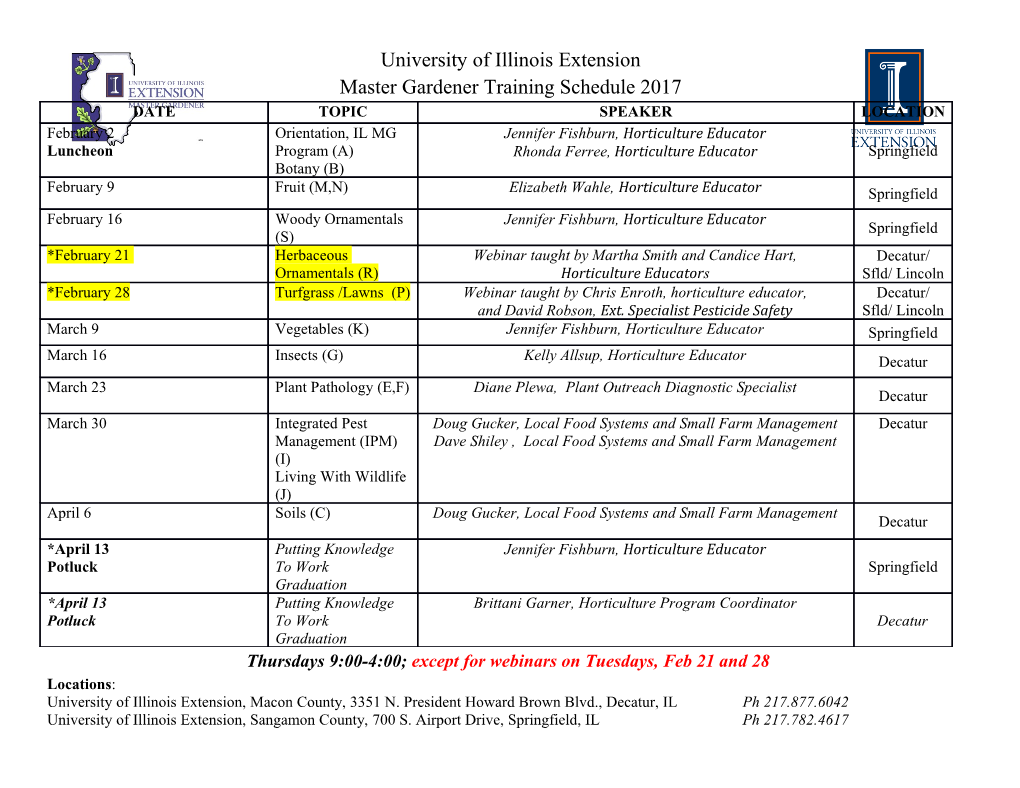
Annals of Pure and Applied Mathematics Vol. 20, No. 1, 2019, 35-47 Annals of ISSN: 2279-087X (P), 2279-0888(online) Published on 11 September 2019 www.researchmathsci.org DOI: http://dx.doi.org/10.22457/apam.623v20n1a6 Symmetrical Trapezoidal Normal Distribution and its Parameter Estimation Soontorn Boonta 1 and Somchit Boonthiem 2 1Department of General Science, Kasetsart University Sakon Nakhon 47000, Thailand . E-mail: [email protected] 2Mathematics and Statistics Program, Sakon Nakhon Rajabhat University, Sakon Nakhon 47000, Thailand. E-mail: [email protected] Received 20 July 2019; accepted 2 September 2019 Abstract. The trapezoidal rule is applied for construction a new distribution based on normal distribution, is called trapezoidal normal distribution under the same conditions. Furthermore, we illustrate the parameter estimation of the trapezoidal normal distribution using standard differential evolution (DE) algorithm for the sample sizes equal to 10 to 100. The results show the K-S statistic of the trapezoidal normal distribution is less than the K-S statistic of the normal distribution of each sample size data. Keywords: Trapezoidal normal distribution, Differential evolution algorithm. AMS Mathematics Subject Classification (2019): 62F10 1. Introduction Normal distribution is one of the most important continuous probability distribution which is widely used in statistical analysis and other fields such as biology, economics, engineering, and so forth. The random variable X is called a normal distribution with the real location parameter µ and the positive scale parameter σ , denoted by X∼ N (µ, σ 2 ) and its probability density function is of the form −µ 2 −1x 1 σ φ()x;, µ σ = e2 ,. x ∈ ℝ X σ2 π The cumulative distribution function is of the form x F()() x;,µσ= φ x ;, µσ dx . X∫−∞ X Obviously, it is too complicated to directly calculate the value of the cumulative ( µ σ ) distribution function. However, there are many articles that estimate FX x ; , using different methods see e.g. [1-4]. In addition, an application of normal distribution was 35 Soontorn Boonta and Somchit Boonthiem used to join exponential distribution and to determine the inefficiency level of the firms due to the effect of exogenous factors [5]. Furthermore, a few researchers studied some properties of generalized trapezoidal distribution and its applications which triangular and uniform distributions are special cases of the trapezoidal family. Van Dorp and Kotz [6] allows for a non-linear behavior at its tails and a linear incline (or decline) in the central stage and another. Van Dorp et al. [7] developed two novel elicitation procedures for the parameters of a special case of the generalized trapezoidal family by restricting ourselves to a uniform (horizontal) central stage in accordance with the central stage of the original trapezoidal distribution. In this paper, we shall introduce a new distribution based on normal distribution which is called trapezoidal normal distribution by applying trapezoidal rule. 2. Definitions and main theorems We propose definition, theorem and corollary that relate to the probability density function g( x ;Ρ , φ ) in case of 2n + 1 nodes and 2n + 2 nodes. ≥ ℓ > Ρ = { … } Definition 1. Let k 3 be an integer, ,d 0 be real numbers. A set x1, x 2 , , x k ( ℓ ) < <⋯ < −= − = ℓ is called a k, x1 , , d node if x1 x 2 x k such that x2 x 1 xk x k − 1 and − = =… − xi+1 x i d , i2,3, , k 2. Ρ ( ℓ ) The node is denoted by the notation Node k, x1 , , d . Remark 1. Let k ≥ 3 be an integer, ℓ,d > 0 be real numbers. Ρ ( ℓ ) = + ℓ = +ℓ +( − ) 1.1 If be a k, x1 , , d node, x2 x 1 , xxk 1 2 kd 3 and = ++ℓ ( − ) =… − xi+1 x 1 id1 , where i2,3, , k 2. 1.2 Let f be a function and I be the identity function on Ρ, we denote ∆ ( ) =( ) − ( ) f x k fxk fx k −1 ∆ =∆( ) = − xk Ixk x k x k −1 1 f() x =()fx()() + fx − k 2 k k 1 1 x =Ix() =() xx + − . k k2 k k 1 Remark 2. Let k≥3, n ≥ 1 be integers, ℓ,d > 0 be real numbers and C be a center node. 1 2.1 CeNode(2 n+ 2, C ,ℓ , d ) =Noden2 +−−− 2, C n dℓ , ℓ , d is called even node. 2 2.2 CoNode(2 n+ 1, C ,ℓ , d ) =Noden(2 +−−− 1, C( n 1) dℓ , ℓ , d ) is called odd node. 36 Symmetrical Trapezoidal Normal Distribution and its Parameter Estimation Theorem 3. Let µ∈ℝ, σ > 0,d > 0, n ∈ ℕ , φ be a probability density function 2n µ σ 2 Ρ = { … } ( + µ ℓ ) <φ () < of N ( , ) , and x12, x , , x 21n+ be CoNode2 n 1, , , d . If 0∑ xk d 1 , k =3 1 2n ℓ =1 − φ ()x d and φ () ∑ k x2 k =3 φ ( x ) φ () +2 () − <≤ x2 xx 212 ; xxx , ℓ ∆φ ()x ( Ρ φ ) =φ () +k () − <≤= … (1) g x ; , xk xxxxxk kkk;−1 , 3,4, ,2 n d φ ()x φ () −2n () − <≤ x2n xx 22 nnn; xxx 21+ , ℓ when the other of g( x ;Ρ , φ ) is zero. Then g( x ;Ρ , φ ) is a probability density function. ∞ Proof: We will show that g( x ;Ρ ,φ ) ≥ 0 for all x ∈ℝ and gx();,Ρφ dx = 1 . ∫−∞ φ ( ) [ ] φ ( ) [ ] Since x is increasing on x1, x n+ 1 and x is decreasing on xn+1, x 2 n + 1 , ∞ x + g( x ;Ρ ,φ ) ≥ 0 for all x . Next, we consider gx()();,Ρφ dx =2n 1 gx;, Ρ φ dx so we ∫−∞ ∫ x1 have 2n ∞ x2 xk x 2n + 1 g() x;Ρ ,φ dx =Ρ+∫gx()()();,φ dx∑ ∫ gx;, Ρ+ φ dx ∫ gx;, Ρ φ dx ∫−∞ x x− x 1 k =3 k 1 2 n φ( x)ℓ2n φ ( x ) ℓ =2 +φ () + 2 n ∑ xk d 2k =3 2 φ( x)ℓ2n φ ( x ) ℓ =2 +φ () + 2 ∑ xk d 2k =3 2 2n =φ()()ℓ + φ x2 ∑ xk d . k =3 1 2n Since ℓ =1 − φ ()x d is an appropriate value, φ () ∑ k x2 k =3 x ∞ 2n+ 1 gx()();,Ρφ dx ==1 gx ;, Ρ φ dx ∫ ∫ −∞ x1 Therefore, g( x ;Ρ , φ ) is a probability density function. □ 37 Soontorn Boonta and Somchit Boonthiem Theorem 4. Let µ∈ℝ, σ > 0,d > 0, n ∈ ℕ , φ be a probability density function of 2n+ 1 µ σ 2 Ρ = { … } ( + µ ℓ ) <φ () < N ( , ) , and x12, x , , x 22n+ be CeNode2 n 2, , , d . If 0∑ xk d 1 , k =3 1 2n+ 1 ℓ =1 − φ ()x d and φ () ∑ k x2 k =3 φ ( x ) φ () +2 () − <≤ x2 xx 2 ; xxx 12 , ℓ ∆φ ()x ( Ρ φ ) =+φ () k () − <≤=… + g x ; , xk xx k ; xxxk kk−1 , 3,4, ,2 n 1 (2) d φ ()x φ () −2n+ 1 () − <≤ x21n+ xx 21 nnn +++; xxx 21 22 , ℓ when the other of g( x ;Ρ , φ ) is zero. Then g( x ;Ρ , φ ) is a probability density function. Proof: The proof of Theorem 4 follows from Theorem 3. Definition 2. The random variable X has trapezoidal-normal distribution for parameters (µ, σ ) such that µ ∈ℝ and σ > 0 corresponding to Conode(2 n+ 1,µ ,ℓ , d ) or Cenode(2 n+ 2,µ ,ℓ , d ) denoted by XTN∼ (µ, σ 2 , n , Ρ) , if there exists a probability density function satisfying equations (1) and (2), respectively. Definition 3. A probability distribution is said to be symmetric if and only if there exists ( −δ) =( + δ ) δ a value x0 such that fx0 fx 0 for all real number , where f is the probability density function if the distribution is continuous or the probability mass function if the distribution is discrete. Theorem 5. Let g( x ;Ρ , φ ) be the probability density function of trapezoidal-normal distribution for parameters (µ, σ ) , g(µ−Ρ x;, φ) = g( µ +Ρ x ;, φ ) for all real number x , g( x ;Ρ , φ ) is called symmetric. ∈[ ] ∈ ℝ Proof: Let x xk, x k +1 for all x . Let x lie in a segment that has the first point, φ ( ) φ ( ) ( xk, x k ) and the end point, ( xk+1, x k + 1 ) . We have a straight line equation in the form 38 Symmetrical Trapezoidal Normal Distribution and its Parameter Estimation ∆φ ( x ) yx() =k +1 ()() xx − + φ x . k∆ k k xk +1 Since φ ( x) is symmetry, φµ( −x) = φµ( + x ) . We will show that g(µ+Ρ x;, φ) = g( µ −Ρ x ;, φ ) . Here, we consider only 5 cases of odd node and similarity to consider of even node: µ + < µ − > Case 1. For x x 1 , we get x x 2n+ 1 . µ−> µ − = (µ+ Ρ φ ) = (µ− Ρ φ ) = Since x2 x1 x 2n+ 1 , we have g x ; , 0 and g x ; , 0 . Thus, g(µ+Ρ x;, φ) = g( µ −Ρ x ;, φ ) . µ + > Case 2. For x x 2n+ 1 , by similar process as in Case 1, we get gx(µφµ+Ρ=;,) g( −−Ρ=( x) ;, φµφ) gx( −Ρ ;, ) <µ + < µ+( µ + Ρ φ ) Case 3. For x1 x x 2 , we get ( x, g x ; , ) lie in a segment that has a straight line equation ∆( Ρ φ) φ ( ) g x2 ; , x 2 yx() = +Ρ= gx(); , φ () xx − 1 ∆ 1ℓ 1 x2 Thus, φ( x) φ ( x ) gxyx()()µφµ+Ρ=; , +=2() µ +−= xx 2 ()() µ +−+ xxx φ 1 ℓ1 ℓ 2 2 <µ − < (µ−( µ − Ρ φ )) Conversely, we consider for x2n x x 2 n + 1 , we get x, g x ; , lie in segment that has straight line equation ∆( Ρ φ) φ ( ) g x2n+ 1; , x 2 n yx() =()() xxgx ++Ρ=−; , φ()() xxx −+ φ 2n ∆ 2n 2 n ℓ 2n 2 n x2n+ 1 Thus, (µ− Ρ φ ) =(µ − ) g x ; , y2n x φ ( x ) =−2n ()()µ −−x x + φ x ℓ 2n 2 n φ ( x ) =2 ()()µ +−+x x φ x .
Details
-
File Typepdf
-
Upload Time-
-
Content LanguagesEnglish
-
Upload UserAnonymous/Not logged-in
-
File Pages13 Page
-
File Size-