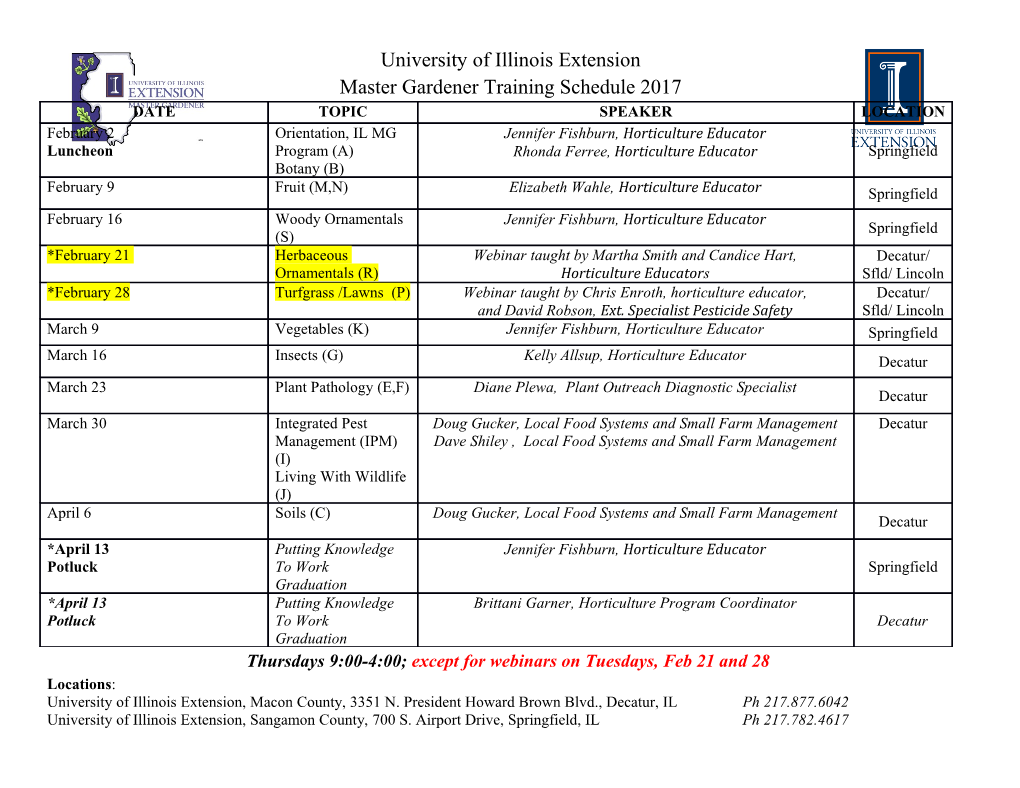
Devarshi Naik et al, / (IJCSIT) International Journal of Computer Science and Information Technologies, Vol. 5 (3) , 2014, 3289 - 3293 A Review on Image Segmentation Clustering Algorithms Devarshi Naik , Pinal Shah Department of Information Technology, Charusat University CSPIT, Changa, di.Anand, GJ,India Abstract— Clustering attempts to discover the set of consequential groups where those within each group are more closely related to one another than the others assigned to different groups. Image segmentation is one of the most important precursors for image processing–based applications and has a crucial impact on the overall performance of the developed systems. Robust segmentation has been the subject of research for many years, but till now published work indicates that most of the developed image segmentation algorithms have been designed in conjunction with particular applications. The aim of the segmentation process consists of dividing the input image into several disjoint regions with similar characteristics such as colour and texture. Keywords— Clustering, K-means, Fuzzy C-means, Expectation Maximization, Self organizing map, Hierarchical, Graph Theoretic approach. Fig. 1 Similar data points grouped together into clusters I. INTRODUCTION II. SEGMENTATION ALGORITHMS Images are considered as one of the most important Image segmentation is the first step in image analysis medium of conveying information. The main idea of the and pattern recognition. It is a critical and essential image segmentation is to group pixels in homogeneous component of image analysis system, is one of the most regions and the usual approach to do this is by ‘common difficult tasks in image processing, and determines the feature. Features can be represented by the space of colour, quality of the final result of analysis. Image segmentation is texture and gray levels, each exploring similarities between the process of dividing an image into different regions such pixels of a region. The result of image segmentation is a set that each region is homogeneous. of regions that collectively cover the entire image, or a set A. K-means Clustering of contours extracted from the image. K-Means algorithm is an unsupervised clustering Robust image segmentation is a difficult task since often algorithm that classifies the input data points into multiple the scene objects are defined by image regions with non- classes based on their inherent distance from each other.[3] homogenous texture and colour characteristics and in order One of the most common iterative algorithms is the K- to divide the input image into semantically meaningful means algorithm [2], broadly used for its simplicity of regions many developed algorithms either use a priori implementation and convergence speed. K-means also knowledge in regard to the scene objects or employ the produces relatively high quality clusters considering the parameter estimation for local texture [1]. low level of computation required. The k-means method Clustering is the search for distinct groups in the feature aims to minimize the sum of squared distances between all space. It is expected that these groups have different points and the cluster centre. K-means algorithm is structures and that can be clearly differentiated. The statistical clustering algorithm. clustering task separates the data into number of partitions, Data clustering is method that creates groups of objects which are volumes in the n-dimensional feature space. (clusters). K-means algorithm is based upon the index of Clustering is a classification technique. Given a vector of N similarity or dissimilarity between pairs of data components. measurements describing each pixel or group of pixels (i.e., K-means algorithm is iterative, numerical, non- region) in an image, a similarity of the measurement vectors deterministic and unsupervised method[4]. K-means and therefore their clustering in the N-dimensional perform well with many data sets, but its good performance measurement space implies similarity of the corresponding is limited mainly to compact groups. pixels or pixel groups. Therefore, clustering in K-Means clustering generates a specific number of measurement space may be an indicator of similarity of disjoint, flat (non-hierarchical) clusters. It is well suited to image regions, and may be used for segmentation purposes. 3289 Devarshi Naik et al, / (IJCSIT) International Journal of Computer Science and Information Technologies, Vol. 5 (3) , 2014, 3289 - 3293 generating globular clusters. The K-Means method is B. Fuzzy c-means numerical, unsupervised, non deterministic and iterative. Fuzzy C-Mean (FCM) is an unsupervised clustering algorithm that has been applied to wide range of problems involving feature analysis, clustering and classifier design. FCM has a wide domain of applications such as agricultural engineering, astronomy, chemistry, geology, image analysis, medical diagnosis, shape analysis, and target recognition. With the developing of the fuzzy theory, the fuzzy c-means clustering algorithm based on Ruspini fuzzy clustering theory was proposed in 1980s[11]. Start Input Image Get the size of image Fig. 2 K-means clustering Process Calculate the possible distance it can achieve Identify the possible number (1) of iteration The procedure follows a simple and easy way to classify a given data set through a certain number of clusters calculate the given (assume k clusters) fixed a priori. The main idea is to define dimensions of image k centroids, one for each cluster. These centroids should be placed in a cunning way because of different location causes different result. So, the better choice is to place them iteration begins as much as possible far away from each other [4]. The next step is to take each point belonging to a given data set and No associate it to the nearest centroid. When no point is pending, the first step is completed and an early group age Possible number of is done. At this point it is necessary to re-calculate k new iterations centroids as bar centers of the clusters resulting from the previous step. After obtaining these k new centroids, a new Yes binding has to be done between the same data set points and the nearest new centroid. A loop has been generated. As a Stop result of this loop, one may notice that the k centroids change their location step by step until no more changes are Fig. 3 Fuzzy c-means clustering Process done. In other words centroids do not move any more. An image can be represented in various feature spaces, Finally, this algorithm aims at minimizing an objective and the FCM algorithm classifies the image by grouping function, in this case a squared error function [4]. similar data points in the feature space into clusters. This clustering is achieved by iteratively minimizing a cost K-means algorithm has three basic disadvantages [4]: function that is dependent on the distance of the pixels to K, the number of clusters must be determined. the cluster centers in the feature domain. The pixels on an Different initial conditions produce different results. image are highly correlated, i.e. the pixels in the immediate The data far away from center pull the centers away neighbourhood possess nearly the same feature data. from optimum location. Therefore, the spatial relationship of neighbouring pixels is an important characteristic that can be of great aid in The entire document should be in Times New Roman or imaging segmentation. Times font. Type 3 fonts must not be used. Other font The FCM algorithm assigns pixels to each category by types may be used if needed for special purposes. using fuzzy memberships. Let X=(x1, x2,.,xN) denotes an image with N pixels to be partitioned into c clusters, where 3290 Devarshi Naik et al, / (IJCSIT) International Journal of Computer Science and Information Technologies, Vol. 5 (3) , 2014, 3289 - 3293 xi represents multispectral (features) data. The algorithm is Euclidean) or similarity (e.g., correlation). Next, the an iterative optimization that minimizes the cost function algorithm merges the two nearest clusters and updates all defined as follows: the distances to the newly formed cluster via some linkage method, and this is repeated until there is only one cluster left that contains all the vectors. Three of the most common (2) ways to update the distances are with single, complete or where uij represents the membership of pixel in the average linkages. This process does not define a partition of the system, cluster, is the cluster center, is a norm metric, but a sequence of nested partitions, where each partition and m is a constant. The parameter m controls the fuzziness contains one less cluster than the previous partition. To of the resulting partition, and m=2 is used in this study. The obtain a partition with K clusters, the process must be cost function is minimized when pixels close to the centroid stopped K − 1 steps before the end. Different linkages lead of their clusters are assigned high membership values, and to different partitions, so the type of linkage used must be low membership values are assigned to pixels with data far selected according to the type of data to be clustered. For from the centroid. The membership function represents the instance, complete and average linkages tend to build probability that a pixel belongs to a specific cluster. compact clusters, while single linkage is capable of C. Expectation Maximization building clusters with more complex shapes but is more likely to be affected by spurious data [13]. Expectation Maximization(EM) is one of the most common algorithms used for density estimation of data E. Self organizing maps points in an unsupervised clustering. The algorithm relies The Self-Organizing Map (SOM) is a clustering on finding the maximum likelihood estimates of parameters algorithm that is used to map a multi-dimensional dataset when the data model depends on certain latent variables. In onto a (typically) two-dimensional surface.
Details
-
File Typepdf
-
Upload Time-
-
Content LanguagesEnglish
-
Upload UserAnonymous/Not logged-in
-
File Pages5 Page
-
File Size-