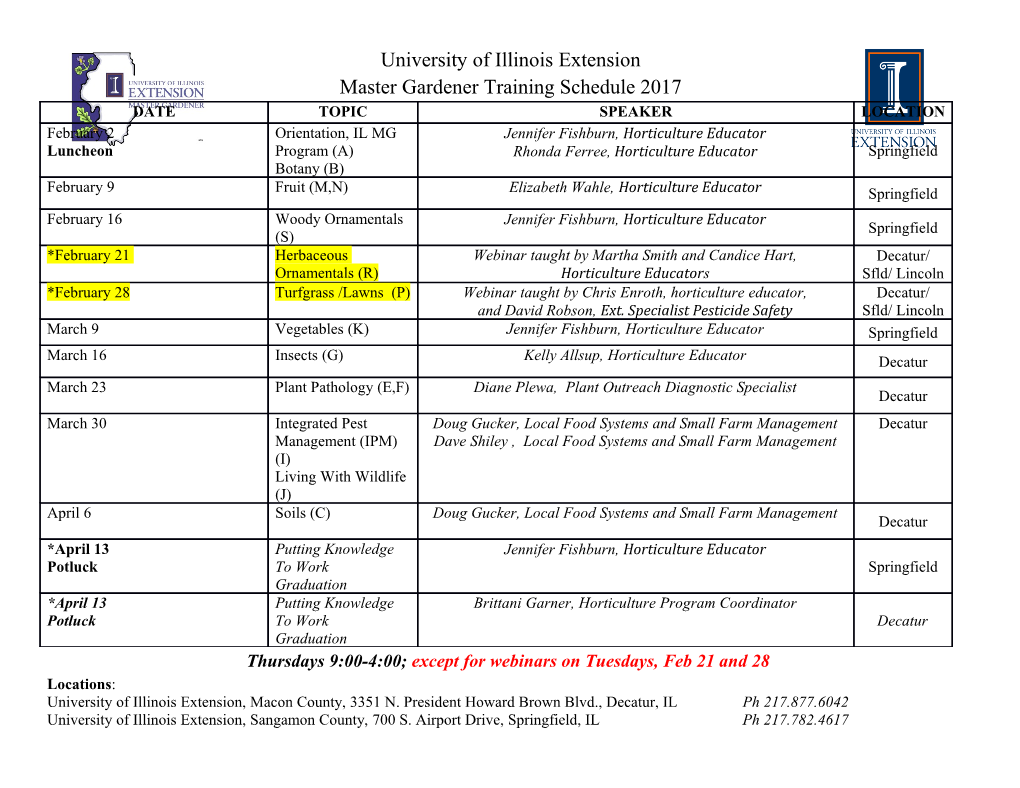
Towards Data Warehouse Quality Metrics Coral Calero Mario Piattini ALARCOS Research Group ALARCOS Research Group University of Castilla-La Mancha (Spain) University of Castilla-La Mancha (Spain) Rda. Calatrava s/n 13071 Ciudad Real - Spain Rda. Calatrava s/n 13071 Ciudad Real - Spain [email protected] [email protected] Carolina Pascual Manuel A. Serrano ALARCOS Research Group ALARCOS Research Group University of Castilla-La Mancha (Spain) University of Castilla-La Mancha (Spain) Rda. Calatrava s/n 13071 Ciudad Real - Spain Rda. Calatrava s/n 13071 Ciudad Real - Spain [email protected] [email protected] process” [INM96]. Data warehouses have become the key trend in corporate computing in the last years, since they Abstract provide managers with the most accurate and relevant information to improve strategic decisions. Jarke et al. Organizations are adopting datawarehouses to [JAR00] forecast a 12 Millions American dollars for the manage information efficiently as “the” main data warehouse market. organizational asset. It is essential that we can Different life cycles and techniques have been assure the information quality of the data proposed for data warehouse development [HAM96] warehouse, as it became the main tool for [KEL97] [KIM98]. However the development of a data strategic decisions. Information quality depends warehouse is a difficult and very risky task. It is essential on presentation quality and the data warehouse that we can assure the information quality of the data quality. This last includes the multidimensional warehouse as it became the main tool for strategic model quality. In the last years different authors decisions [ENG99]. have proposed some useful guidelines to design Information quality of a data warehouse comprise data multidimensional models, however more objective warehouse system quality and data presentation quality indicators are needed to help designers and (see figure 1). In fact, it is very important that data in the managers to develop quality datawarehouses. In data warehouse reflects correctly the real world, but it is this paper a first proposal of metrics for also very important that data can be easily understood. In multidimensional model quality is shown together data warehouse system quality, as in an operational with their formal validation. database [PIA00], three different aspects could be considered: DBMSs quality, data model quality (both 1 Introduction conceptual and logical) and data quality. In order to assess DBMS quality we can use an Nowadays organizations can store vast amounts of data international standard like [ISO98], or some of the existing obtained at a relatively low cost, however these data fail to product comparative studies. This type of quality should provide information [GAR98]. To solve this problem, be addressed in the product selection stage of the data organizations are adopting a data warehouse, which is warehouse life cycle. defined as a “collection of subject-oriented, integrated, Data quality is composed by the data definition quality non-volatile data that supports the management decision (degree to which data definition accurately describes the meaning of the real-world entity type or fact-type that the The copyright of this paper belongs to the paper’s authors. Permission to data represents. Also meets the needs of all information copy without fee all or part of this material is granted provided that the customers to understand the data they use), the data copies are not made or distributed for direct commercial advantage. content quality (degree to which data values accurately Proceedings of the International Workshop on Design and represent the characteristics of the real-world entity or fact Management of Data Warehouses (DMDW'2001) and meet the need of the information costumers to perform Interlaken, Switzerland, June 4, 2001 their jobs effectively) and the data presentation quality (D. Theodoratos, J. Hammer, M. Jeusfeld, M. Staudt, eds.) (try to capture the degree in which the format presented is intuitive for the use to be made of the information) http://sunsite.informatik.rwth-aachen.de/Publications/CEUR-WS/Vol-39/ C. Calero, M. Piattini, C. Pascual, M.A. Serrano 2-1 [ENG98]. This kind of quality must address mostly in the Metrics could be used to build prediction systems for extraction, filtering, cleaning and cleansing, database projects, to understand and improve software synchronization, aggregation, loading, etc. activities of the development and maintenance projects, to maintain the life cycle. In the last years very interesting techniques quality of the systems, highlighting problematic areas, and have been proposed to assure data quality [BOU00]. to determine the best ways to help practitioners and researchers in their work. The final goal of our work is to define a set of metrics for assuring data warehouse quality by means of INFORMATION QUALITY measuring the dimensional data model quality. In the next section we will present the method we use for defining correct metrics. A first proposal of metrics for data DATAWAREHOUSE PRESENTATION warehouses will be described in section 3 and an example QUALITY QUALITY of the proposed metrics will be shown in section 4. Section 5 will present the formal validation of the metrics and conclusions and future work will come in the last section. DBMS DATA MODEL DATA QUALITY QUALITY QUALITY 2 Defining Valid Metrics Metrics definition must be done in a methodological way and it is necessary to follow a number of steps to ensure DIMENSIONAL PHYSICAL the reliability of the proposed metrics. Figure 2 presents MODEL QUALITY MODEL QUALITY the method we follow for the metrics proposal [CAL01]. Figure 1 Information and data warehouse quality METRIC DEFINITION Last, but not least, data warehouse model quality has a great influence in the overall information quality. The designer has to choose the physical tables, processes, indexes and data partitions, representing the logical data warehouse and facilitating its functionality [JAR00]. Two EMPIRICAL VALIDATION different aspects should be considered: dimensional data THEORETICAL VALIDATION model quality and physical data model quality. In fact EXPERIMENTS CASE STUDIES these two are often considered as different stages in the data warehouse life cycle. Dimensional data model is usually designed using the star schema modeling facility, which allows good response Figure 2. Steps followed in the definition and validation of times and an easy understanding of data and metadata for metrics both users and developers [KIM98]. Different techniques have also been researched for In this figure we have three main activities: optimizing physical data models [HAR96] [LAB97]. Our work focuses on dimensional data model quality. · Metrics definition. The first step is the proposal Different authors have suggested interesting of metrics. This definition is made taking into recommendations for achieving a “good” dimensional data account the specific characteristics of the system model [KIM98] [ADM98] [INM96]. However quality we want to measure and the experience of criteria are not enough on their own to ensure quality in designers of these systems. A goal-oriented practice, because different people will generally have approach, as GQM (Goal-Question-Metric) different interpretations of the same concept. According [BAS84] can also be very useful in this step. to the Total Quality Management (TQM) literature, · Theoretical validation. The second step is the measurable criteria for assessing quality are necessary to formal validation of the metrics. The formal avoid “arguments of style” [BOM97]. The objective validation helps us to know when and how apply should be to replace intuitive notions of design “quality” the metrics. There are two main tendencies in with formal, quantitative measures in order to reduce metrics validation: the frameworks based on subjectivity and bias in the evaluation process. However, axiomatic approaches [WEY88] [BRI96] [MOR97] for data modeling to progress from a “craft” to an and the ones based on the measurement theory engineering discipline, the desirable qualities of data [WIT97] [ZUS98]. The strength of measurement models need to be made explicit [LIN94]. A metric is away theory is the formulation of empirical conditions of measuring a quality factor in a consistent and objective from which we can derive hypothesis of reality. manner The final information when applying this kind of C. Calero, M. Piattini, C. Pascual, M.A. Serrano 2-2 frameworks in to know to which scale a metric NAFT(S) = NA(FT) + NFK(FT) pertains and based on this information we can Where FT is the fact table of the star S. know which statistics and which transformations can be done with the metric. · NA(S). Number of attributes of a star. · Empirical validation. The goal of this step is to prove the practical utility of the proposed NA(S) = NAFT(FT) + NADT(S) metrics. Although there are various ways of Where FT is the fact table of the star S. performing this step, basically we can divide the empirical validation into experimentation and case · NFK(S). Number of foreign keys of a star. studies [BAS99] [FEN97]. NDT As shown in figure 2, the process of defining and NFK(S) = NFK(FT) + å NFK(DTi) validating metrics is evolutionary and iterative. As a result i=1 of the feedback, metrics could be redefined based on Where FT is the fact table of the star S discarded theoretical or empirical validations. At the and Dti is the dimensional table number i of the star S moment, we have finished only a first iteration of metrics definition and theoretical validation for data warehouse · RSA(S). Ratio of star attributes. Quantity of the quality. number of attributes of dimension tables per This work only considers the two steps related with number of attributes of the fact table of the star. definition and formal validation. It is clear that these are only a first approach because it is fundamental to made NADT (S) empirical validation in order to prove which of all of the RSA(S) = proposed metrics are useful in the real world rejecting the NAFT(FT) ones that do not prove its uselfulness.
Details
-
File Typepdf
-
Upload Time-
-
Content LanguagesEnglish
-
Upload UserAnonymous/Not logged-in
-
File Pages10 Page
-
File Size-