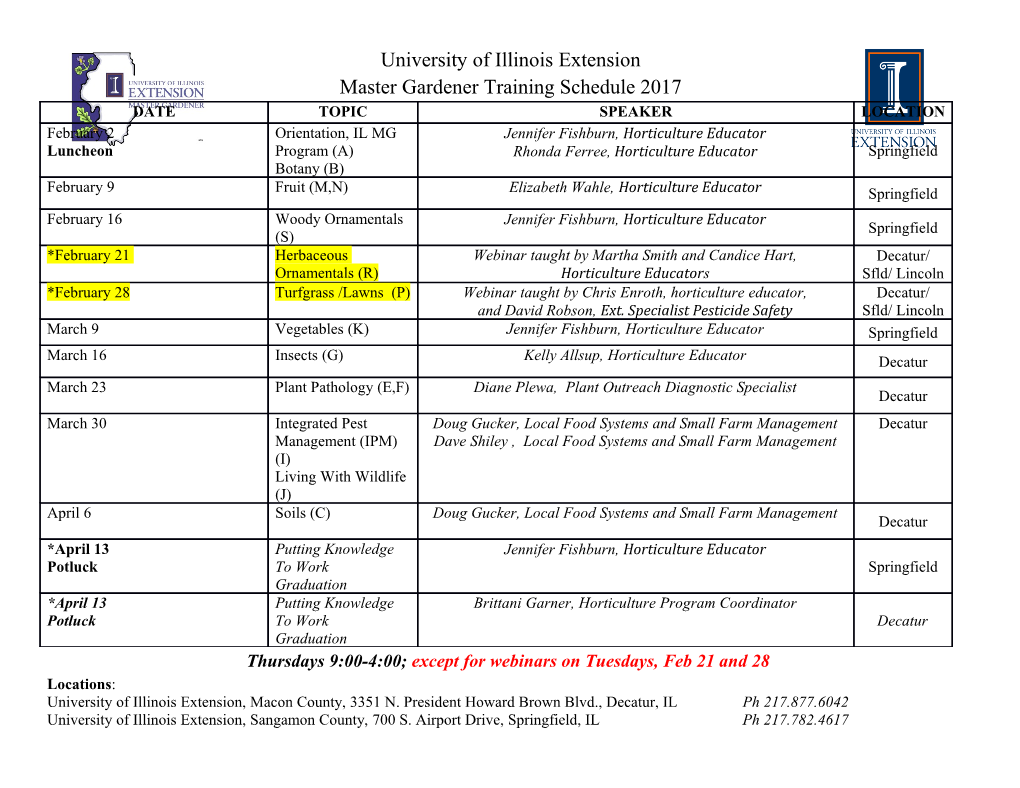
From: ISMB-93 Proceedings. Copyright © 1993, AAAI (www.aaai.org). All rights reserved. Representation for Discovery of Protein Motifs Darrell ConklinT, Suzanne Fortier~, Janice Glasgowt Departments~t of Computing and Information Sciencet and Chemistry Queen’s University Kingston, Ontario, Canada K7L 3N6 [email protected] Abstract pattern of amino acid residues. Protein motifs can be roughly classified into four categories. Sequence There are several dimensions and levels of com- motifs are linear strings of residues with an implicit plexity in which information on protein mo- topological ordering. Sequence-structure motifs are tifs may be available. For example, one- sequence motifs with secondary structure identifiers at- dimensional sequence motifs may be associated tached to one or more residues in the motif. Structure with secondary structure identifiers. Alterna- motifs are 3D structural objects, described by posi- tively, three-dimensional information on polypep- tions of residue objects in 3D Euclidian space. Apart tide segments may be used to induce prototypical from a topological linear ordering on the residues, three-dimensional structure templates. This pa- structure motifs are free of sequence information. Fi- per surveys various representations encountered nally, structure-sequence motifs are combined 1D- in the protein motif discovery literature. Many 3D structures that associate sequence information with of the representations are based on incompatible a structure motif. Figure 1 illustrates these four types semantics, making difficult the comparison and of protein motifs, along with somefurther subclassifi- combination of previous results. To make better cations which are elaborated upon later in the paper. use of machine learning techniques and to pro- The first three motif types are discussed by Thornton vide for an integrated knowledge representation and Gardner (1989); the structure-sequence motif will framework, a general representation language -- be presented in this paper. in which all types of motifs can be encoded and This paper has two objectives. The first is to provide given a uniform semantics -- is required. In this a survey of previous research on protein motif discov- paper we propose such a model, called a spatial ery according to the above categorization. The second description logic, and present a machine learning aim is to present a new approach to protein motif rep- approach based on the model. resentation and discovery, which is based on knowledge representation ideas of description logics and machine Introduction learning principles of structured concept formation. In the proposed representation language, sequence and A task of growing importance in the management of structure motifs have a uniform model-theoretic se- biochemical data is the ability to perform general- mantics. The processes of generalization and struc- ization and abstraction over large sets of related ob- tured concept formation are presented. The paper con- servations. Abstractions offer a form of conceptual cludes with a discussion of the role of protein motifs in aggregation, modelling, and explanation of observa- molecular scene analysis. tions, a method for practical data compression, and also serve to index large databases for efficient infor- mation retrieval. There exist recurrent patterns and Discovery of protein motifs rules of structural biochemistry hidden in the Protein Research in protein motif discovery generally falls into Data Bank (Bernstein et al., 1977); knowledge discov- the area of unsupervised machine learning; a machine ery techniques can help to uncover these patterns and is given a set of observations (i.e., a set of unclassified rules. Generalized patterns can facilitate information protein fragments) and must find a clustering of these retrieval and data incorporation, providing a concep- observations along with a description of each cluster tual framework in which new structural data can be (i.e., a motif describing the fragments in the cluster). related. They can also be used for prediction and an- A rigorous mathematical semantics for a motif is nec- ticipation of molecular conformation, since they often essary in order to determine whether an observation is represent common3D structural features. an instance of a motif. There has been a considerable A protein motif is an abstraction of some observed amount of research on machine discovery of protein Conklin 101 1) Sequence(conserved) G-X-G-X-X-G 5) Structure 2) Sequence(consensus) X-h-X-p-X-X-X 6) Structure-sequence (conserved) 3) Sequence-structure (conserved) G-X-G-X-X-G E-T-H-H-H-H 4) Sequence-structure(consensus) p-X-G 7) Structure-sequence (consensus) H-H-H s P ]X~x Figure 1: Various types of protein motifs. Legend; X : any residue, G : glycine, V : valine, H : helix, E : B-strand, T : turn, p : polar, s : small, h : hydrophobic. motifs. Below we describe and compare a handful of on the comparison of sequence motifs: see Lathrop methods in terms of their representation theory, the (Lathrop et al., 1993) for a good survey of this work. type of motif under consideration, and the semantics Sequence motifs are discovered from a maximal align- given to motifs. ment of one or more protein sequences, and the ab- straction of residues at aligned positions. Conserved Structure motifs residues are those identical at corresponding alignment Hunter and States (1991) apply Bayesian classification positions. It is uncommonto find long connected se- techniques to protein structure motif discovery. This quences of conserved residues in non-homologous pro- method produces clusterings which are evaluated ac- teins (Sternberg and Islam, 1990), hence the need for cording to Bayes’ formula with a prior distribution abstraction at alignment positions (e.g., abstracting favouring fewer classes; given two clusterings, each Ala and Phe to X) to produce a sequence motif. Taylor with the same number of classes, the method will prefer (1986) uses a more general abstraction scheme; amino the clustering which has a tighter fit to the data. Mo- acids are classified into nondisjoint groups based on tifs are represented by a probability distribution over physicochemical properties such as hydrophobicity and Cartesian coordinates for the backbone atoms of each polarity which axe expected to have an influence on residue. Thus motifs are probabilistic concepts; frag- protein folding. ments fall into a class with a certain probability. The PIMAmethod of Smith and Smith (1990) (also Roomanet al. (1990a) use an agglomerative numer- see (Lathrop et al., 1993)) discovers consensus ical clustering technique to discover structure motifs, quence motifs which cover an entire set of sequences which are represented by a pro~otypical fragment. In from functionally related proteins. A physicochemical contrast to the Hunter and States approach, only the classification of amino acids somewhatdifferent from Ca position is used as a descriptor for the position of Taylor’s (1986) is employed. Discovered patterns can the amino acid in Euclidian space. Thus amino acids contain special "gap" identifiers, which can function as are represented by point rather than line data. Simi- 0 or 1 residues. PIMA is a supervised learning method, laxity between fragments is measured by using an RMS since the class of related sequences (e.g., from the pro- metric of the inter-C~ distances. A fragment is an in- tein kinase family) is delimited prior to discovery of stance of a class by virtue of being within an RMS covering sequence motif. distance threshold from the prototypicai motif of that Much of the work on protein secondary structure class. prediction is based on the a priori definition of se- quence motifs that are predictive of a certain type of Sequence and sequence-structure motifs secondary structure identifier. Thornton and Gardner Protein sequence motifs are the most commonly en- (1989) refer to these motifs as "structure-related se- countered motif type in the molecular biology litera- quence motifs". For example, Roomanet al. (1989) ture. There is, for example, an extensive literature associate with each amino acid in a motif a standard 102 ISMB--93 secondary structure identifier (e.g., Figure 1, motif The sequences corresponding to this core for each 3). It is demonstrated that there exist associations training protein are then aligned. Conserved residues for which sequence templates reliably characterize sec- are retained and assigned to the commonstructure mo- ondary structure. Rooman et al. (1990b) report tiL A notable difference between this and other struc- study very similar to previous work (Roomanet ai., ture motif work is that the technique does not require a ¯ 1989), but instead replace the structure identifiers with training set of fragments of fixed size. Blundell’s group identifiers discovered by the previously discussed struc- is concerned with knowledge based protein modelling:. ture motif analysis (Roomanet al., 1990a). protein structure prediction, where the main source of Harris et al. (1992) use a sequence alignment pro- information comes from exploration and abstraction cedure to discover similarities and clusters of protein of knownstructures. In this spirit, Sali and Blundell sequence regions. Roughly 10,000 classes of variable- (1990) develop an elaborate scheme for the comparison length sequences were uncovered. Their method does of protein structures. The results of a comparison form not find a comprehensible motif description for discov- a "generalized
Details
-
File Typepdf
-
Upload Time-
-
Content LanguagesEnglish
-
Upload UserAnonymous/Not logged-in
-
File Pages8 Page
-
File Size-