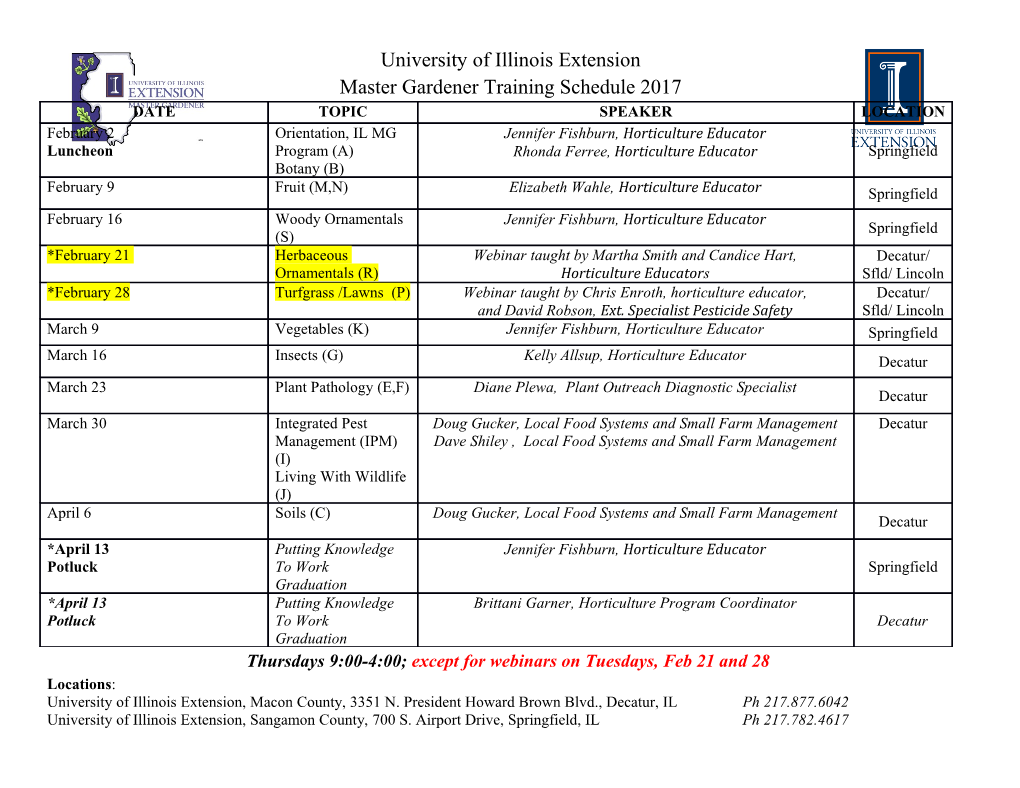
D.G. Bonett (8/2018) Module 3 One-factor Experiments A between-subjects treatment factor is an independent variable with a 2 levels in which participants are randomized into a groups. It is common, but not necessary, to have an equal number of participants in each group. Each group receives one of the a levels of the independent variable with participants being treated identically in every other respect. The two-group experiment considered previously is a special case of this type of design. In a one-factor experiment with a levels of the independent variable (also called a completely randomized design), the population parameters are 휇1, 휇2, …, 휇푎 where 휇푗 (j = 1 to a) is the population mean of the response variable if all members of the study population had received level j of the independent variable. One way to assess the differences among the a population means is to compute confidence intervals for all possible pairs of differences. For example, with a = 3 levels the following pairwise comparisons of population means could be examined. 휇1 – 휇2 휇1 – 휇3 휇2 – 휇3 In a one-factor experiment with a levels there are a(a – 1)/2 pairwise comparisons. Confidence intervals for any of the two-group measures of effects size (e.g., mean difference, standardized mean difference, mean ratio, median difference, median ratio) described in Module 2 can be used to analyze any pair of groups. For any single 100(1 − 훼)% confidence interval, we can be 100(1 − 훼)% confident that the confidence interval has captured the population parameter and if v 100(1 − 훼)% confidence intervals are computed, we can be at least 100(1 − 푣훼)% confident that all v confidence intervals have captured their population parameters. For example, if 95% confidence intervals for 휇1 – 휇2, 휇1 – 휇3, and 휇2 – 휇3 are computed, we can be at least 100(1 − 3훼)% = 100(1 – .15)% = 85% confident that all three confidence intervals have captured the three population mean differences. When considering v confidence intervals for some measure of effect size, the researcher would like to be at least 100(1 − 훼)% confident, rather than at least 100(1 − 푣훼)% confident, that all v confidence intervals will capture the v population effect size values. One simple way to achieve this is to use a Bonferroni adjustment 훼* = 훼/v rather than 훼 in the critical t-value or critical z-value for each confidence interval. 1 D.G. Bonett (8/2018) When examining all possible pairwise differences, the Tukey-Kramer method yields a narrower confidence interval than the Bonferroni method. The classical Tukey-Kramer method for comparing all possible pairs of means assumes equal population variances, but a version of the Tukey-Kramer method that does not require equal population variances is available. SPSS provides an option to compute Games-Howell confidence intervals for all pair-wise comparisons of means that are the same as the unequal variance version of the Tukey-Kramer confidence intervals. The Tukey-Kramer and Games-Howell methods are used only when the researcher is interested in examining all possible pairwise differences. A Bonferroni confidence interval will be narrower than a Tukey- Kramer or Games-Howell confidence interval if, prior to an examination of the sample results, the researcher is interested in only u < v of the v = a(a – 1)/2 possible pairwise comparisons. For u planned comparisons, the Bonferroni adjustment is 훼* = 훼/u. However, if u of the v possible pairwise comparisons appear interesting after an examination of the sample results, it is necessary to use 훼* = 훼/v and not 훼* = 훼/u. Example 3.1. There is considerable variability in measures of intellectual ability among college students. One psychologist believes that some of this variability can be explained by differences in how students expect to perform on these tests. Ninety undergraduates were randomly selected from a list of about 5,400 undergraduates. The 90 students were randomly divided into three groups of equal size and all 90 students were given a nonverbal intelligence test (Raven’s Progressive Matrices) under identical testing conditions. The raw scores for this test range from 0 to 60. The students in group 1 were told that they were taking a very difficult intelligence test. The students in group 2 were told that they were taking an interesting “puzzle”. The students in group 3 were not told anything. Simultaneous Tukey-Kramer confidence intervals for all pairwise comparisons of population means are given below Comparison 95% Lower Limit 95% Upper Limit 휇1 – 휇2 -5.4 -3.1 휇1 – 휇3 -3.2 -1.4 휇2 – 휇3 1.2 3.5 The researcher is 95% confident that the mean intelligence score would be 3.1 to 5.4 greater if all 5,400 undergraduates had been told that the test was a puzzle instead of a difficult IQ test, 1.4 to 3.2 greater if they all had been told nothing instead of being told that the test is a difficult IQ test, and 1.2 to 3.5 greater if they all had been told the test was a puzzle instead of being told nothing. The simultaneous confidence intervals allow the researcher to be 95% confident regarding all three conclusions. Linear Contrasts Some research questions can be expressed in terms of a linear contrast of population means, ∑푎 푐 휇 , where 푐 is called a contrast coefficient. For example, 푗=1 푗 푗 푗 2 D.G. Bonett (8/2018) in an experiment that compares two costly treatments (Treatments 1 and 2) with a new inexpensive treatment (Treatment 3), a confidence interval for (휇1 + 휇2)/2 – 휇3 may provide valuable information regarding the relative costs and benefits of the new treatment. Statistical packages and various statistical formulas require 푎 linear contrasts to be expressed as ∑푗=1 푐푗휇푗 which requires the specification of the contrast coefficients. For example, (휇1 + 휇2)/2 – 휇3 can be expressed as (½)휇1 + (½)휇2 + (-1)휇3 so that 푐1= .5, 푐2 = .5, and 푐3= -1. Consider another example where Treatment 1 is delivered to groups 1 and 2 by experimenters A and B and Treatment 2 is delivered to groups 3 and 4 by experimenters C and D. In this study we may want to estimate (휇1 + 휇2)/2 – (휇3 + 휇4)/2 which can be expressed as (½)휇1 + (½)휇2 + (-½)휇3 + (-½)휇4 so that 푐1= .5, 푐2 = .5 푐3= -.5 and 푐4= -.5. 푎 A 100(1 − 훼)% unequal-variance confidence interval for ∑푗=1 푐푗휇푗 is 2̂2 푎 푎 푐푗 휎푗 ∑푗=1 푐푗휇̂푗 푡훼/2;푑푓√ ∑푗=1 (3.1) 푛푗 2 2 2 4 4 푎 푐푗 휎̂푗 푎 푐푗 휎̂푗 where df = [∑푗=1 ] /[ ∑푗=1 2 ]. When examining v linear contrasts, 훼 can 푛푗 푛푗 (푛푗−1) be replaced with 훼* = 훼/v in Equation 3.1 to give a set of Bonferroni simultaneous confidence intervals. If the sample sizes are approximately equal and there is convincing evidence from previous research that the population variances are not highly dissimilar, then the unequal-variance standard error in Equation 3.1 could be replaced with an equal- 2 푎 2 2 푎 2 variance standard error√휎̂푝 ∑푗=1 푐푗 /푛푗 where 휎̂푝 = [∑푗=1(푛푗 − 1) 휎̂푗 ]/푑푓 and 푎 df = (∑푗=1 푛푗) − 푎. Standardized Linear Contrasts In applications where the intended audience may be unfamiliar with the metric of the response variable, it could be helpful to report a confidence interval for a standardized linear contrast of population means which is defined as 푎 ∑푗=1 푐푗휇푗 휑 = 푎 2 √∑푗=1 휎푗 /푎 and is generalization of the standardized mean difference defined in Module 2. The denominator of 휑 is called the standardizer. Some alternative standardizers have 3 D.G. Bonett (8/2018) been proposed for linear contrasts. One alternative standardizer averages variances across only those groups that have a non-zero contrast coefficient. Another standardizer uses only the variance from a control group. Although not recommended for routine use, the most popular standardizer is the square root of 2 휎̂푝 defined above, which can be justified only when the population variances are approximately equal or the sample sizes are equal. An approximate equal-variance 100(1 − 훼)% confidence interval for 휑 is 휑̂ ± 푧훼/2푆퐸휑̂ (3.2) 푎 푎 2 2 2 푎 1 푎 2 where 휑̂ = ∑푗=1 푐푗휇̂푗/√(∑푗=1 휎̂푗 )/푎 and 푆퐸휑̂ = √(휑̂ /2푎 ) ∑푗=1 + ∑푗=1 푐푗 /푛푗. 푛푗−1 An unequal-variance confidence interval for 휑 is available and is recommended in studies with unequal sample sizes. When examining v linear contrasts, 훼 can be replaced with 훼* = 훼/v in Equation 3.2 to give a set of Bonferroni simultaneous confidence intervals. Example 3.2. Ninety students were randomly selected from a research participant pool and randomized into three groups. All three groups were given the same set of boring tasks for 20 minutes. Then all students listened to an audio recording that listed the names of 40 people who will be attending a party and the names of 20 people who will not be attending the party in random order. The participants were told to simply write down the names of the people who will attend the party as they hear them. In group 1, the participants were asked to draw copies of complex geometric figures while they were listening to the audio recording and writing. In group 2, the participants were not told to draw anything while listening and writing. In group 3, the participants were told to draw squares while listening and writing. The number of correctly recorded attendees was obtained from each participant.
Details
-
File Typepdf
-
Upload Time-
-
Content LanguagesEnglish
-
Upload UserAnonymous/Not logged-in
-
File Pages24 Page
-
File Size-