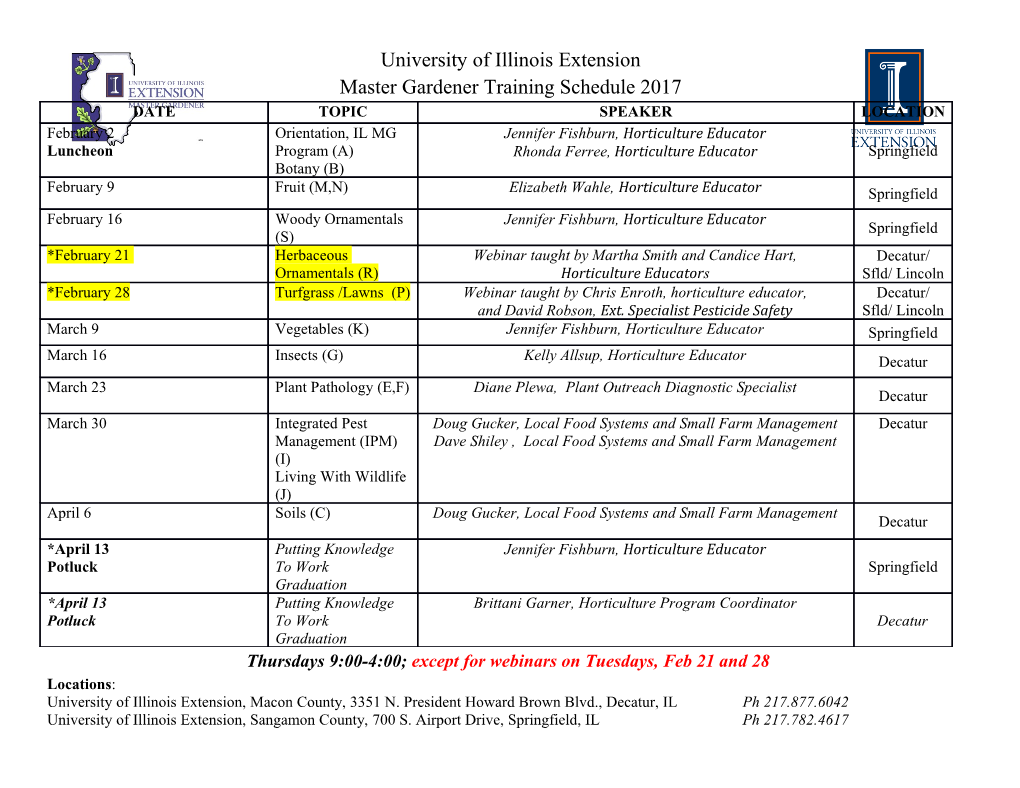
STATISTICAL METHODS FOR INTEGRATING DISPARATE DATA SOURCES by Prosenjit Kundu A dissertation submitted to Johns Hopkins University in conformity with the requirements for the degree of Doctor of Philosophy Baltimore, Maryland April, 2020 Copyright 2020 by Prosenjit Kundu All rights reserved Abstract My thesis is about developing statistical methods by integrating disparate data sources with real data applications, and identifying gene-environment interac- tions (G E) in more extensive studies using existing analytical methods. We × propose a general and novel statistical framework for combining information on multivariate regression parameters across multiple different studies which have varying level of covariate information (Chapter 2). We illustrate the method us- ing real data for developing a breast cancer risk prediction model. We propose a generalized method of moments (GMM) approach for analyzing two-phase studies where we take into account the dependent structure of the datasets across the two-phases (Chapter 3). We illustrate the method using real data on Wilm’s tumor, a common type of kidney cancer in children. We analyze the largest gene by smoking interaction study for pancreatic ductal adenocarcinoma risk conducted to date using existing statistical methods (Chapter 4). Primary Readers Nilanjan Chatterjee (Advisor) Professor Department of Biostatistics & Department of Oncology ii Bloomberg School of Public Health, School of Medicine, The Johns Hop- kins University Alison Patricia Klein Professor Department of Oncology School of Medicine, The Johns Hopkins University Mei-Cheng Wang Professor Department of Biostatistics Bloomberg School of Public Health, The Johns Hopkins University Debashree Ray Assisstant Professor Department of Epidemiology Bloomberg School of Public Health, The Johns Hopkins University Alternate Readers Elizabeth L. Ogburn Assisstant Professor Department of Biostatistics Bloomberg School of Public Health, The Johns Hopkins University Xiaobin Wang Professor Department of Population, Family and Reproductive Health Bloomberg School of Public Health, The Johns Hopkins University iii Acknowledgments As this long voyage of my PhD comes to an end, I want to take this moment to express my sincere gratitude to all the people who brought me to this significant stage of my life. I want to thank my Ph.D. advisor, Dr. Nilanjan Chatterjee, for supporting me during the past four years. He is a great teacher and an incredible mentor. One of the greatest things about Nilanjan-Da is the way he thinks. I remember many instances where he would explain the intuition behind a problem in a simple way that left me awestruck. I am genuinely grateful to him for having me injected with the ability to think about a problem intuitively before jumping into the mathematical details. I am thankful to him for helping me enrich my knowledge skills during our regular meetings. I also value his mentorship above and beyond academia, guiding and motivating me during difficult times. Besides my thesis advisor, I would like to thank Dr. Alison P. Klein, Dr. Mei-Cheng Wang, and Dr. Debashree Ray, for serving on my thesis committee. I want to pay my special regards to Alison for introducing me to the exciting research area in pancreatic cancer and guiding me with the fourth chapter of my thesis. I am grateful to Dr. Elizabeth L. Ogburn and Dr. Xiaobin Wang for serving as alternate members of my thesis committee. iv I remain eternally grateful to our department chair, Dr. Karen Bandeen- Roche, for all her support and advice during the past years. I want to take this opportunity to thank Dr. Bimal Kumar Roy (Indian Statistical Institute, Kolkata), Dr. Ciprian Crainiceanu, and Dr. Karen Banden Roche, who first provided me the opportunity to work (as an intern) in the Department of Bio- statistics, JHSPH, back in 2013. I am extremely grateful to them. My special thanks to Ciprian and the SMART group for having me introduced to the ex- citing and emerging area of wearable computing. The departmental admissions, administration & finance, information sys- tems were an integral part of my PhD journey. I want to acknowledge all of them, and especially, I would like to thank Mary Joy Argo, for sharing her ex- pertise and solving all my academic issues; Maria Beason, for assisting me in scheduling educational meetings; Debbie Cooper, Saima Ahmed, Fallon Bach- man, Debra Moffitt, Patty Hubbard for helping me with administrative concerns whenever needed, and Jiong Yang, for assisting me with any cluster-specific is- sues. A huge thanks to all. I had the excellent opportunity to work with some great collaborators: Dr. Runlong Tang, Dr. Allison Meisner, Dr. Evelina Mocci, Panc4, and PanScan members, Dr. Raymond J. Carroll, Dr. Joe Coresh, Natalie Daya, Dr. Liz Selvin, Brian Mcgrath, Statistical Genetics group and I am genuinely thankful for all their insights and fruitful discussions. I am also grateful to PCORI and NIH for their financial support. The charming city, Baltimore, will forever reamain close to my heart. In the past few years, Baltimore has become my hometown and I am lucky to have made amazing friends here. Thank you all for supporting me throughout this v entire process and helped me in maintaining a work-life balance. I want to start by thanking Kayode Sosina for being an awesome officemate and for being just a phone call away for intense research discussions. Special thanks to Parichoy Pal Choudhury, Sumit Sahu, and Shatabdi Pal for making me feel home away from home. They were my guardians, who kept me safe from grief and failures. A heartfelt thank you to Kunal Kundu for all his visits during my stay in Baltimore and for cheering me up at critical times. Thanks to Debangan Dey, Sayan Ghoshal, Soumya Banerjee, Anindya Bhaduri, Arunima Banerjee, and Neha Agarwala. They have become my family now. Thank you for being a part and celebrating the exciting moments of my life with me, be it hanging-out on weekends, studying in the library, planning sudden trips, eating at buffets, and sometimes gambling. My PhD journey has become an easy ride for these people. Some other friends who deserve special mention are Lacey Etzkorn, Linda Gai, Batel Blechter, Haoyu Zhang, Dora Zhang, Subhra Shankar Koley, Amartya Bhattacharjee, Ipshita Bhattacharya, Tushita Mukhopadhyay, Sayantan Dutta, Roshni Roy, Diptavo Dutta among others. To my long list of friends located around the world: Lokaditya Ryali, Avijit Singh, Arnab Das, Debarya Dutta, Prakash Chakraborty, Sandipan Chattopadhyay, Indrayudh Ghoshal, Sourav Sarkar (chutku), Indranil Bhattacharya and others, thank you for all your visits, texts and phone calls. I want to thank Neha Agarwala, one of the most important persons of my life. Thank you for being there with me and making this journey worthwhile. Thank you for all the beautiful memories, for inspiring me and being a wonderful friend. I can’t thank enough my family: Maa (Soma Kundu), Baba (Debdas Kundu), vi Dada (Ankit Kundu), and Boudi (Shrutilekha Roy), for all their endless, un- paralleled love and support. I couldn’t have asked for a better childhood friend than my dada. Maa and baba have always sacrificed their wishes to fulfill my dreams. They took the best care of me and provided the best academic envi- ronment at home. Whatever I am today is because of my family. Thank you, baba, maa, dada , and boudi. vii Dedications Dedicated to Maa, Baba, Dada, Boudi, Neha, and friends for all your love and inspiration viii Table of Contents Table of Contents vi List of Tables x List of Figures xii 1 Introduction 1 2 Generalized Meta-Analysis 8 2.1 Introduction . 8 2.2 Models and Methods . 10 2.2.1 Formulation of the model . 10 2.2.2 A Special Case Involving Linear Regression Model . 11 2.2.3 Generalized meta-analysis . 13 2.2.4 Iterated Reweighted Least Square Algorithm . 18 2.2.5 Diagnostic Test for Model Violation . 21 2.3 Simulations . 22 2.3.1 Homogeneous Population . 23 2.3.2 Heterogeneous Population . 28 ix 2.3.3 Power Evaluation of the Diagnostic Test (TGENMET A) . 29 2.4 Real Data Analysis . 32 2.5 Discussion . 39 3 Analysis of Two-Phase Studies using Generalized Method of Moments 43 3.1 Introduction . 43 3.1.1 Model Formulation . 46 3.1.2 Sampling Design . 46 3.1.3 Method . 47 3.2 Simulation Studies Resembling US National Wilms Tumor Data 49 3.3 Application to US National Wilms Tumor Data . 53 3.4 Discussion . 55 4 Genome-wide Interaction Scan Identifies Gene by Smoking in- teraction at 2q21.3 for Pancreatic Cancer Risk 61 4.1 Introduction . 61 4.2 Results . 64 4.2.1 Established GWAS regions . 67 4.3 Methods . 70 4.3.1 Study sample . 70 4.3.2 Genome-wide association genotyping data . 71 4.3.3 Smoking and demographic assessment . 72 4.3.4 Statistical analyses . 72 4.4 Discussion . 75 x 5 Conclusions and Future Work 79 5.0.1 Contribution . 79 5.0.2 Future Work . 82 References 86 A Chapter 1 127 A.1 Asymptotic Equivalence of GENMETA Estimator and Simple Meta-Analysis Estimator When All the Reduced Models Are the Same to the Maximal Model . 127 A.2 Newton-Raphson’s Method and Iteratively Reweighted Least Squares Algorithm . 130 A.2.1 Case I : φ and φk’s are known. 131 A.2.2 Case II : φ and φk’s are unknown. 134 A.3 Full proof of theorem 2.2.1 and Checking regularity assumptions in two examples . 136 A.3.1 Regulartity Assumptions for Theorem 2.2.1 . 136 A.4 Simulation Results for Log-normally Distributed Covariates . 146 B Chapter 2 148 B.1 Estimating equation in phase-I . 148 B.2 Estimating equation in phase-II . 150 B.3 Consistency and Asymptotic Normality .
Details
-
File Typepdf
-
Upload Time-
-
Content LanguagesEnglish
-
Upload UserAnonymous/Not logged-in
-
File Pages180 Page
-
File Size-