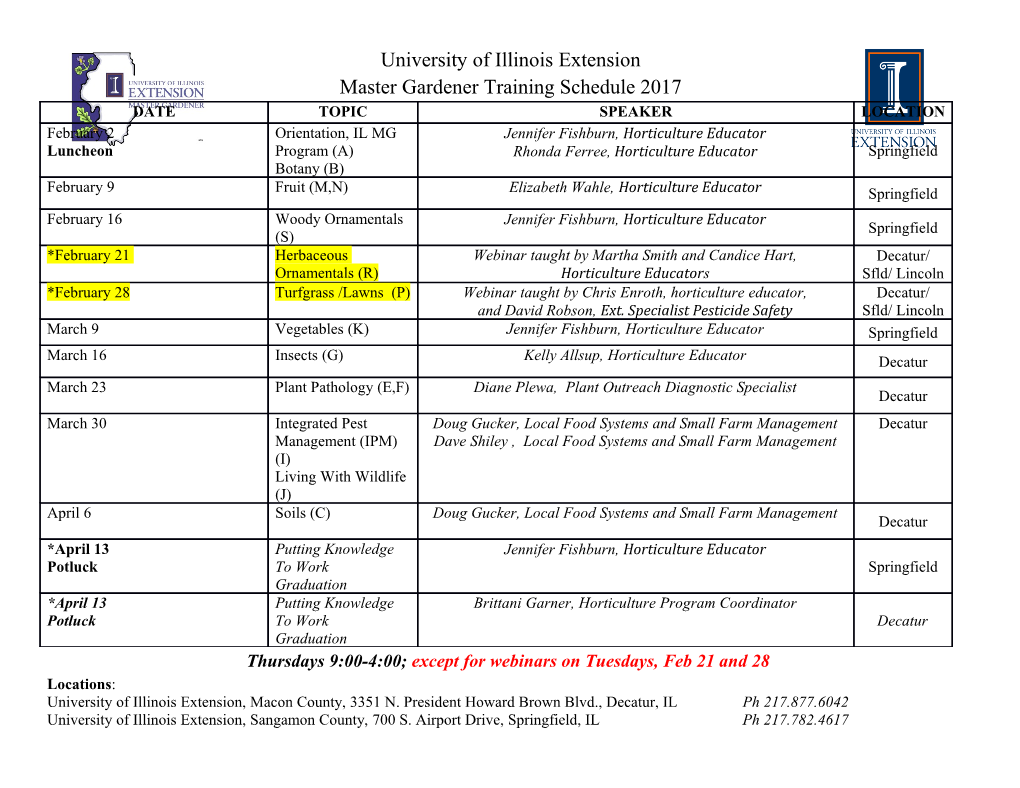
Systems-Based Approaches at the Frontiers of Chemical Engineering and Computational Biology: Advances and Challenges Christodoulos A. Floudas Princeton University Department of Chemical Engineering Program of Applied and Computational Mathematics Department of Operations Research and Financial Engineering Center for Quantitative Biology Outline • Theme: Scientific/Personal Journey • Research Philosophy at CASL (Computer Aided Systems Laboratory, Princeton University) • Research Areas: Advances & Challenges • Acknowledgements Greece Ioannina, Greece Ioannina,Greece (Courtesy of G. Floudas) Thessaloniki, Greece Aristotle University of Thessaloniki Department of Chemical Engineering Aristotle University of Thessaloniki Undergraduate Studies(1977-1982) Pittsburgh, PA Carnegie Mellon University Graduate Studies How did it all start? • Ph.D. Thesis: Sept. 82 – Dec. 85 •Advisor: Ignacio E. Grossmann • Synthesis of Flexible Heat Exchanger Networks (82-85) • Uncertainty Analysis (82-85) Princeton, NJ Princeton University Computer Aided Systems Laboratory Interface Product & Process Systems Engineering • Chemical Engineering • Applied Mathematics Research • Operations Research Areas • Computer Science • Computational Chemistry Computational Biology & Genomics • Computational Biology Unified Theory • address fundamental problems and applications via mathematical and Research modeling of microscopic, mesoscopic and macroscopic level Philosophy • rigorous optimization theory and algorithms • large scale computations in high performance clusters Mathematical Modeling, Optimization Theory & Algorithms Themes Discovery at the Macroscopic Level Discovery at the Microscopic Level Research Areas (1986 – present) Process Operations: Process and Product Scheduling, Planning and Design & Synthesis Uncertainty Optimization Theory & Methods: - Mixed-Integer Nonlinear Optimization - Deterministic Global Optimization Interaction of Design, Computational Biology & Synthesis & Control Genomics Process & Product Design and Synthesis (1986-) Christodoulos A. Floudas Princeton University Process & Product Design and Synthesis • Distillation Sequences (86-91) • Heat Exchanger Networks (86-91) • Reactor Networks and Reactor-Separator-Recycle (87-02) • Phase Equilibrium (94-02) • Azeotropic Separations (96-02) • Shape Selective Separation/Catalysis (05-) Rational Design of Shape Selective Separation and Catalysis C.E. Gounaris, C.A. Floudas, J. Wei Department of Chemical Engineering, Princeton University Fundamental Questions • Given a candidate set of zeolite portals and a pair of molecules (e.g., Molecule A and Molecule B), (a) can we identify the best zeolite portal which can separate molecule A from molecule B more effectively? (b) can we generate a rank-ordered list of zeolite portals for such separation? (c) can we identify such zeolite portals for any pair of molecules and the complete set of known zeolites? Molecular Footprints • Start with a simple molecular model: • Atoms are spheres of some effective radius • Bond lengths and angles considered fixed • Given this 3-d conformation, rotate suitably the molecule and project it onto the 2-d xy-plane • This projection is a set of circles • Circle centers are projections of atom nuclei • Circle radii are effective radii of atom spheres • Different 3-d orientations result into different projections • We define the molecular footprint to be such a projection that would likely be explored when penetration through a portal occurs Molecular Footprints - Examples • Aromatics : (a) benzene (b) toluene (c) o-xylene (d) m-xylene (e) p-xylene • Benzene is a planar molecule and results into a linear projection • The other aromatics have “almost” linear footprints Strain-based Screening • When a guest molecule approaches a host portal : No passage - There is no orientation for which all projected atom nuclei fall inside the portal area Constrained passage - There is some orientation for which all projected atom nuclei fall inside the portal area, but some circles have to be squeezed for a complete fit There is some orientation for which all Free passage - circles fall completely inside the portal area Strain-based Screening - Model • Let us define : (squeezed radius) rs Amount of distortion on an atom : δ = (original radius) ro ⎛⎞ Total Strain for a guest molecule ⎛⎞11 11 SS=+=GH S ⎜⎟ − +⎜⎟ − ∑∑δ 12δδδ 6⎜⎟ 12 6 to penetrate through a host portal : ij⎝⎠ii⎝⎠ jj • Every projection is associated with some total strain and there is an optimal projection that exhibits the minimum strain, denoted as S* • Define Strain Index : SI=+log(1 S * ) • SI is a measure of total distortion needed for penetration SI = 0 0 < SI < ∞ SI --> ∞ Strain-based Screening - Results • 38 molecules / 217 zeolite windows Strain Index Strain-based Screening - Results • 38 molecules / 217 zeolite windows Detail: Strain Index of aromatics on some 12-oxygen ring windows Interaction of Design & Control (1987-2000) Christodoulos A. Floudas Princeton University Interaction of Design & Control • Structural Properties: Generic Rank • Dynamic Operability of MIMO Systems: Time Delays & Transmission Zeroes • Multi-objective Framework for the Interaction of Design and Control • Optimal Control of Reactors • Dynamic Models in the Interaction of Design and Control Deterministic Global Optimization (1987-) Christodoulos A. Floudas Princeton University Deterministic Global Optimization How/When did we start? Motivation: Multiple local minima in - distillation sequencing - heat exchanger networks - reactor network synthesis - pooling problems Initial Studies: 1987-89 Historical Global Optimization Perspective 5496 2397 # Publications 1046 97 25 33 1960-1979 1980-1984 1985-1989 1990-1994 1995-1999 2000-2006 ChE Global Optimization Perspective: Early Contributions Floudas, Agarwal, Ciric (1989) Stephanopoulos & Westerberg (1975) Floudas & Visweswaran (1990) Westerberg & Shah (1978) Wang & Luus (1978) ChE Publications 1975 1980 1985 1990 1995 2000 2005 Deterministic Global Optimization • GOS: Global Optimum Search (88-89) • GOP: Biconvex, Bilinear, Polynomial (90-96) • aBB: Twice Continuously Differentiable Constrained Nonlinear Problems (94-98) • SMIN-aBB, GMIN-aBB: Mixed-Integer Nonlinear (98-00) • DAE: Differential-Algebraic Systems (98-02) • Bilevel Nonlinear Problems (01-05) • Convex Envelopes for Trilinear Monomials (03-04) • Convex Underestimators for Trigonometric Functions (04-05) • G-aBB: Generalized aBB (04-) • P-aBB: Piecewise aBB (05-) • Tight Convex Underestimators for General C2 Functions (06-) Deterministic Global Optimization: Chemical Engineering Applications • Phase & Chemical Reaction Equilibrium • Homogeneous, Heterogeneous & Reactive Azeotropes • Pooling Problems • Parameter Estimation & Data Reconciliation • Trim Loss Minimization • Generalized Pooling Problems • Nesting of Arbitrary Shapes Generalized Pooling Problem Meyer, Floudas (2006) Sources Plants Destinations Q1: What is the optimal topology? Binary Terms Q2: Which plants exist? Binary Variables Formulation of Generalized Pooling Problem Objective: Minimize Overall Cost - Plant construction and operating costs - Pipeline construction and operating cost Binary Variables a -y s,e: Existence of stream connecting source s to exit stream e. b - y t,e: Existence of stream connecting plant t to exit stream e. c - y t,t’: Existence of directed stream connecting plant t to plant t’. d - y s,t: Existence of stream connecting source s to plant t. e - y t: Existence of plant t. Problem Characteristics - Mixed integer bilinear programming problem with bilinearities involving pairs of continuous variables, (b,f) and (c,f) and (d,f). - Nonconvex mass balance constraints on the species include bilinear terms. - Industrial case study: |C| = 3, |E| = 1, |S| = 7, |T| = 10. - Number of nonconvex equality constraints: |C| x (|T| + |E|). (33) - Number of bilinear terms: |C| x |T| x (|E| + |S| + 2|T| - 2). (780) - Complex network structure with numerous feasible yet nonoptimal possibilities. - Number of binary variables: |T| x (|E| + |S| + |T|) + |S| x |E|. (187) - Fixing the y variables, the problem is a nonconvex bilinear NLP. Feasible Solutions Objective function value: 1.086e6 Objective function value: 1.198e6 S1 S1 S2 S2 T3 T3 S3 S3 T7 T7 S4 E1 S4 E1 T9 T9 S5 S5 T10 T10 S6 S6 S7 S7 Objective function value: 1.132e6 Objective function value: 1.620e6 S1 S1 S2 S2 T2 T11 S3 S3 T3 T33 S4 E1 S4 E1 T7 T7 S5 S5 T9 T9 S6 S6 S7 S7 Industrial Case Study Components: 3 Best known solution: 1.086 x 106 Sources: 7 Lower bound on solution: 1.070 x 106 Exit streams: 1 Absolute Gap: 0.016 x 106 Potential plants: 10 Relative Gap: 1.5 % Formulation ℜ var. {0,1} var Constr. CPU (s) Obj (106) Nonconvex 207 187 424 2.5 1.086 Bilinear Terms 987 187 3544 58 0.550 RLT 3850 187 19321 3621 0.743 Subnetwork {t3, t7, t9, t10} Bin RLT N = 2 766 79 4866 519 0.977 Bin RLT N = 3 766 91 6234 816 1.005 Bin RLT N = 4 766 103 7602 3672 1.022 Bin RLT N = 5 766 115 8970 7617 1.031 Bin RLT N = 6 766 127 10338 85800 1.051 Bin RLT N = 7 766 139 11706 59486 1.070 Challenges/Opportunities Current Status: Great Success for Theory & Algorithm for Small to Medium-size Applications • Improved Convex Underestimation Methods • Now Theoretical Results on Convex Envelopes • Medium to Large-scale C2-NLPs – Pooling Problems • Medium to Large-scale MINLPs – Product & Process Design/Synthesis/Operations – Signal Transduction/Metabolic Pathways – Generalized Pooling Problems • New Theory and Algorithms for DAE Models • New Theory and Algorithms for Grey/Black
Details
-
File Typepdf
-
Upload Time-
-
Content LanguagesEnglish
-
Upload UserAnonymous/Not logged-in
-
File Pages88 Page
-
File Size-