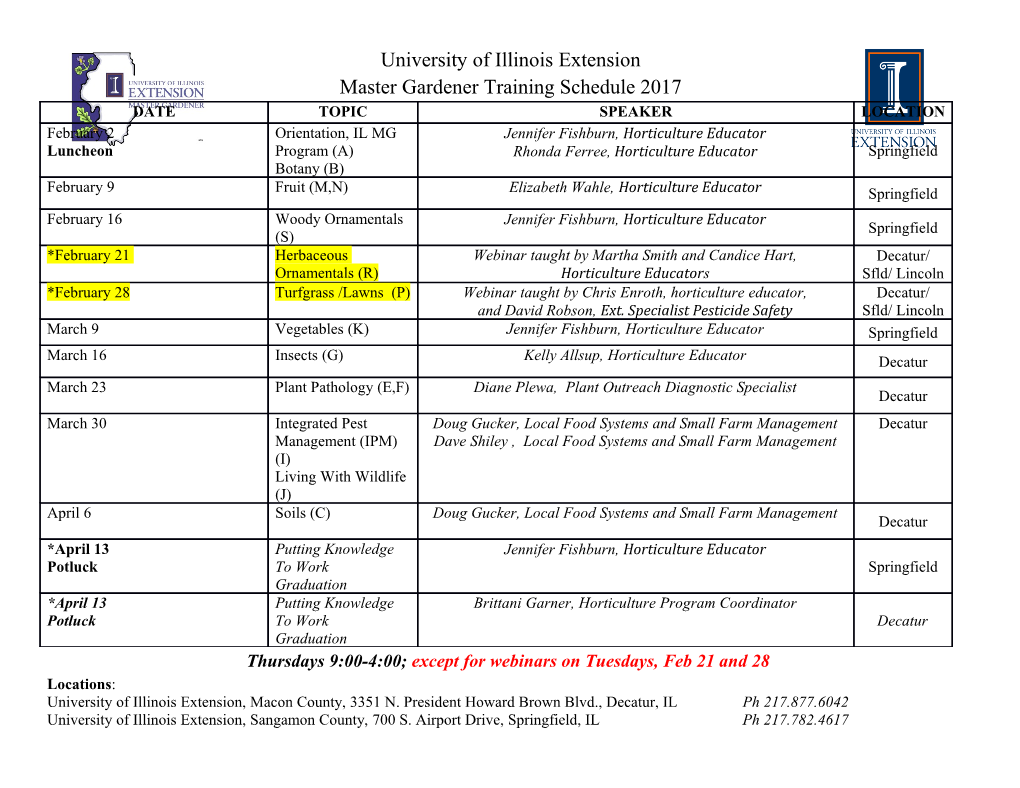
A-1 A Self-Paced Brain Computer Interface (BCI) Based Point-And-Click System Xin Yi Yong*, Rabab Ward, Gary Birch Background and Objective Point-and-dwell assistive systems have the difficulty of identifying whether the user’s aim is to make a selection or to obtain information. To overcome this, our lab looked into the feasibility of developing a point-and-click system that uses an eye-tracker for ‘pointing?and a self-paced brain computer interface (BCI) for ‘clicking? i.e., selection-making. The first step was to implement a point-and-click system with a simulated BCI switch. Our study investigates: (i) the typing speed the system can achieve with the state- of-the-art BCI system. (ii) the use of eye-trackers in detecting eye blinks and saccades. (iii) how well movement-related EEG trials collected from the experiments can be classified. (iv) the effects of artefacts (ocular, facial muscle, head movement, etc.) on system performance, and how to deal with them. Methods A point-and-click eye-tracking system was implemented to operate an on-screen keyboard [1] for text- entry purposes. EEG signals were recorded at a sampling rate of 128 Hz from 15 electrodes positioned over the motor cortex area. EOG, facial EMG, and eye-tracker data were also recorded. Eight able- bodied subjects were asked to point at the desired target using an eye-tracker [2], then attempt a finger extension (detected by a switch) to make a selection. To simulate a real-world BCI system, errors were introduced so that its performance matches the best self-paced BCI system [3] (TPR = 70%, FPR = 1%). The experiment consisted of three to four sessions, each lasting ten minutes. The subjects were asked to type sentences (randomly selected from a database [4]) displayed on the screen. Thirty combinations of bipolar EEG channels were generated and segmented using a sliding hamming window of size 128 samples, with a 93.75% overlap. Eye-blinks and saccades artefacts were detected using the eye-tracker data. Only EEG segments free from these artefacts were processed, using discrete wavelet transform, an autoregressive model of order 8 and power spectral density (1 ?30 Hz). 50 to 200 most discriminative features were selected and classified as either a no-control state (subject not activating the brain switch) and an intentional control state (subject is attempting finger extension). Linear Discriminant Analysis (LDA) was used as a classifier. Results For a BCI-based point-and-click system with a TPR of 70% and FPR of 1%, the typing speeds achieved by the subjects varied from 5.8 to 11.3 correct chars/min. Classification of the EEG trials (using LDA 10x10 cross validation) showed TPR ranging from 40.5% to 92.3%, with an average of 64.2% ?22.6%, for FPR = 1%. Discussion and Conclusions These results are encouraging. The typing speed can be improved as the subjects were novice eye- tracker and on-screen keyboard users. The eye-tracker was reliable in detecting eye blinks and saccades, and does not require additional EOG channels for artefact detection. By using more advanced signal processing algorithms, the accuracy and system performance can be further improved. Currently, we are studying other types of artefacts commonly arise and how much they affect the system performance. Artefact detection and removal algorithms will be developed to make the system more robust and always functional. References [1] The Dynamic Keyboard|CanAssist. < http://www.canassist.ca/dynamic-keyboard > . [2] Mirametrix Eye-tracker. < http://www.mirametrix.com/s1-eye-tracker.html > . [3] A. Bashashati, R. K. Ward, G. E. Birch. Towards development of a 3-state self-paced brain-computer interface. Computational Intelligence and Neuroscience, pages 1 ?8, 2007. [4] I. S. MacKenzie and R. W. Soukoreff. Phrase sets for evaluating text entry techniques. In Ext. Abstracts on Human Factors in Computing Systems CHI 2003, ACM Press, page 754-755, 2003. Keywords Brain computer interface (BCI), point and click system, eye-tracker, Electroencephalograph (EEG) A-10 Feedback Information-Theoretic Paradigms for Designing Brain-Machine Interfaces Abdullah Akce*, Rui Ma, Martin McCormick, Miles Johnson, Cyrus Omar, Edward Maclin, Timothy Bretl, Todd Coleman Background and Objectives We have developed an information-theoretic approach to designing brain-computer interfaces that explicitly takes into account both the inherent uncertainty in measurement and interpretation, and the important role of sensory feedback. Our approach was experimentally shown to enhance the overall BCI performance by using binary EEG motor imagery, and was used to remotely teleoperate an unmanned aircraft flying at a fixed altitude. In order to distinguish between left- and right-hand motor imagery, we also built a Common Spatial Analytical Pattern (CSAP) and Hidden Markov Model (HMM) based classifier. Methods Our approach views BCI as the means by which the intent of the user is communicated to a prosthetic device to accomplish a task. This communication is enhanced by using sensory feedback, in our case a graphical display. We represent the intent as a sequence in an ordered symbolic language with associated statistical language model. We model the EEG device and the graphical display as a noisy binary channel with noiseless feedback. This abstraction allows the problem of interface design to be reformulated as the problem of deriving an optimal communication protocol using tools from feedback information theory. Such a protocol is provided by the combination of arithmetic coding as a classical method of lossless data compression with posterior matching as a capacity-achieving channel code that uses feedback to avoid the necessity of forward error correction. The classifier uses the group’s newly developed common spatial analytic pattern (CSAP) to extract discriminative signals that capture large disparities for each class by viewing it as a blind-source separation problem. These signals are processed by an HMM to perform classification by belief propagation and to account for the independence between consecutive classifications. A classification occurs at a variable rate when the belief probability exceeds a threshold. Results We designed two applications to evaluate our information-theoretic approach: (1) interface for text entry where the intent is an English sentence; and (2) interface for path entry where the intent is a smooth planar curve composed of circular arcs chosen from a finite alphabet. In our experiments with nine able-bodied subjects with limited exposure to EEG motor imagery, the best rates were 6 chars/minute in text spelling and 12.5 arcs/minute in path entry. We also recorded EOG and EMG data to verify that the artifacts were not the enabling factors of the performance. Finally, we demonstrated the feasibility of our approach in the online setting where action needs to be taken while communicating the intent. A human pilot remotely teleoperating a model UAV succeeded in two different target surveillance tasks. We measured the performance of the CSAP-based classifier in a set of experiments with able-bodied subjects and obtained the highest information transfer rate of 60.9 bits/min which is significantly better than the previously reported rate of 37.1 bits/min. Discussion and Conclusions We presented an optimal communication protocol which is not only optimal but also can be easily understood and implemented by a human user. This protocol was used in applications where the task can be modeled as a sequence of symbols. We achieved promising performance gain by employing sensory feedback in the optimal way by using tools from information theory and by developing a classifier that provides a promising information transfer rate. References McCormick, M., Ma, R., & Coleman, T. (2010, March). An Analytic Spatial Filter and A Hidden Markov Model for Enhanced Information Transfer Rate in EEG-based Brain Computer Interfaces? ICASSP, Dallas, TX. (to appear) Akce, A., Johnson, M., & Bretl, T. (2010, May). Remote Teleoperation of an Unmanned Aircraft with a Brain-Machine Interface: Theory and Preliminary Results Int. Conf. Rob. Aut. (ICRA), Anchorage, AK. (to appear) C. Omar, A. Akce, M. Johnson, T. Bretl, R. Ma, E. Maclin, M. McCormick, and T. P. Coleman, "A Feedback Information-Theoretic Approach to the Design of Brain-Computer Interfaces", International Journal on Human-Computer Interaction, special issue on Brain-Computer Interfaces, submitted Nov 2009. Support This research has been sponsored in part to TB and TC by a Seed Grant from the Center for Healthy Minds, funded through NIH/NIA under Award No. P30-AG023101; to TB by awards NSF-CNS-0931871 and NSF-CMMI-0956362-EAGER; to TC by the AFOSR Complex Networks Program via Award No. FA9550- 08-1-0079; and to CO by the NSF Graduate Research Fellowship. Keywords Feedback information theory, electroencephalography (EEG) motor imagery, text speller, path specification, remote teleoperation A-11 Development of a laryngeal surface electromyographic biofeedback system for an efficient neurally-controlled communication interface Emily Mugler*, Patrick Rousche, James Patton Brain-computer interface (BCI) has been applied with increasing frequency and success in recent years for the purposes of neural rehabilitation, augmentation and automated physical therapy. Although there have been significant achievements with repair, replacement and enhancement of motor control and neurological prostheses, similar advancement in the field for communication prostheses has been limited.
Details
-
File Typepdf
-
Upload Time-
-
Content LanguagesEnglish
-
Upload UserAnonymous/Not logged-in
-
File Pages268 Page
-
File Size-