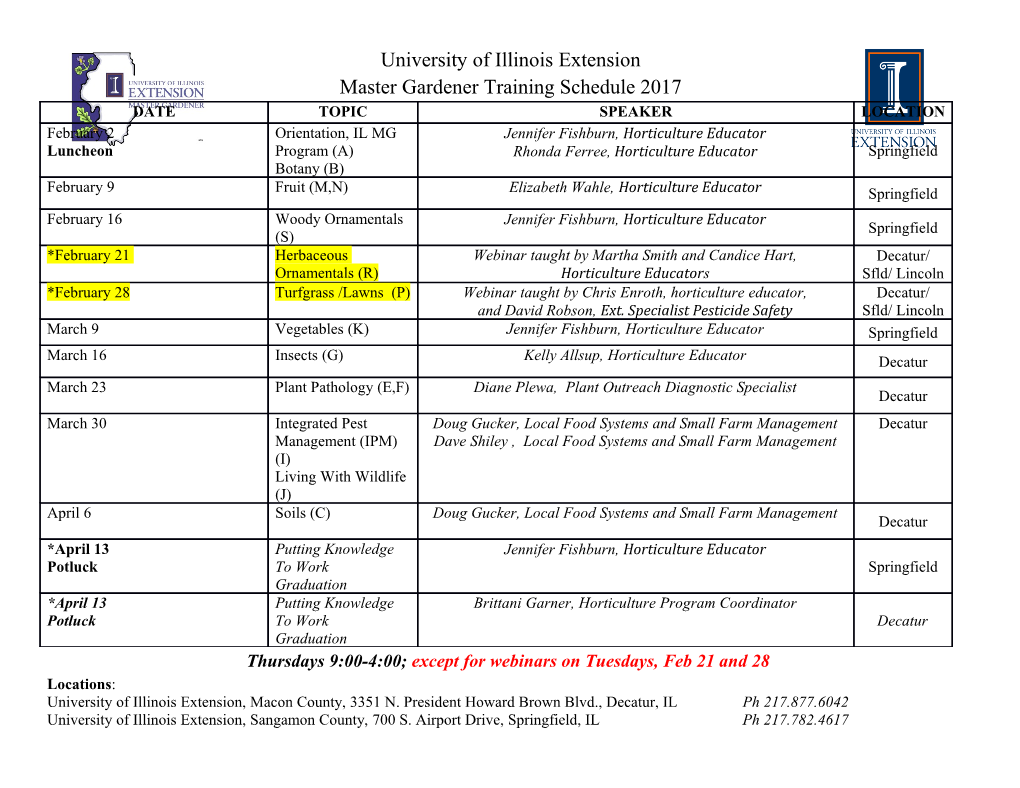
Chapter 9 Fourier Optics Part I Fourier Transform Fourier Series • Periodic function f(t) = f(t+T) for all t – T is the period – Period related to frequency by T = 1/푓0 = 2휋/휔0 – 0 is called the fundamental frequency • So we have f (t) a0 (an cos2nf0 bn sin 2nf0 ) n1 a0 (an cosn0 bn sin n0 ) n1 • n0 = 2n/T is nth harmonic of fundamental frequency Fourier Series • Calculation of Fourier coefficients hinges on orthogonally of sine, cosine functions T 0 sin(m0t)cos(n0t)dt 0, all m,n T T sin(m t)sin(n t)dt 0 0 0 2 mn T T cos(m t)cos(n t)dt 0 0 0 2 mn 1, when 푚 = 푛 where 훿 = is called Kronecker delta 푚푛 0, when 푚 ≠ 푛 Also T sin m0t dt 0, allm 0 T cosm0t dt 0, all m 0 Fourier Series • Based on above relationship, one can calculate the Fourier coefficients as 2 T a f (t)cos(n t) dt n T 0 0 2 T b f (t)sin(n t) dt n T 0 0 • Some rules simplify calculations – For even functions f(t) = f(-t), such as cos(t), bn terms = 0 – For odd functions f(t) = -f(-t), such as sin(t), an terms = 0 Fourier Series • Square wave 1, 0 t 1/ 2 f (t) 1,1/ 2 t 1 1 T/2 T -1 4 sin 30t sin 50t sin 70t f (t) sin 0t 3 5 7 Fourier Series • Square wave Gibbs phenomenon: ringing near discontinuity 1.5 1.0 n = 1 n = 2 0.5 n = 3 n = 4 ) t 0.0 ( f -0.5 -1.0 -1.5 0.0 0.5 1.0 1.5 2.0 t Fourier Series • Triangular wave +V T/2 T -V 4V t, 0 t T / 4 T 4V f (t) t 2V , T / 4 t 3T / 4 T 4V V t, 3T / 4 t T T 8V sin 30t sin 50t sin 70t f (t) 2 sin 0t 2 2 2 3 5 7 Fourier Series Parseval's theorem • If some function f(t) is represented by its Fourier expansion on an interval [-l, l], then 2 l 1 2a0 2 2 f(x)anbn l 2l 4n1 n1 • Useful in calculating power associated with waveform Fourier Series Exponential form of Fourier Series f (t) a0 an cosn0t bn sin n0t n1 Since 1 cosn t ein0t ein0t 0 2 1 sin n t ein0t ein0t 0 2 j Then ein0t ein0t ein0t ein0t f (t) a0 an bn n1 2 2i an ibn in0t an ibn in0t f (t) a0 e e n1 2 2 Fourier Series Introducing new coefficients a ib a ib c~ n n , c~ n n , c~ a n 2 n 2 0 0 We have ~ ~ in0t ~ in0t f (t) c0 cne cne n1 Or ~ in0t f (t) cne n The coefficients can easily be evaluated ~ an ibn 1 T i T c f (t)cosn t dt f (t)sin n t dt n 2 T 0 0 T 0 0 1 T 1 T in t f (t)cosn t isin n t dt f (t)e 0 dt T 0 0 0 T 0 Fourier Transform Fourier analysis for nonperiodic functions • Basic idea: extend previous method by letting T become infinite • Example: recurring pulse V0 t -a/2 a/2 T Fourier Transform Fourier analysis for nonperiodic functions The Fourier coefficients ~ 1 T in0t c f (t)e dt n T 0 1 a / 2 in t V e 0 dt T a / 2 0 Thus, V ein0a / 2 ein0a / 2 a sin(n a / 2) ~ 0 0 0 cn V0 n 2i 2 n0a / 2 Fourier Transform Fourier analysis for nonperiodic functions Since 푇 = 2휋/휔0, one has a sin(n a / 2) a sin(na /T ) ~ 0 cn V0 V0 T n0a / 2 T na /T We are interested in what happens as period T gets larger, with pulse width a fixed – For graphs, a = 1, V0 = 1 Fourier Transform Fourier analysis for nonperiodic functions 0.25 0.6 a/T a/T 0.5 0.2 0.4 0.15 0.3 0.1 0.2 0.05 0.1 0 coefficient value, T=5 coefficient value, T=2 0 0 50 100 150 0 50 100 150 -0.05 -0.1 -0.1 -0.2 frequency frequency 0.12 a/T 0.1 0.08 0.06 0.04 0.02 coefficient value, T=10 0 0 50 100 150 -0.02 -0.04 frequency Fourier Transform Fourier analysis for nonperiodic functions • We can define f(inw0) in the following manner ~ 1 a / 2 in0t c V e dt n T a / 2 0 ~ a / 2 in0t cnT a / 2V0e dt ~ ~ ~ cn cn F(in0 ) cnT 2 2 0 Since difference in frequency of terms ∆휔 = 휔0 in the expansion. Hence F(in ) c~ 0 n 2 Fourier Transform Fourier analysis for nonperiodic functions • Since ~ in0t f (t) cne n • It follows that F(in ) f (t) lim 0 ein0t T n 2 • As we pass to the limit, ∆휔 → 푑휔, 푛∆휔 → 휔 so we have 1 it f (t) F(i)e d 2 Fourier integral Fourier Transform • This is subject to convergence condition f (t) dt • Now observe that since ~ 1 T in0t c f (t)e dt n T 0 • We have ~ a / 2 in0t cnT F(in0 ) a / 2 f (t)e dt • In the limit as 푇 → ∞ a / 2 it it F(i) a / 2 f (t)e dt f (t)e dt Fourier Transform • Since f(t) = 0 for t < -a/2 and t > a/2 • Thus we have the Fourier transform pair for nonperiodic functions F(i) Ff (t) f (t) F -1 F(i) Fourier Transform • For pulse of area 1, height a, width 1/a, we have 1/ 2a it sin / 2a F() ae dt 1/ 2a / 2a • Note that this will have zeros at 휔 = 2푎푛휋, 푛 = 0, ±1, ±2, … • Considering only positive frequencies, and that “most” of the energy is in the first lobe, out to 2푎휋, we see that product of 1 bandwidth 2푎휋 and pulse width = 2휋 푎 a t -1/2a 1/2a T Fourier Transform 5 1.5 1.0 1 Width = 1 -1/2 1/2 Width = 0.2 0.5 -1/10 1/1 F 0 0.0 -0.5 -40 -20 0 20 40 Fourier Transform • Let 푎 → ∞ , but keep the same area, then 푓(푡) →spike of infinite height (delta function) – Transform → line F(i) =1 – Thus transform of delta function contains all frequencies sin / 2a F() lim 1 a / 2a So we get delta function, f(t) = (t) Fourier Transform Properties of delta function (x) 1 0 x 0 • Definition (x) x x 0 0 (x)dx 1 (x)dx • Area for any > 0 • Sifting property (x) f (x)dx f (0) (x- x0) (x x0 ) f (x)dx f (x0 ) 1 x 0 x0 since 0 x x0 (x x0 ) x x0 Fourier Transform Some common Fourier transform pairs F{1} 2 () F{ (x)} 1 f (x) F () f (x) F() 1 2 … x x 0 0 0 0 f (x) F () f (x) F() 1 1 … x x 0 0 0 0 Fourier Transform f (x) A (k) k x f (x) x d / 2 x d / 2 0 F{ f (x)} eid / 2 eid / 2 2cos(d / 2) f (x) B (k) f (x) x d / 2 x d / 2 id / 2 id / 2 x k F{ f (x)} e e 2isin(d / 2) 0 f (x) cos x 0 f (x) A (k) F{ f (x)} ( 0 ) ( 0 ) x f (x) sin0 x k 0 F{ f (x)} i ( 0 ) ( 0 ) f (x) B (k) x k 0 Fourier Transform Some common Fourier transform pairs function transform function transform Fourier Transform Properties of Fourier transforms • Simplification: F(i) 20 f (t)cost dt, f (t) even F(i) 20 f (t)sint dt, f (t) odd • Negative t: Ff (t) F *(i) • Scaling – Time: 1 i Ff (at) F a a – Magnitude: Faf (t) aF(i) Fourier Transform Properties of Fourier transforms • Shifting: Ff (t a) F(i)eia i0t Ff (t)e Fi( 0 ) 1 Ff (t)cos t Fi( ) Fi( ) 0 2 0 0 1 Ff (t)sin t Fi( ) Fi( ) 0 2 0 0 • Time convolution: 1 F F1(i)F2 (i) - f1( ) f2 (t )d • Frequency convolution: 1 F f (t) f (t) F (i)F i( )d 1 2 2 1 2 Linear System • A principal application of any transform theory comes from its application to linear systems – If system is linear, then its response to a sum of inputs is equal to the sum of its responses to the individual inputs • The response of something (e.g., a circuit) to a delta function is called its “impulse response” or called “point spread function” in optics, often denoted h(t) Linear System • The output signal is the convolution of the input signal and response +∞ 푌 푡 = −∞ 푥 휏 푓 푡 − 휏 푑휏 Or 푌 휔 = 푥 휔 푓(휔) Convolution Convolution and transforms (continued) • Example – Signal is square wave, u(t) = sgn(sin(x)) sin(2n 1) t u(t) 0 n1 2n1 – This has Fourier transform i (2n 1)0 (2n 1)0 U (i) 2 n1 2 2 – So response Y(i) is i (2n 1)0 (2n 1)0 Y (i) H (i)U (i) H (i) 2 n1 2 2 Part II Fourier Transform and Optics Diffraction Theory exp(ikr) E dxdy Fraunhofer diffraction A Aperture r R X 2 Y 2 Z 2 r X 2 (Y y)2 (Z z)2 R 1 (Yy Zz) / R2 exp(ikR) E(Y, Z) A expik(Yy Zz) / R dydz R Aperture E(Y , Z) A( y, z)expik(Yy Zz) / R dydz y Y kY kY / R Let , then P(Y,Z)Z kZ kZ / R dydz r R Y x i(kY ykZ z) E(kY ,kZ ) A( y, z)e dydz X z Z i(kY ykZ z) E(kY ,kZ ) A(y, z)e dydz Diffraction Theory Fraunhofer diffraction • Each image point corresponds to a spatial frequency.
Details
-
File Typepdf
-
Upload Time-
-
Content LanguagesEnglish
-
Upload UserAnonymous/Not logged-in
-
File Pages44 Page
-
File Size-