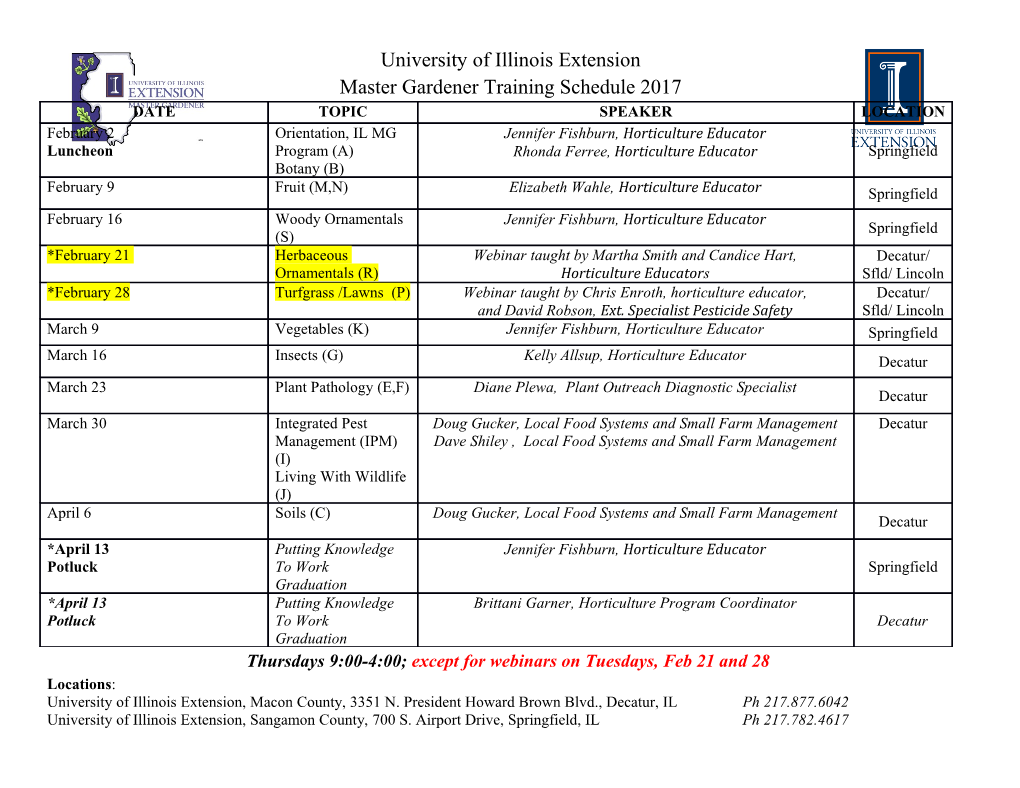
Chapter Eight Conclusion Jorge Luis Romeu IIT Research Institute Rome, NY 13440 June 4, 1999 Executive Summary In this last chapter we summarize what has been accomplished in the areas of statistical analysis of materials data. We also discuss possible topics for future work in the area of statistical training for materials engineers and scientists. Finally, we overview the data sets and software used in the case studies presented. What Has Been Accomplished As we explained in the Introduction chapter, the objective of this SOAR is to provide support and extension materials for better understanding the data analysis statistical procedures in handbooks [6, 7]. It can also be used as reading material by practitioners in statistical analysis, either as a methods refresher or as a discussion on the statistical thinking behind the procedures used in the mentioned handbooks. We have covered several important topics. We have discussed univariate and bivariate statistical methods, for both, qualitative and quantitative variables. We have seen the most important and frequently used statistical distributions in materials data analysis. Among the continuous distributions, we have discussed the Normal, Lognormal and Weibull. Among the discrete distributions we have worked with the Binomial and the Discrete Uniform. We have discussed the meaning, interpretation, use and estimation of distribution parameters and other performance measures of interest. These include measures of central tendency such as the mean, median and mode and measures of dispersion such as the variance, ranges and coefficient of variation. Finally, we have discussed other measures of location such as the percentiles, which are relevant to the study of the concepts of A and B basis allowables. We have discussed and provided contextual examples for several sampling distributions of interest, such as the Student t and Fisher’s F distributions, used in the comparisons of two or more samples. We have also discussed the Chi-Square distribution, useful in testing for association and for testing the variance of a sample. Finally, we have discussed (and provided examples for) the use of several non-parametric distributions, especially for the Anderson-Darling, Mann-Whitney and Kruskal-Wallis non-parametric tests for the comparisons of two or more samples. We have discussed in detail (and have provided GoF examples of) the important problem of establishing the (underlying population) distribution of a sample. We have then discussed (and provided several graphical and analytical procedures for) detecting potential outliers in a data set. For, once a statistical distribution has been established, we want to know if all the data in the set conform to it. We have also discussed the serious problems and consequences of detecting and mechanically discarding outliers. We have discussed in detail the implementation of confidence intervals and hypothesis tests, for certain distribution parameters of interest. We have also provided contextual examples and step-by-step procedures for developing them. In particular, we have discussed the derivation of large and small sample confidence intervals for the mean. We have also presented practical examples of the derivation of confidence bounds and tolerance bounds and intervals. We have discussed in detail the implementation of hypothesis tests for the mean of one and two populations and the tests of association between two qualitative variables (contingency tables). We have also provided contextual examples and step-by-step procedures for developing them. We have provided interpretations for types I and II errors and their corresponding risks. We have discussed the problems of establishing the sample size for a pre-specified confidence interval and their statistical consequences. We have discussed the problem of the implementation of one-way analysis of variance (ANOVA), the model assumptions and several graphical and analytical procedures to check their validity. We have discussed procedures for comparing the means of several samples and for establishing joint confidence intervals for their differences. For, these are required once the ANOVA procedure detects that the population means do differ. We have discussed the problem of implementing simple linear regression and non-linear (quadratic and cubic) regressions. We have discussed the regression model assumptions and presented several graphical and analytical procedures to check their validity. We have also discussed the important problem of fitting several (linear and non-linear) regression models to the same data set and then selecting the one that best fits (or describes) the underlying problem structure. Finally, we have discussed, in detail, the dilemma of modeling the data instead of the problem, ever present in data analysis. Finally, we have presented two complete chapters discussing case studies of real life data analyses: one in ANOVA and another one in regression, respectively. The data have been borrowed from the handbooks [6, 7] and the RECIPE materials data analysis program [5]. We have developed these case studies as we would have analyzed them for a research project. We have started with the EDA (exploratory data analysis) description of the data. We have proceeded to the formulation of conjectures (hypotheses) that would have then been tested. Finally, we have actually tested these hypotheses via the implementation of different statistical models, such as ANOVA, regression, t and F and non-parametric tests. We have checked, in each case, the validity of model or test assumptions. Finally, we have derived the corresponding statistical and problem context conclusions. Data and Software Two necessary ingredients for data analysis work are the data (raw material) and the analysis tools (statistical software). In the previous chapters we have thoroughly used and discussed both of them. We will now provide some other technical details about them. At the start of this SOAR we dedicated an entire chapter to discuss data, its quality and its pedigree. We did this both, from the specialized point of view of the materials data analysis as well as from the purely statistical one. We included a long list of materials data literature sources, for the interested reader to pursue this topic further. We also discussed ways to check that the data used is reliable, via the materials science concepts of data pedigree, validation and accreditation. This says how important is data to us. Then, we extensively used several real data sets, that we identified in the corresponding chapters. We present an annotated list in Appendices 2 and 3. For the SOAR readers convenience we also include text files of these data sets. With this, we hope to encourage the readers to redo the analyses and gain the practical experience. To implement these analyses some statistical software is needed. We have tried to keep such need to a bare minimum by limiting our own use to two packages: Microsoft Office Excel and Minitab. Both can be used in the analyses. Many of these analyses were and can be readily implemented using Excel spreadsheet formats and its linear regression and graphical capabilities. Minitab is a specialized (statistical) software package widely used in statistical education. Minitab web page is http://www.minitab.com/. We have used the student version of Minitab, which is independently available at a nominal cost. It also comes with many general statistics book. It is easy to learn and use and this author has utilized it for years, in his statistics college courses. Any other statistical software (e.g. SAS, S-Plus, SPSS, etc.) would also do. For, the procedures used in this SOAR are very general and are included in most statistics software packages. We have deliberately used both, Excel and Minitab, to make the point that these procedures are at the reach of almost any one who has access to a PC. No special endorsement should be inferred about either software. Finally, there are several specialized materials data analysis statistical packages in the Web, of easy and free access,. The reader can find some of them in the NIST Statistical Engineering Division’s Web Page: http://www.itl.nist.gov/div898/. We have not used this specialized software here because the intent of this SOAR has been to discuss the implementation of statistical procedures. The use of specialized software in our analyses would have defeated such SOAR intent. In our next and final section, we propose several subjects for future or extension work. The development of training materials that discuss the access and use of such specialized statistical software, is one of them. Future Work We have accomplished several objectives in this SOAR. However, this is only the beginning of a long journey into the vast world of statistical data analysis. The interested traveler may want to find out what are some other possible topics that might follow. So far, we have seen material on univariate and bivariate data analysis. When there are more than two pieces of information per observation (say k pieces) we have a k-variate (observation) random vector. Then, we deal with k-variate (in general multivariate) data analysis. The statistical problems discussed for the case of one and two dimensions are now magnified by the higher dimensionality of the problems. However, the analysis possibilities also increase proportionately. For, now we can look at the relationships between the random vector components in multiple and very interesting ways. We
Details
-
File Typepdf
-
Upload Time-
-
Content LanguagesEnglish
-
Upload UserAnonymous/Not logged-in
-
File Pages6 Page
-
File Size-