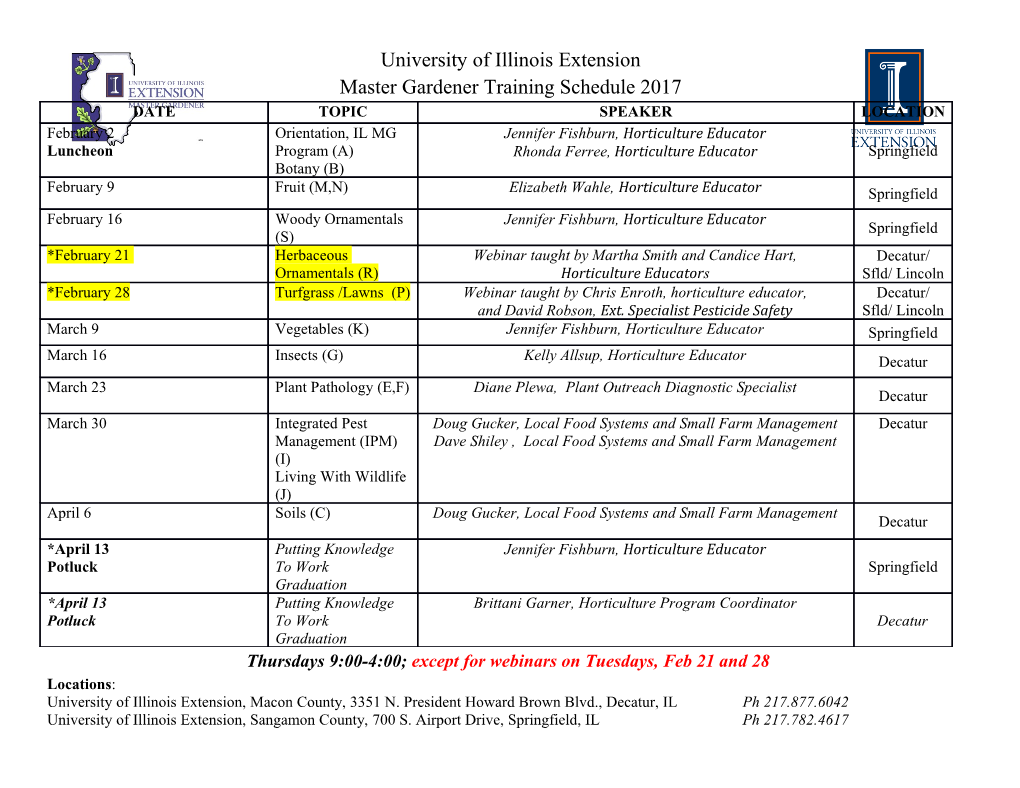
P1D.7 Interpreting Dropsonde Measurements of Turbulence in the Tropical Cyclone Boundary Layer Jeffrey D. Kepert ∗ Centre for Australian Weather and Climate Research A partnership between the Australian Bureau of Meteorology and CSIRO AMS Hurricanes Conference, Apr 28 - May 2, 2008 1. Introduction Dropsonde 981820069 90 Some of the most dangerous winds on earth occur in tropical cyclones. Their de- ) structive power depends as much on their −1 80 gustiness, or turbulence, as on their mean strength. Further, the efficiency with which 70 energy is extracted from the ocean to power the tropical cyclone depends strongly on 60 the boundary layer turbulence. Thus un- derstanding turbulence in the tropical cy- 50 clone boundary layer is crucial. At one Azimuthal velocity (m s level, this understanding should be a simple 40 problem because stability effects become −50 −40 −30 −20 −10 0 10 20 small as the wind speed increases. At an- Radial velocity (m s−1) other, the problem is complex because the strong winds severely modify the air-sea in- Fig. 1. Measured wind hodograph from a terface. Finally, testing theoretical predic- dropsonde deployed into the eyewall of Hur- tions and extrapolations from lower wind ricane Georges on 19 September 1998. speeds is problematic due to the severe dif- ficulty in taking observations where it mat- ters, in close to the interface. layer (Franklin et al. 2003; Wroe and Barnes Over the last decade, U.S. hurricane re- 2003; Schneider and Barnes 2005; Kepert connaissance aircraft have deployed thou- 2006a,b; Montgomery et al. 2006; Bell and sands of dropsondes in and near the eye- Montgomery 2008; Schwendike and Kepert wall of tropical cyclones. These instruments 2008), but the high sampling rate implies parachute towards the surface, reporting that there is a considerable potential to il- back wind, temperature, humidity and pres- luminate the turbulent structure as well. sure at ½-second intervals, or about every Figure 1 shows the hodograph of a sin- 6 m vertically (Hock and Franklin 1999). gle dropsonde deployed into the eyewall of These measurements have had a consider- Hurricane Georges (1998). It is clear that able impact on our knowledge of the mean the measured wind profile is consistent with structure of the tropical cyclone boundary many of the characteristics of turbulence: it is random, spatially correlated, contains ∗Centre for Australian Weather and Climate Re- search, Bureau of Meteorology, 700 Collins St, Mel- multiple scales, and is vortical. bourne Vic 3000. Email: [email protected] This paper presents a theoretical frame- 1 work for interpreting the turbulence signal Charnock’s relation (3) in combination with within these measurements. Proper inter- (1) and (2) is quite consistent with a linear pretation requires close attention to the re- increase of CD over the wind speed range of sponse characteristics of the instrument, interest. careful quality control, and a suitable theo- retical description of 3-dimensional surface b. Dropsonde dynamics layer turbulence. In this section, a theory is developed for Several useful results can be inferred from the mean and turbulent accelerations expe- the comparison of theory and measurement. rienced by a dropsonde as it falls through This paper will focus on one of these, the the atmospheric surface layer. The turbu- marine drag coefficient C . The main result D lent part of the theory is an extension of Kris- is that C does not increase indefinitely with D tensen (1993)’s analysis of cup anemome- wind speed, thus confirming recent direct ter dynamics to the case where the mean measurements (Powell et al. 2003; Black flow and the turbulence are not stationary et al. 2007; French et al. 2007) by a new in time. and independent technique, and extending The dropsonde is a first-order linear filter those results to much higher wind speeds. of the true wind (Hock and Franklin 1999): ˙ 2. Theory ˜(t) = s˜(t) + τ0s˜(t) (4) a. Air-sea momentum exchange where (,˜ ˜) is the instantaneous horizontal wind vector, (s˜, s˜y) is the horizontal compo- The surface stress τ may be written nent of the dropsonde velocity, τ0 = ƒ /g ≈ 1.2 s is a time scale, and ƒ is the dropsonde τ = ρC U2 = ρ2 (1) D 10 ∗ fall velocity. We adopt the usual surface- layer convention that the -axis is in the where ρ is the air density, U10 is the 10-m mean wind direction, y is to the left and z mean wind speed and CD the drag coeffi- is upwards. The solution of (4) is (Kristensen cient. Over the ocean, CD is thought to in- 1993) crease approx linearly with wind speed, as ∞ reflected in numerous empirical parameteri- 1 s˜ (t) = e−τ/τ0 ˜(t − τ)dτ (5) sations, for example Large and Pond (1981). τ0 Z0 The drag coefficient can be related to the We decompose all variables into mean and surface roughness length z0 through the log- arithmic wind profile, fluctuating components, ˜(t) = U(t) + (t), s˜(t) = S(t) + s(t), ∗ z U(z) = log (2) U(t) = S(t) + τ0S˙(t) (6) k z0 (t) = s(t) + τ0s˙(t) (7) with z = 10 m, where k is von Kärmän’s Here, the ergodic hypothesis is unavail- constant and the roughness length z over 0 able because the dropsondes are falling the ocean is frequently parameterised after through gradients of both mean and turbu- Charnock (1955) lence quantities, and so “mean” should be 2 interpreted as an ensemble-average follow- ∗ z0 = α . (3) ing the dropsonde trajectory. g In the case where the mean wind profile U(z) is logarithmic (2), an analytic solution Here α, the Charnock coefficient, is an em- is available for (6), pirical constant and g is the gravitational ac- celeration. The value α = 0.011 is widely ∗ t ( ) = ( ) − −t/τ0 used, see for example Fairall et al. (2003). S t U t e Ei , (8) k τ0 2 where Ei is the exponential integral func- With initial condition 〈s2(−∞)〉 = 0, the solu- tion and the dropsonde fall velocity has been tion is used for the space-time conversion, t = −ƒ z. 〈s2(t)〉 An expression for the variance of the drop- 2 ∞ sonde acceleration is found by multiplying −2τ/τ0 = e 〈(t − τ)s(t − τ)〉dτ (16) (7) in turn by , s and s˙, ensemble- τ0 Z0 averaging, and a little manipulation which can be evaluated from (13) by a nu- 2 2 〈 〉 − 2〈s〉 + 〈s 〉 merical integration. 〈s˙ 2〉 = (9) 2 Note that τ0 ∞ 4π2ƒ 2τ2 Angle brackets denote an ensemble average 2 0 〈 〉−〈s〉 = S(ƒ )dƒ = H at any fixed point in the dropsonde trajec- Z 2 2 2 −∞ 1 + 4π ƒ τ0 tory. We now proceed to evaluate the right- (17) hand side of (9). and Taking the fluctuating part of (5), multiply- ing by and ensemble-averaging, 2 ∞ 〈s2〉−〈s 〉 = H − H e−2τ/τ0 dτ (18) ∞ τ0 Z0 −τ/τ0 〈s〉 = R(−τ)e d(τ/τ0), (10) Z0 where H is a high-pass filtered wind veloc- ity variance. Thus, from (9) the dropsonde where 2 -acceleration variance 〈s˙ 〉 depends only R(−τ) = 〈(t)(t − τ)〉 (11) on the high-frequency part of the turbulence is the autocovariance of the along-stream spectrum, consistent with the fact that dif- wind component with lag −τ. We represent ferentiation is a high-pass filtering opera- R by its spectrum S, tion. ∞ Dropsondes do not measure a simultane- −2πƒ τ R(−τ) = S(ƒ )e dƒ (12) ous wind profile, but rather a time-series Z−∞ along a slant trajectory. Thus the above the- Thus the covariance between the - ory requires the autocorrelation of the time- component of the dropsonde and wind ve- series along the dropsonde slant trajectory: locities is R(−τ) ≡ R(−Uτ, 0, ƒ τ, −τ) ∞ 1 (19) = R(0, 0, ƒ τ, 0), 〈s〉 = S(ƒ )dƒ (13) Z 2 2 2 −∞ 1 + 4π ƒ τ0 where the equality with the instantaneous Note that the wind velocity variance can also vertical profile follows by Taylor’s hypothe- be written in terms of S by virtue of Parse- sis. val’s theorem, Thus the one-dimensional line spectrum in 3 ∞ the vertical direction, F (k ), is required. 11 3 2 〈 〉 = S(ƒ )dƒ (14) There seem to be no published forms for Z−∞ such spectra in the atmospheric surface layer, but they may be derived from the full Multiplying (7) by s and ensemble- averaging gives another equation for a first- spectral velocity tensor, theoretical forms of order linear filter, which were given by Kristensen et al. (1989) and Mann (1994). Here, we shall adopt the τ ∂〈s2〉 2 2 0 former. 〈s〉 = 〈s 〉 + τ0〈ss˙〉 = 〈s 〉 + , 2 ∂t The one-dimensional along-stream turbu- (15) lence line spectrum is defined as the Fourier 3 transform of the along-stream autocorrela- 0 10 tion 1 ∞ F1(k ) = e−k1r1 R1(r )dr (20) j 1 j 1 1 2π Z −2 −∞ 10 where the indices , j = 1, 2, 3 refer to the three Cartesian axes. The spectral tensor is n S the extension of this transformation to three −4 dimensions, 10 1 −k·r j(k) = Rj(r)e dr (21) 3 3 (2π) ZZZR −6 10 −4 −2 0 2 Line spectra in any direction may be ob- 10 10 10 10 n tained from j(k) by integrating out the components of k normal to the desired di- Fig. 2. Atmospheric surface-layer velocity rection. In particular, vertical line spectra line spectra, for the (blue), (red) and are obtained by (green) velocity components. The dashed ∞ ∞ curves are for line spectra in the along- F3(k ) = (k , k , k )dk dk (22) j 3 j 1 2 3 1 2 stream direction, and the solid curves are for Z−∞ Z−∞ the vertical.
Details
-
File Typepdf
-
Upload Time-
-
Content LanguagesEnglish
-
Upload UserAnonymous/Not logged-in
-
File Pages8 Page
-
File Size-