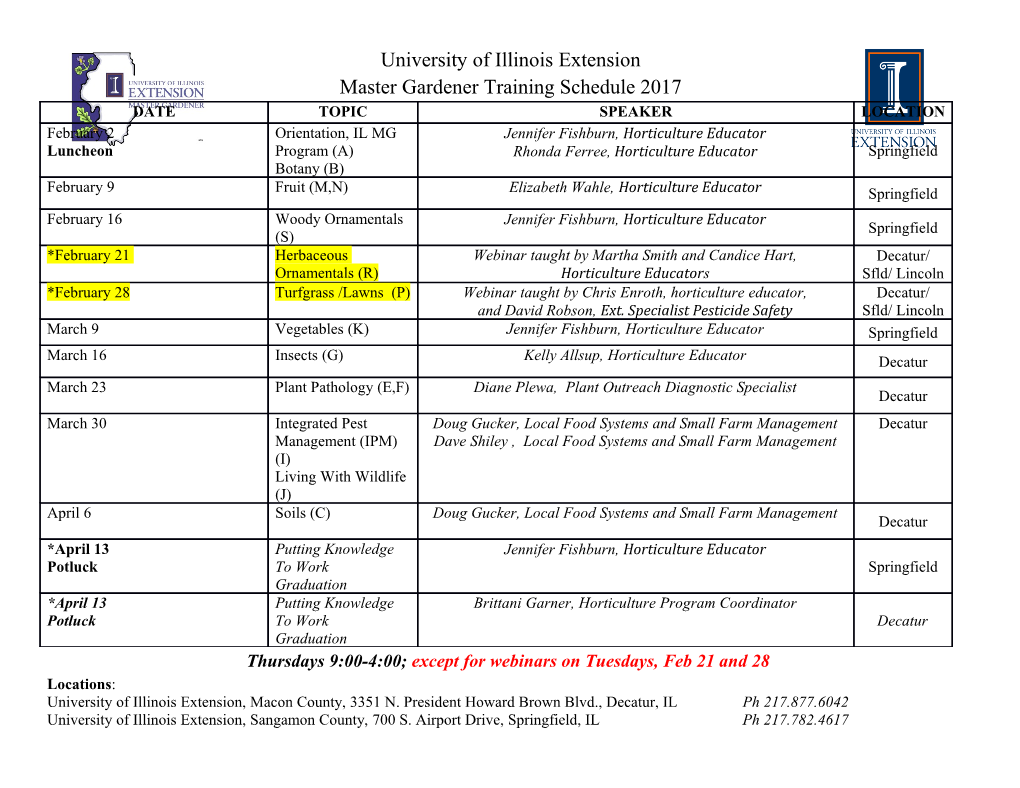
List of Symbols V solution space for the global optimization problem d(·, ·) distance function in the space V dH (·, ·) Hamming distance function · 2 Euclidean norm in the space V · arbitrary norm in the space V N dimension of the global optimization problem (N =dim(V )) D admissible set of solutions to the global optimization problem Φ : D→R+ objective function of a maximization problem Φ˜ : D→R+ objective function of a minimization problem x∗ global maximizer (or minimizer) to the global optimization problem x+ local maximizer (or minimizer) to the global optimization problem meas(·) Lesbegue measure function Loc set of local optimization methods loc(·) local optimization method Rloc attractor of the local minimizer x+ with respect to the strictly x+ decreasing local optimization method loc(·) B + x+ basin of attraction of the local minimizer x P random sample, population Pr(·) probability measure M(A) space of probabilistic measures over the set A b(·) function that selects the best fitted individual in a population Dr ⊂D grid of encoded points (set of phenotypes) r =#Dr the cardinality of phenotype set U genetic universum (set of genotypes) code : U →Dr encoding bijective function dcode : D −→ U partial function of inverse encoding (decoding partial function) Ω binary genetic universum in Chapters 3 - 5, space of elementary events in Section 6.1.5 l binary code length Z2 group {0, 1} with the addition modulo 2 204 List of Symbols ⊕ the addition operator in Z2, coordinate-by-coordinate addition of binary vectors from Z2×,...,×Z2 codea(·) affine binary encoding codeG(·) Gray binary encoding f : U → R+ fitness function Scale(·) nonlinear function that modifies fitness µ number of individuals in the parental population self (·) probability distribution of selection Elite subset of individuals that pass to the next epoch with the probability 1 1 vector of l ones ˆi the inverse of the binary vector i (ˆi = 1 ⊕ i) pm rate of binary mutation pc rate of binary crossover [·] evaluation function for boolean expressions ⊗ coordinate-by-coordinate multiplication of binary vectors type type of binary crossover mutx(·) probability distribution for the binary mutation of an individual x crossx,y(·) probability distribution for the binary crossover of individuals x and y N (e, C) realization (result of sampling) of the N-dimensional Gauss random variable with the mean vector e and the covariance matrix C N (e, σ) realization (result of sampling) of the one dimensional Gauss random variable with the mean value e and the standard deviation σ U[0, 1] realization (result of sampling) of the random variable of the uniform probability distribution over the real interval [0, 1] λ number of offspring individuals in a single genetic epoch κ individual life time parameter of the evolutionary algorithm in section 5.3.2, raster resolution in section 6.1.10 S space of schemata H schemata from the space S ∆(H) length of the schemata H ℵ(H) degree of the schemata H E(·) expected value operator E space of states of genetic algorithms eqp equivalence relation among vectors of genotypes Sµ group of permutations of the µ-element set Λr−1 unit r − 1 simplex in Rr r−1 Xµ ⊂ Λ finite set of states of the genetic algorithm with finite population (µ<+∞) #A cardinality of the set or multiset A n =#E cardinality of the space of states πt genetic algorithm state probability distribution in the epoch t τ Markov transition function of genetic algorithm states Q probability transition matrix F (·) selection operator for the simple genetic algorithm List of Symbols 205 M(·) mixing operator for the simple genetic algorithm G(·) genetic (heuristics) operator for the simple genetic algorithm M mixing matrix K⊂Λr−1 set of fixed points of the genetic operator G dim(V ) dimension of the vector space V diam(A) diameter of the subset A of a metric space N set of natural numbers Z set of integers Z+ set of nonnegative integers Z+ = N ∪{0} R set of rational numbers R+ set of nonnegative rational numbers top(V ) topology on V (the family of open sets in V ) A the topological closure of the set A in the proper topology (A)− the complement of the set A, i.e. (A)− = V \ A if it is contained in the space V χA the characteristic function of the set A. Assuming A iscontainedinthespaceV χA : V →{0, 1},χA(x)=1if x ∈ A, χA(x)=0otherwise · operator of the upper round “ceiling” · operator of the lower round “floor” Int : R → N function turning back the nearest integer to the argument int(A) interior of the set A in the proper topology 2 diag : RN → RN function turning back the square diagonal matrix diag(v) with the diagonal equal to the vector v supp(g) support of the real valued function g : A → R, supp(g)={x ∈ A; g(x) =0 } I identity mapping I the matrix of linear identity of finite dimensional vector space Dom(f) domain of the function f u(t) vector of parameters that control genetic operations in the epoch t L(Rr → Rr) space of linear mappings from Rr into itself Γ Gamma Euler’s function References 1. Ali M, Storey C (1994) Topographical Multilevel Single Linkage. Journal of Global Optimization 5:349–358. 2. Anderson RW (1997) The Baldwin Effect. Chapter C.3.4.1 in [15]. 3. Anderssen RS, Bloomfield P (1975) Properties of the random search in global optimization. Journal of Optimization Theory and Applications 16:383–389. 4. Arabas J (1995) Evolutionary Algorithms with the varying population cardi- nality and the varying crossover range. PhD Thesis, Warsaw University of Technology, Warsaw (in Polish). 5. Arabas J (2001) Lectures in Evolutionary Algorithms. WNT, Warsaw (in Pol- ish). 6. Arabas J (2003) Sampling measure of an evolutionary algorithm. In: Proc. of 6-th KAEiOG Conf., Łagów Lubuski, 15–20. 7. Arabas J, Michalewicz Z, Mulawka J (1994) GAVaPS – a Genetic Algorithm with Varying Population Size. Proc.ofICEC’94, Orlando, Florida, IEEE Press, 73–76. 8. Arabas J, Słomka M (2000) Pseudo-random number generators in the initial population generation. In: Proc. of 4-th KAEiOG Conf., Lądek Zdrój, 7–12 (in Polish). 9. Archetti Betrò B (1978) On the Effectiveness of Uniform Random Sampling in Global Optimization Problems. Technical Report, University of Pisa. 10. Bäck T, Fogel DB, Michalewicz Z eds. (2000) Evolutionary Computation 1. Basic Algorithms and Operators. Institute of Physics Publishing, Bristol and Philadelphia. 11. Bäck T, Fogel DB, Michalewicz Z eds. (2000) Evolutionary Computation 2. Advanced Algorithms and Operators. Institute of Physics Publishing, Bristol and Philadelphia. 12. Bäck T (1996) Evolutionary Algorithms in Theory and Practice. Oxford Univ. Press. 13. Bäck T (1997) Self–adaptation. Chapter C.7.1 in [15]. 14. Bäck T (1997) Mutation Parameters. Chapter E.1.2 in [15]. 15. Bäck T, Fogel DB, Michalewicz Z (1997) Handbook of Evolutionary Compu- tations. Oxford University Press. 16. Bäck T, Schütz M (1996) Intelligent Mutation Note Control in Canonical Ge- netic Algorithm. Foundation of Intelligent Systems. In: Ras ZW, Michalewicz Zeds.Proc. of 9-th Int. Symp. ISIM’96., LNCS 1079, Springer. 208 References 17. Bagley JD (1967) The Behavior of Adaptive Systems with Employ Genetic and Correlation Algorithms. PhD Thesis, University of Michigan, Dissertation Abstracts International 28(12), 5106B. (Univ. Microfilms No. 68–7556). 18. Bahadur RR (1966) A Note on Quantiles in Large Samples. Annals of Math- ematical Statistics 37:577–580. 19. Beasley D, Bull DR, Martin RR (1993) A Sequential Niche for Multimodal Function Optimization. Evolutionary Computation Vol. 1, No. 2, 101–125. 20. Becker RW, Lago GV (1970) A Global Optimization Algorithm. In: Proc. of Allerton Conf. on Circuits and System Theory, Monticallo, Illinois, 3–15. 21. Bethke AD (1981) Genetic Algorithms as Function Optimizers. PhD Thesis, University of Michigan, Dissertation Abstracts International 41(9), 3503B, (Univ. Microfilms No. 8106101). 22. Betrò B (1981) Bayesian Testing of Nonparametric Hypotheses and its Appli- cation to Global Optimization. Technical Reports CNR-IAMI, Italy. 23. Betrò, B Schoen F(1987) Sequential Stopping Rules for the Multistart Algo- rithm in Global Optimization. Mathematical Programming 38:271–286. 24. Betrò, B Schoen F(1992) Sequential Stopping Rules for the Multistart Algo- rithm in Global Optimization. Mathematical Programming 52:445–458. 25. Beyer HG (1995) Toward a Theory of Evolution Strategies: Self-adoption. Evolutionary Computation 3:311–348. 26. Beyer HG (2001) The Theory of Evolution Strategies. Springer. 27. Beyer HG, Rudolph G (1997) Local Performance Measures. Chapter B.2.4. in [15]. 28. Billingsley P (1979) Probability and Measure. John Willey and Sons, New York, Chichester, Brisbane, Toronto. 29. Boender CGE (1984) The Generalized Multinominal Distribution: A Bayesian Analysis and Applications. PhD Thesis, Erasmus University, Rotterdam, Cen- trum voor Wiskunde en Informatica, Amsterdam. 30. Boender CGE, Rinnooy Kan AHG (1991) On when to Stop Sampling for the Maximum. Journal of Global Optimization 1:331–340. 31. Boender CGE, Rinnoy Kan AHG (1985) Bayesian Stopping Rules for a Class of Stochastic Global Optimization Methods. Technical Report, Econometric Institute, Erasmus University, Rotterdam. 32. Boender CGE, Rinnoy Kan AHG (1987) Bayesian Stopping Rules for Multi- start Global Optimization Methods. Mathematical Programming 37:59–80. 33. Boender CGE, Rinnoy Kan AHG, Vercellis C (1987) Stochastic Optimization. In: Andreatta G, Mason F, Serafini P eds. Advanced School on Stochastics in Combinatorial Optimization. Word Scientific, Singapore. 34. Boender CGE, Rinnoy Kan AHG, Stougie L, Timmer GT (1982) A Stochastic Method for Global Optimization. Mathematical Programming 22:125–140. 35. Boender CGE, Zieliński R (1985) A Sequential Bayesian Approach to Esti- mating the Dimension of a Multinominal Distribution. In: Sequential Methods in Statistics. Banach Center Publications Vol.
Details
-
File Typepdf
-
Upload Time-
-
Content LanguagesEnglish
-
Upload UserAnonymous/Not logged-in
-
File Pages18 Page
-
File Size-