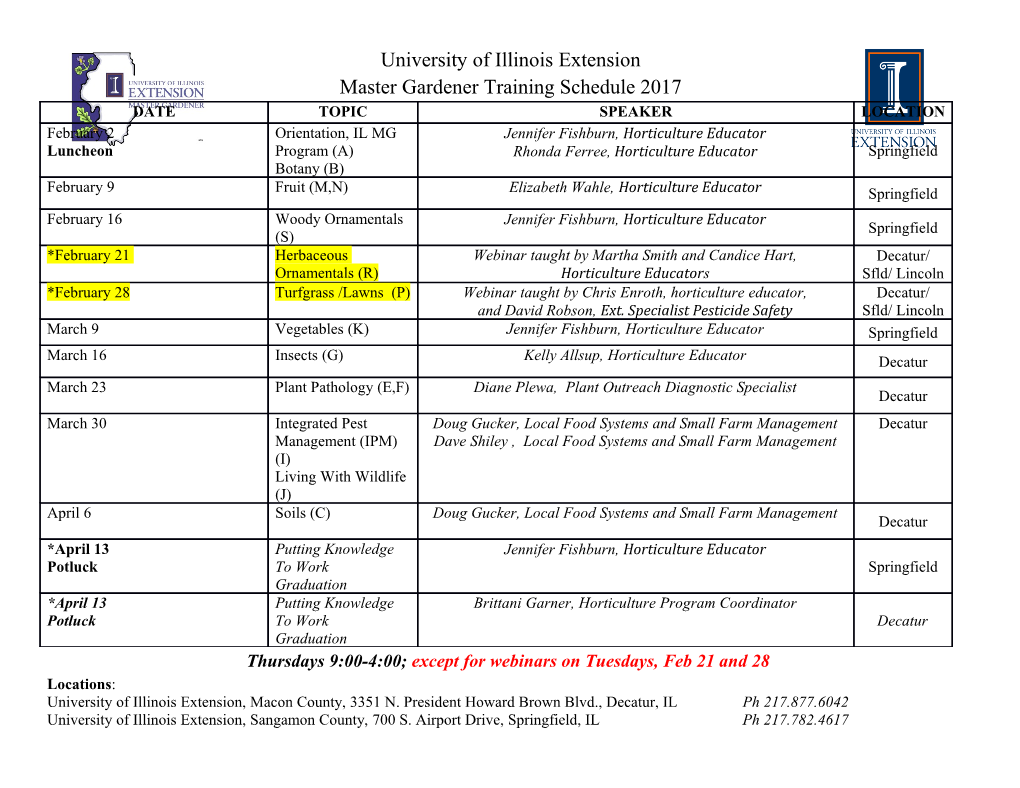
Notes on Malliavin Calculus Joe Jackson May 20, 2020 These are lecture notes for a summer 2020 mini course on Malliavin Calculus. First, we will review stochastic integration, and introduce the basic operators of Malliavin calculus. We will then take a detour to study some basic SDE theory, and see the connection between SDEs and the Cauchy problem. Finally, we will explain how Malliavin calculus can be applied to give a probabilistic proof of H¨ormander's Theorem. Sections 2 and 4 of the notes borrow heavily from the book Introduction to Malliavin Calculus by David Nualart, and much of Section 5 on H¨ormander'sTheorem I learned from an expository paper by Martin Hairer, titled On Malliavin's Proof of H¨ormander'sTheorem. Contents 1 Probabilistic Setup 2 1.1 Wiener Space . .2 1.2 Definite It^oIntegral . .2 1.3 Indefinite It^oIntegral . .4 1.4 Stratonovich Integral . .4 2 The Malliavin Derivative and its Adjoint 5 2.1 Definitions . .5 2.2 Interpreting D and δ ...............................7 2.3 Some Tools . .8 3 Stochastic Differential Equations 10 3.1 Existence and Uniqueness . 11 3.2 The Derivative of an SDE Solution . 13 3.3 Cauchy Problem and SDEs . 14 4 Malliavin Calculus and Densities 15 4.1 Some Results in 1-D................................ 15 4.2 Main Criteria for the Existence of Smooth Densities . 17 5 H¨ormander'sTheorem 17 5.1 Statement of the Theorem . 18 5.2 Intuition about H¨ormander'sCondition . 19 1 1 Probabilistic Setup Let (Ω; F; P) be a probability space, and X :Ω ! R a random variable. We would like to talk about the \derivative" of X, but this is hopeless without some more analytical structure on Ω. Luckily, many random variables of interest are defined as functionals of some Brownian motion, in which case we might as well take (Ω; F; P) to be the Wiener space. 1.1 Wiener Space Definition 1.1. The Wiener space is the probability space (Ω; F; P) where Ω = C(R+; R), F is the Borel σ-algebra there, and P is the unique measure such that the process Bt(!) = !(t) is a Brownian Motion. The Wiener space also comes with a natural choice of filtration, namely the augmentation of the natural filtration of B. More precisely, we take F = (Ft)t≥0, where Ft = σ(fBs : s ≤ tg _ fA 2 F : P[A] = 0g): (1) For the rest of these notes, (Ω; F; P) will be the Wiener space, B :Ω × R+ will be the Brownian motion Bt(!) = !(t), and F will be the filtration defined in (1). Exercise 1.2. Let H denote the set of random variables of the form F (!) = f(!(t1); :::; !(tn)) = f(Bt1 (!); :::; Btn (!) n for some 0 ≤ t1 < ::: < tn < 1 and f : R ! R bounded and measurable. Show that H is dense in L2(Ω; F). 1.2 Definite It^oIntegral Now we define the integral of a process with respect to B. Definition 1.3. A process X :Ω × R+ ! R is called simple if it takes the form X = P 2 i Hi1(ti;ti+1](t) for a some 0 = t0 < t1 < ::: < tn < 1 and Hi 2 L (Ω; Fti ). The integral of a simple process is easy to define, and analogous to the Riemann integral of a step function. P R 1 : P Definition 1.4. If X = i Hi1(ti;ti+1](t) is simple, then 0 XtdBt = i Hi(Bti+1 − Bti ) 2 L2(Ω) is the It^ointegral of X with respect to B. R 1 2 We now have a well-defined map X 7! 0 XtdBt from simple processes into L (Ω) = L2(Ω; F). In fact, this map is an isometry. Proposition 1.5. If X and Y are simple processes, then Z 1 Z 1 2 2 h XtdBt; YtdBtiL (Ω) = hX; Y iL (Ω×R+) 0 0 2 Exercise 1.6. Prove Proposition 1.5. 2 Now we want to identify the closure of the set of simple processes in L (Ω × R+). Definition 1.7. A process X :Ω×R+ ! R is progressively measurable (or progressive) if for all t, XjΩ×[0;t] is measurable with respect to the σ-algebra Ft ⊗B([0; t]). The progressive σ-algebra, denoted P, is the σ-algebra on Ω × R+ generated by all progressive processes. Exercise 1.8. Show that P = σ(fA 2 F ⊗ B([0;T ]) : A \ (Ω × [0; t]) 2 Ft ⊗ B([0; t]) 8 tg): 2 2 We will use L (P) to denote the space L (Ω × R+; P), and view it in the natural way as 2 2 a closed subspace of L (Ω × R+) = L (Ω × R+; F ⊗ B(R+)). Then we have the following: 2 2 Proposition 1.9. The closure of the space of simple process in L (Ω × R+) is L (P). Exercise 1.10. The proof of Proposition 1.9 takes some work, but one inclusion is easy. Which one is it, and why? In light of Propositions 1.5 and 1.9, the It^ointegral on simple processes extends uniquely R 1 2 to an isometry X 7! 0 XsdBs on L (P), which we will also call the It^ointegral. More precisely, we have: Definition 1.11. For X 2 L2(P), the It^ointegral of X with respect to B is given R 1 R 1 n 2 n by 0 XsdBs := limn!1 0 Xs dBs, where the limit is taken in L (Ω), and fX g is any 2 sequence of simple process approaching X in L (Ω × R+). R T R 1 For T < 1 we define 0 XtdBt := 0 Xt1[0;t]dBt. R t R t Example 1.12. We can prove directly from the definitions that 0 sdBs = tBt − 0 Bsds. ∆ Indeed, for a partition ∆ = (t0; :::; tn) with 0 = t0 < ::: < tn = t, let h (t) be the step function ∆ X h = ti1[ti;ti+1): i ∆ 2 R t Then h (s) ! s in L ([0; t]) as jj∆jj ! 0, so by the definition of the It^ointegral, 0 sdBs is the L2 limit of the random variables Z t X h(s)dBs = ti(Bti+1 − Bti ) 0 i as the mesh of ∆ tends to zero. We have X X X X X ti(Bti+1 − Bti ) = tiBti+1 − ti+1Bti+1 + ti+1Bti+1 − tiBti i i i i i X = tBt − Bti+1 (ti+1 − ti): i P R t 2 Thus the proof is complete if we can show that Bt (ti+1 −ti) ! Bsds in L , as jj∆jj ! i i+1 0 P 0 but this follows from the dominated convergence theorem, because clearly i Bti+1 (ti+1 − R t 2 ti) ! 0 Bsds almost surely, and the sequence is dominated by t sup0≤s≤t jBsj 2 L . 3 1.3 Indefinite It^oIntegral Let M2 be the be the space of continuous, square integrable martingales M = (Mt)t≥0 such 2 that supt E[jMtj ] < 1. The martingale convergence theorem shows that if M 2 M2, then 2 2 there exists M1 2 L (Ω; F1) such that Mt ! M1 a.s. and in L . Furthermore, Doob's maximal inequality shows that M2 is a Hilbert space under the inner product hM; NiM2 = E[M1N1]. We will now use this Hilbert space structure to define the indefinite It^ointegral. P Definition 1.13. If X = i Gi1(ti;ti+1] is a simple process, then the indefinite integral of X with respect to B is the process Z t X (t; !) 7! XsdBs(!) := Gi(!)(Bti+1^t(!) − Bti^t(!)): 0 i R We will often denote the indefinite integral by XsdBs. Just as with the definite integral, we have an isometry property: R Proposition 1.14. If X simple, then XsdBs is a continuous square integrable martingale. For X; Y simple, Z Z 2 2 h XdB; Y dBiM = hX; Y iL (Ω×R+): R 2 As in the definite case, this allows us to define an isometry X 7! XdBs from L (P) to M2. 2 R R n Definition 1.15. For X 2 L (P), XdB = limn!1 X dB, where the limit is taken in n 2 M2 and X is any sequence of simple processes approaching X in L (Ω × R+). Exercise 1.16. Review Doob's Lp maximal inequality (if you need to), and use it to give a proof that M2 is complete. 2 We define H := L (R+). If we restrict to deterministic integrands, the definite It^ointegral 2 R 1 gives an isometry H ! L (Ω), h 7! B(h) := 0 h(t)dBt. In fact, the image of the map B : H ! L2(Ω) contains only Gaussian random variables. Proposition 1.17. If h 2 H, the continuous martingale R hdB is a Gaussian process; R t1 R tn that is, for any 0 ≤ t1; :::; tn ≤ 1, the random vector ( 0 h(t)dBt; :::; 0 h(t)dBt) is a multivariate Gaussian. In particular, B(h) is a Gaussian random variable. The map B can be viewed as a special case of something called Gaussian white noise, and is a basic building block of Malliavin calculus. 1.4 Stratonovich Integral For most of these notes we will use the It^ointegral, but it will be helpful when stating H¨ormander'stheorem to also have the Stratonovich formulation of SDEs at our disposal. 4 This section is a very short and very informal introduction to the Stratonovich integral.
Details
-
File Typepdf
-
Upload Time-
-
Content LanguagesEnglish
-
Upload UserAnonymous/Not logged-in
-
File Pages20 Page
-
File Size-