivariate OLP Regression) The OLPp y x = ( 1)s br j N r; s; t regression line y = a + bx is obtained by solving i=1 r+s+t=p p X X s t r p r p (xi ) yi a b r 1 x y y x F 0 (b) = ( 1) r r;p rb = 0 (9) r s p r=1 = ( 1) br X r; s; t r+s+t=p for b and setting X N 1 r s t a = y bx: (xi ) yi a b N x y y x (10) ( i=1 ) X Example 6: For p = 2, which is least-squares, one solves p t = ( 1)s br a b : r; s; t r;s y x 0 = b : (11) r+s+t=p 2;0 1;1 X Now separate out the terms with t = 0 and obtain For p = 4 one solves 3 2 s p t 0 = b 3 b + 3 b : (12) E = ( 1) br a b + F 4;0 3;1 2;2 1;3 r; s; t r;s y x r+s+t=p;t=0 For p = 6 one solves X 6 where 0 = b5 5 b4 + 10 b3 6;0 5;1 4;2 p 2 F = ( 1)s br 103;3b + 52;4b 1;5: r; s; 0 r;s r+s=p (13) Xp Now that the analog of OLS, called OLP, has been de- p r p r = ( 1) r;p rb : scribed, the corresponding bivariate theory of generalized r r=0 least-powers can be described as well. X Observe that applying the trinomial expansion theorem to the error expression E and then setting a = y bx produces the B. Bivariate Generalized Means and XMRp Notation same result F as rst setting a = b and then applying y x The axioms of a generalized mean were stated by us the binomial expansion: previously [8], [9] drawing from the work of Mays [11] N and also from Chen [2]. They are stated here again for 1 p F = b (xi ) yi convenience. N x y i=1 Denition 7: A function M (x; y) denes a generalized X N p mean for x; y > 0 if it satises Properties 1-5 below. If 1 p r r p r p r = b (xi ) yi ( 1) N r x y it satises Property 6 it is called a homogeneous generalized i=1 r=0 mean. The properties are: p X X p r p = ( 1) 1. (Continuity) M (x; y) is continuous in each variable. r 2. (Monotonicity) M (x; y) is non-decreasing in each vari- r=0 X N able. 1 r p r r (xi ) yi b 3. (Symmetry) M (x; y) = M (y; x) : N x y ( i=1 ) 4. (Identity) M (x; x) = x: p X 5. (Intermediacy) min (x; y) M (x; y) max (x; y) : p r p r = ( 1) r;p rb : 6. (Homogeneity) M (tx; ty) = tM (x; y) for all t > 0: r r=0 X All known means are included in this denition. All the means discussed in this paper are homogeneous. The Example 4: For p = 2, which is least-squares, generalized mean of any two generalized means is itself a generalized mean. F = b2 2 b + : (5) 2;0 1;1 0;2 XMRp notation is used here to name generalized regres- In more familiar notation this is sions: if `X' is the letter used to denote a given generalized mean, then XMRp is the corresponding generalized least-pth 2 2 2 F = b 2xyb + : (6) power regression.
Details
-
File Typepdf
-
Upload Time-
-
Content LanguagesEnglish
-
Upload UserAnonymous/Not logged-in
-
File Pages10 Page
-
File Size-