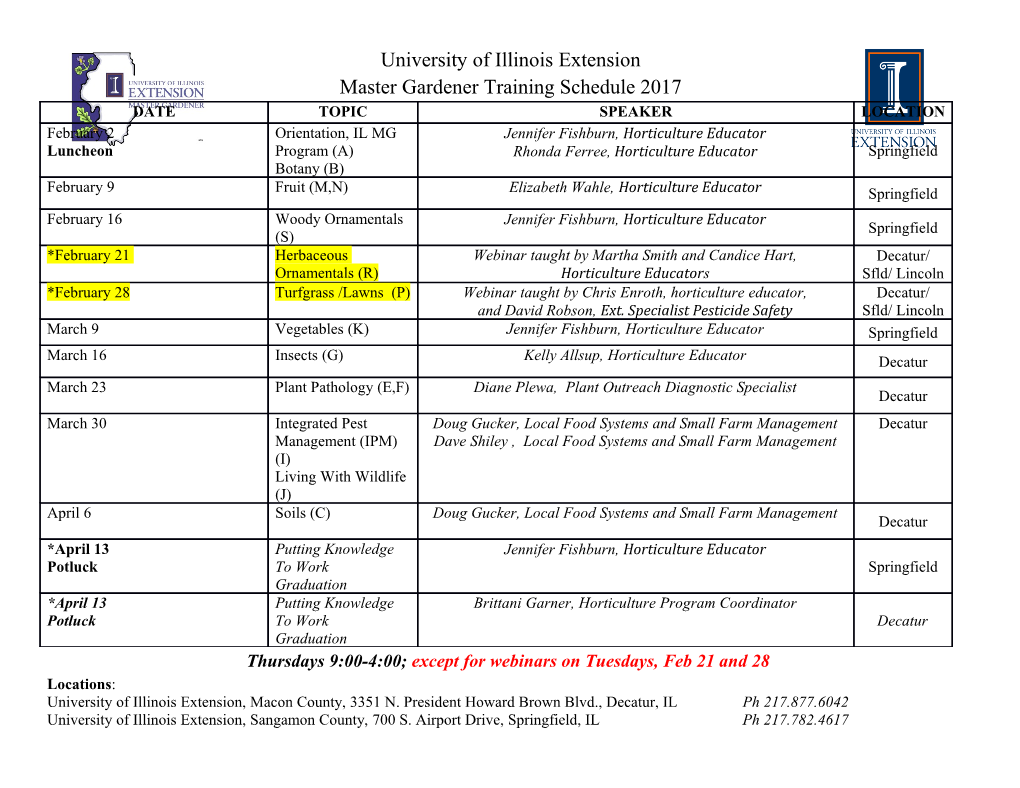
AXIOMS OF PROBABILITY OCT 1, 2018 Sets and operations on sets. Appendix B Review Venn diagrams and set operations: (i) union A [ B, (ii) intersection A \ B (also written as AB), (iii) complement Ac, (iv) difference AnB. Problem. Let A; B; C be subsets of Ω. Represent the following in set notation. (1) Set of elements that are in each of the three subsets = A \ B \ C. (2) Set of elements that are in A but neither in B nor in C = A \ Bc \ Cc. (3) Set of elements that are in at least one of the sets A or B = A [ B. (4) Set of elements that re in both A and B, but not in C = A \ B \ Cc. (5) Set of elements that are in A, but not in B or C or both = An(B [ C). Axiomatic definition of a probability measure. Let Ω (pronounced `omega') be a set called sample space. Elements of Ω are called outcomes while subsets of Ω are called events. Let F be the power set (i.e., set of all subsets of Ω) of Ω. Then a probability, or probability measure, is a function from F to [0; 1] satisfying the following conditions: • P (Ω) = 1. P (;) = 0. • Countable additivity: If A1;A2;::: is a sequence of pairwise disjoint (i.e. Ai \ Aj = ; for all i 6= j) events then 1 1 X P ([i=1Ai) = P (Ai): i=1 In other words, with every event E ⊆ Ω, a probability measure associates a number 0 ≤ P (E) ≤ 1 which is the probability that we observe the event E happening. The triplet (Ω; F;P ) is called a probability space. Remark 1. More generally F is taken to be a σ-field (pronounced ‘sigma-field’), a concept from measure theory. But you don't need to know that right now. Some consequences of the definition. • Finite additivity: If A1;:::;Ak is a finite pairwise disjoint collection of events, then k P [i=1Ai = P (A1) + ::: + P (Ak): This follows from countable additivity by taking Ak+1 = Ak+2 = ::: = ;. • Complements: If A is an event, its complement Ac is the event Ac = ΩnA. Clearly A and Ac are disjoint. Thus, by finite additivity, P (A) + P (Ac) = P (A \ Ac) = P (Ω) = 1. Thus P (Ac) = 1 − P (A): Equally likely outcomes. Suppose the sample space Ω is finite and has N ele- ments f!1;:::;!N g. Say that all outcomes are equally likely if P (!i) := P (f!ig) = 1=N for all i. In that case, it is very easy to find the probability measure. If E is 1 2 an event, then E is a subset of Ω. If #E is the number of elements in E then, by finite additivity, #E P (E) = : N Check that obviously P (Ω) = 1 and P (;) = 0. Example 1. Toss a coin twice. Then Ω = fHH;TT;HT;THg, and 1 P fHHg = P fTT g = P fTHg = P fHT g = : 4 For example, the event A = fHH; T T g, i.e., the event that both tosses are the same, has probability P (A) = 1=2, under the assumption of equally likely outcomes. Example 2. Roll a die twice. Here Ω is the set of 36 possible outcomes f(1; 1); (1; 2); (1; 3);::: (6; 5); (6; 6)g: Let E be the event that the sum of the two rolls is 5. Then E consists of 4 sample points: (1; 4); (2; 3); (3; 2); (4; 1). Hence P (E) = 4=36 = 1=9, under the assumption of equally likely outcomes. Example 3. Sampling with replacement. Imagine an urn with N ≥ 2 balls la- beled by f1; 2;:::;Ng. Pick one ball without looking, note its label, and put it back. Repeat this a total of k times. The k labels picked form a k-tuple (a1; a2; : : : ; ak). This is one random outcome. The sample space Ω is the set of all k-tuples with entries in f1; 2;:::;Ng. Clearly Ω is finite and has N k elements. We say that the sampling is random, or, more correctly uniformly at random, if every outcome in Ω is equally likely. Hence the probability of every tuple is exactly 1=N k, under the assumption of equally likely outcomes. What is the probability that every label sampled is either 1 or 2? Here the event k E := f(!1;:::;!k): !i is 1 or 2 for each ig. Clearly there are #E = 2 . Hence P (E) = 2k=N k = (2=N)k, under the assumption of equally likely outcomes. Example 4. Ordered sampling without replacement. In sampling without replacement, we still have an urn with N ≥ 2 balls labeled by f1; 2;:::;Ng. We pick balls successively and note its label. However, we do not put it back. Hence Ω is the set of arrangements of k labels taken from f1; 2;:::;Ng. Thus #Ω = (n)k. A random ordered sample without replacement means each of these (n)k outcomes are equally likely. What is the probability that every label sampled is either 1 or 2? It is zero if k > 2, since after the first two labels are 1 or 2, the rest cannot be either. Example 5. (Unordered) sampling without replacement. Take an urn with N ≥ 2 balls labeled by f1; 2;:::;Ng. By a random sample without replace- n ment we mean that pick any subset of k balls equally likely. Thus #Ω = k ..
Details
-
File Typepdf
-
Upload Time-
-
Content LanguagesEnglish
-
Upload UserAnonymous/Not logged-in
-
File Pages2 Page
-
File Size-