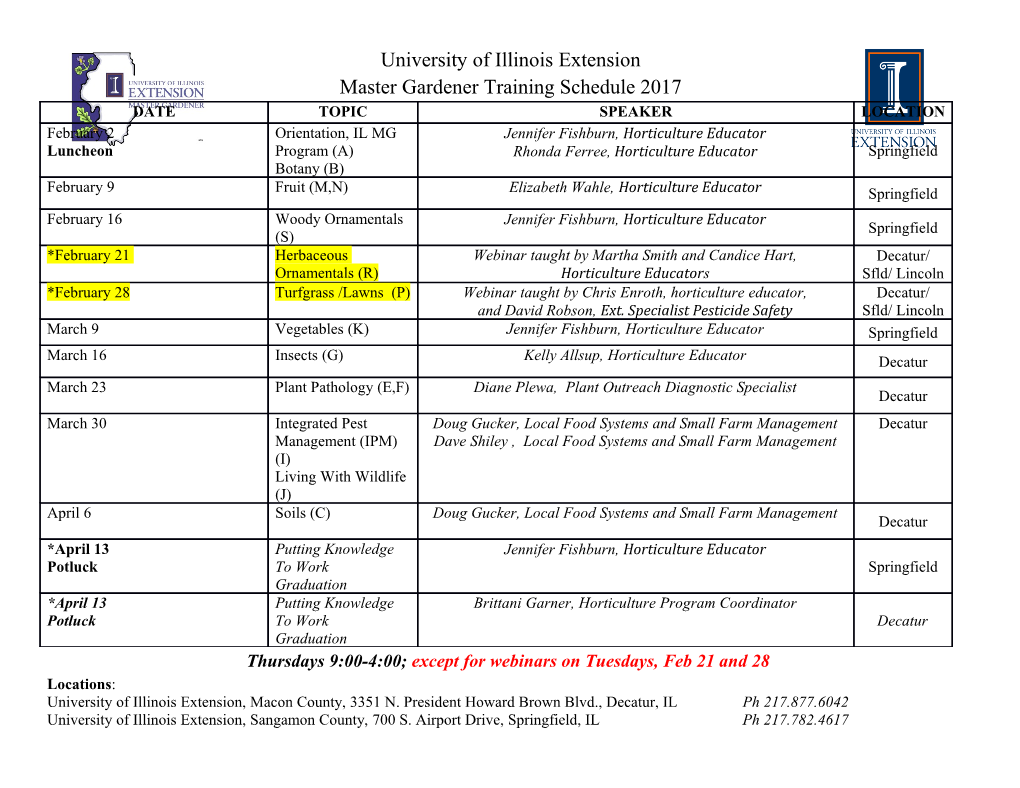
43 INFORMATION CONTENT AND ERROR ANALYSIS Clive D Rodgers Atmospheric, Oceanic and Planetary Physics University of Oxford ESA Advanced Atmospheric Training Course September 15th – 20th, 2008 44 INFORMATION CONTENT OF A MEASUREMENT Information in a general qualitative sense: Conceptually, what does y tell you about x? We need to answer this to determine if a conceptual instrument design actually works • to optimise designs • Use the linear problem for simplicity to illustrate the ideas. y = Kx + ! 45 SHANNON INFORMATION The Shannon information content of a measurement of x is the change in the entropy of the • probability density function describing our knowledge of x. Entropy is defined by: • S P = P (x) log(P (x)/M (x))dx { } − Z M(x) is a measure function. We will take it to be constant. Compare this with the statistical mechanics definition of entropy: • S = k p ln p − i i i X P (x)dx corresponds to pi. 1/M (x) is a kind of scale for dx. The Shannon information content of a measurement is the change in entropy between the p.d.f. • before, P (x), and the p.d.f. after, P (x y), the measurement: | H = S P (x) S P (x y) { }− { | } What does this all mean? 46 ENTROPY OF A BOXCAR PDF Consider a uniform p.d.f in one dimension, constant in (0,a): P (x)=1/a 0 <x<a and zero outside.The entropy is given by a 1 1 S = ln dx = ln a − a a Z0 „ « Similarly, the entropy of any constant pdf in a finite volume V of arbitrary shape is: 1 1 S = ln dv = ln V − V V ZV „ « i.e the entropy is the log of the volume of state space occupied by the p.d.f. 47 ENTROPY OF A GAUSSIAN PDF Consider the Gaussian distribution: 1 1 T 1 − P (x)= n 1 exp[ (x x¯) S (x x¯)] (2π) 2 S 2 −2 − − | | 1 If you evaluate the entropy of a Gaussian distribution you will find it is proportional to log S 2 . | | The contours of P (x) in n-space are ellipsoidal, described by • T 1 (x x¯) S− (x x¯) = constant − − The principal axes of the ellipsoid are the eigenvectors of S, and their lengths are proportional • to the square roots of the corresponding eigenvalues. The volume of the ellipsoid is proportional to the root of the product of the eigenvalues, which • 1 is proportional to S 2 . | | Therefore entropy is the log of the volume enclosed by some particular contour of P (x).A • ‘volume of uncertainty’. 48 ENTROPY AND INFORMATION Information content is the change in entropy, i.e. the log of the ratio of the volumes of uncertainty before and after making a measurement. • A generalisation of ‘signal to noise’. • In the Boxcar case, the log of the ratio of the volumes before and after • In the Gaussian case: • T 1 1 1 1 H = log S log Sˆ = log (K S− K + S− )− S− | a|− | | − | " a a | minus the log of the determinant of the weight of x in the Bayesian expectation. • a 49 The log of the ratio of the volumes of the ellipsoids 50 DEGREES OF FREEDOM FOR SIGNAL AND NOISE The state estimate that maximises P (x y) in the linear Gaussian case is the one which minimises | 2 T 1 T 1 χ =[y Kx] S− [y Kx]+[x x ] S− [x x ] − " − − a a − a The r.h.s. has initially m + n degrees of freedom, of which n are fixed by choosing x to be xˆ, so the expected value of χ2 is m. These m degrees of freedom can be assigned to degrees of freedom for noise dn and degrees of freedom for signal ds according to: T 1 d = E [y Kxˆ] S− [y Kxˆ] n { − " − } and T 1 d = E [xˆ x ] S− [xˆ x ] s { − a a − a } Using tr(CD)=tr(DC), we can see that T 1 ds = E tr([xˆ xa][xˆ xa] Sa− ) { − − T 1} = tr(E [xˆ x ][xˆ x ] S− ) (6) { − a − a } a 51 DEGREES OF FREEDOM FOR SIGNAL AND NOISE II With some manipulation we can find T 1 1 1 T 1 ds = tr((K S"− K + Sa− )− K S"− K) T T 1 = tr(KSaK (KSaK + S")− ) (7) and T 1 1 1 1 dn = tr((K S"− K + Sa− )− Sa− )+m n T 1 − = tr(S"(KSaK + S")− ) (8) 52 INDEPENDENT MEASUREMENTS If the measurement error covariance is not diagonal, the elements of the y vector will not be statistically independent. Likewise for the a priori. The measurements will not be independent functions of the state if K is not diagonal. Therefore it helps to understand where the information comes from if we transform to a different basis. First, statistical independence. Define: 1 1 2 2 y˜ = S"− y x˜ = Sa− x The transformed covariances S˜a and S˜" both become unit matrices. [>>] 53 SQUARE ROOTS OF MATRICES 1 The square root of an arbitrary matrix is defined as A2 where 1 1 A2 A2 = A Using An = RΛnLT for n =1/2: 1 1 A2 = RΛ2 LT 1 1 This square root of a matrix is not unique, because the diagonal elements of Λ2 in RΛ2 LT can have either sign, leading to 2n possibilities. 1 1 We only use square roots of symmetric covariance matrices. In this case S2 = LΛ2 LT is symmetric. 54 SQUARE ROOTS OF SYMMETRIC MATRICES 1 1 Symmetric matrices can also have non-symmetric roots satisfying S =(S2 )T S2 , of which the Cholesky decomposition: S = TT T where T is upper triangular, is the most useful. 1 There are an infinite number of non-symmetric square roots: if S2 is a square root, then clearly so 1 is XS2 where X is any orthonormal matrix. 1 1 T The inverse symmetric square root is S−2 = LΛ−2 L . 1 1 T The inverse Cholesky decomposition is S− = T− T− . 1 The inverse square root T− is triangular, and its numerical effect is implemented efficiently by back substitution. 55 INDEPENDENT MEASUREMENTS[<<] If the measurement error covariance is not diagonal, the elements of the y vector will not be statistically independent. Likewise for the a priori. The measurements will not be independent functions of the state if K is not diagonal. Therefore it helps to understand where the information comes from if we transform to a different basis. First, statistical independence. Define: 1 1 2 2 y˜ = S"− y x˜ = Sa− x The transformed covariances S˜a and S˜" both become unit matrices. The forward model becomes: y˜ = K˜ x˜ + ˜! 1 1 2 2 where K˜ = S"− KSa . 56 INDEPENDENT MEASUREMENTS[<<] If the measurement error covariance is not diagonal, the elements of the y vector will not be statistically independent. Likewise for the a priori. The measurements will not be independent functions of the state if K is not diagonal. Therefore it helps to understand where the information comes from if we transform to a different basis. First, statistical independence. Define: 1 1 2 2 y˜ = S"− y x˜ = Sa− x The transformed covariances S˜a and S˜" both become unit matrices. The forward model becomes: y˜ = K˜ x˜ + ˜! 1 1 2 2 where K˜ = S"− KSa . The solution covariance becomes: ˆ T 1 S˜ =(In + K˜ K˜ )− 57 TRANSFORM AGAIN Now make K˜ diagonal. Rotate both x and y to yet another basis, defined by the singular vectors of K˜ : y˜ = K˜ x˜ + ˜! y˜ = UΛVT x˜ + ˜! → Define: T T T x# = V xy˜ # = U y˜ !# = U ˜! The forward model becomes: y# = Λx# + !# (1) The Jacobian is now diagonal, Λ, and the a priori and noise covariances are still unit matrices, hence the solution covariance becomes: ˆ T 1 2 1 S˜ =(I + K˜ K˜ )− Sˆ# =(I + Λ )− n → n which is diagonal, and the solution itself is 2 1 xˆ# =(In + Λ )− (Λy# + xa# ) 1 not xˆ# = Λ− y# as you might expect from (1). 2 1 Elements for which λi 1 or (1 + λi )− 1 are well measured • $ 2 1 % Elements for which λ 1 or (1 + λ )− 1 are poorly measured. • i % i $ 58 INFORMATION Shannon Information in the Transformed Basis Because it is a ratio of volumes, the linear transformation does not change the information content. So consider information in the x#, y# system: H = S S# S Sˆ# { a}− { } 1 1 2 1 = log( In )+ log( (Λ + I)− ) −2 | | 2 | | 1 = log(1 + λ2) (9) 2 i i X 59 DEGREES OF FREEDOM Degres of Freedom in the Transformed Basis The number of independent quantities measured can be thought of as the number of singular values for which λ 1 i $ The degrees of freedom for signal is 2 2 1 ds = λi (1 + λi )− i X It is also the sum of the eigenvalues of I S˜ˆ. n − Summary: for each independent component xi# 1 2 The information content is 2 log(1 + λi ) • 2 2 1 The degrees of freedom for signal is λ (1 + λ )− • i i 60 THE OBSERVABLE AND NULL SPACES The part of measurement space that can be seen is that spanned by the weighting functions. • Anything outside that is in the null space of K. Any orthogonal linear combination of the weighting functions will form a basis (coordinate • system) for the observable space. An example is those singular vectors of K which have non-zero singular values. The vectors which have zero singular values form a basis for the null space.
Details
-
File Typepdf
-
Upload Time-
-
Content LanguagesEnglish
-
Upload UserAnonymous/Not logged-in
-
File Pages48 Page
-
File Size-