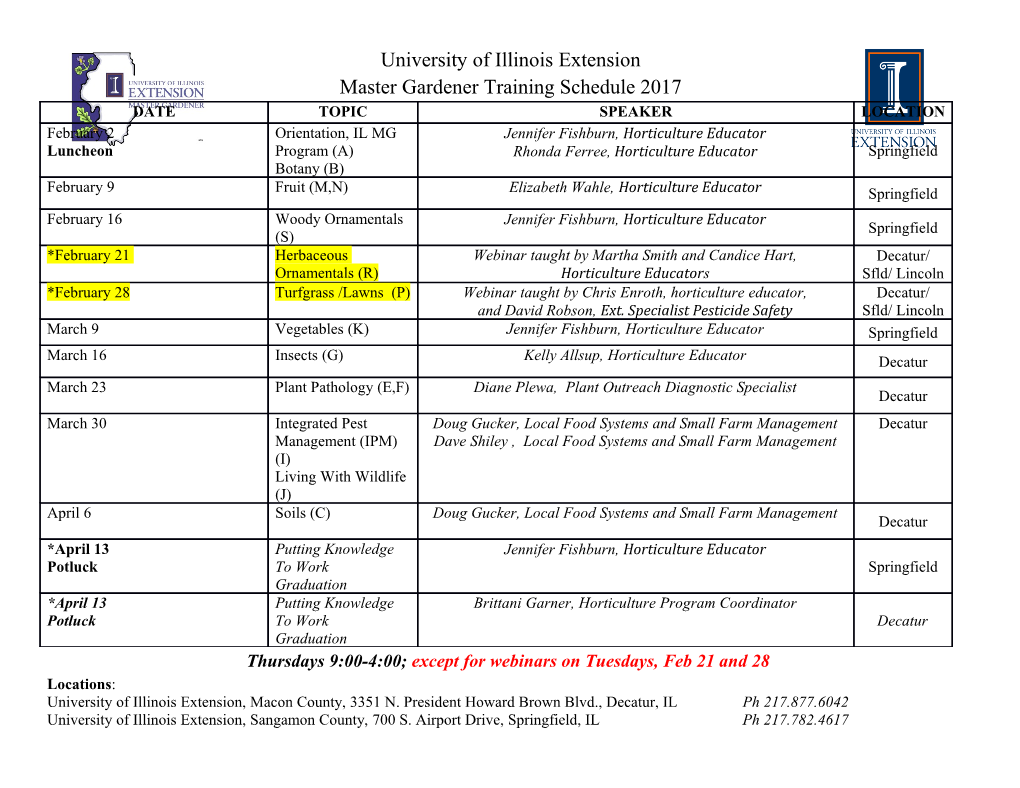
Information Geometry: A Geometry-free Introduction Shane Gu, Nilesh Tripuraneni [email protected] April 2, 2015 Outline Maximum Entropy Principle Exponential Families Information Projection Fisher Information Reference 2 of 21 Motivating Example Problem: model the distribution of English words (in a corpus, etc.) Domain: S =fEnglish wordsg If no data is observed, a sensible default is uniform over S If some data is observed, and some simple statistics are collected, e.g.: Pr(length > 5) = 0.3 Pr(end in ’e’) = 0.45 Pr(start with ’s’) = 0.08 then, what should the distribution be? Intuition: choose a distribution as random as possible but satisfies the above statistics 3 of 21 Entropy Entropy: a measure of randomness, or “unpredictability of information content” Given X has distribution p(x), entropy is:H(X) = − P p(x)log p(x) Nice properties: I Expandibility if X has distribution p1; p2; :::; pn, and Y has distribution fp1; p2; :::; pn; 0g, H(X) = H(Y ) I Symmetry e.g. fp; 1 − pg has same entropy as 1 − p; p I Additivity if X and Y and independent, H(X; Y ) = H(X) + H(Y ) I Subadditivity H(X; Y ) ≤ H(X) + H(Y ) I Normalization A fair coin has 1 bit entropy I “Small for small probability” Entropy of a coin with bias p goes to 0 as p goes to 0 H(X) is the only measure that satisfies the above six properties [Aczel´ et al., 1974] 4 of 21 Maximum Entropy Principle Back to the problem, given features Ti (x), e.g. 1(length > 5), we collect statistics ETi (x) = bi P max H(p) = − x p(x)log p(x) P x p(x)Ti (x) = bi ; i = 1:::k p(x) ≥ 0; x 2 S P x p(x) = 1 I A convex optimization problem with linear constraints! I Finds a distribution that satisfies the statistics and is as random as possible, i.e. as close to uniform as possible I What if our prior is not uniform? 5 of 21 KL Divergence Kullback-Leibler divergence (relative entropy): a distance measure between two distributions, p; q. P p(x) P KL(pjjq) = x p(x)log q(x) = −H(p) + x p(x)log q(x) Properties: I KL(pjjq) ≥ 0, with equality if p = q I KL(pjjq) could be infinite I KL(pjjq) is not KL(qjjp) 6 of 21 MaxEnt (alternate formulation) Given a prior π P p(x) min KL(pjjπ) = x p(x)log π(x) P x p(x)Ti (x) = bi ; i = 1:::k p(x) ≥ 0; x 2 S P x p(x) = 1 I Finds a distribution that satisfies the statistics and is as close to the prior π as possible 7 of 21 MaxEnt: solution Using Lagrange multipliers: F(p; λ, ν) = P p(x) P P P x p(x)log π(x) + i λi ( x p(x)Ti (x) − bi ) + ν( x p(x) − 1) @F p P @p = 1 + log π + i λi Ti − ν = 0 P 1 i λi Ti (x) Solution: p(x) = Z e π(x) (p(x) ≥ 0; x 2 S is automatically satisfied) I the exponential family generated by π D I input space: S 2 R D I base measure π : R −! R I features Ti (x); i = 1; :::; k 8 of 21 Exponential families Given: D I input space: S 2 R D I base measure π : R −! R I features T (x) = T1(x); :::; Tk (x) Exponential family generated by T and π consists of log linear models parametrized by natural parameters η 2 Rk : η·T (x) pη(x) / e π(x) η·T (x)−G(η) pη(x) = e π(x) P η·T (x) Log partition function: G(η) = log x e π(x) Natural parameter space: N = fη 2 Rk : − inf < G(η) < infg 9 of 21 Properties of exponential families P η·T (x) recall log partition function: G(η) = log x e π(x) P η·T (x) 0 x e π(x)T (x) I G (η) = P η·T (x) = ET (x) x e π(x) 00 I G (η) = varT (x) > 0, etc. 0 I G(η) is strictly concave ! G (η) is 1-to-1 I η $ ET (x) has one-to-one mapping (natural paramters $ expectation parameters) Maximum likelihood estimation: I observed data: xi ; i = 1; :::; m 1 P 1 P I L = m i logp(x) = m i (η · T (x) − G(η) + logπ(x)) @L 1 P 0 I @η = m i T (x) − G (η) = Ex∼pdata T (x) − Ex∼pT (x) 0 1 I set derivative to zero: G (η) = m T (x) I MLE is to find η s.t. Ex∼pT (x) = Ex∼pdata T (x) 10 of 21 Example: Univariate Gaussian (x−µ)2 1 − p(x; µ, σ) = p e 2σ2 2πσ2 1 (x;x2)·(µ/σ2;−1=2σ2)−(µ2=2σ2+logσ) I p(x; µ, σ) = p e 2π p I S = R, base measure π(x) = 1= 2π 2 2 2 I T (x) = (x; x ); η = (µ/σ ; −1=2σ ) 2 2 2 η 1 1 I G(η) = µ =2σ + logσ = − 1 + logj j 4η2 2 2η2 − I η 2 R × R 11 of 21 Example: RBM Restricted Boltzmann Machine, more generally, Exponential Family Harmoniums [Welling et al., 2004] I observed variables X = xi ; i = 1; :::; nx , latent variables V = vj ; j = 1; :::; nv P b ·f (x )+P b ·g (v )+P f (x )T ·w ·g (v ) I p(X; V ) / e i x;i i i j v;j j j i;j i i ij j j I p(XjV ); P(V jX) factorizes over p(xi jV ); p(vj jX) respectively P b ·f (x )+P G ·(b +P f (x )T ·w ) I p(X) / e i x;i i i j v;j v;j i i i ij I η = ffbx;i g; fbv;j g; fwij gg Contrastive Divergence: @L I /< f (x ) >p − < f (x ) >p @bx;i i i data i i I CD is gradient ascent in natural parameter space wrt MLE objective 12 of 21 MaxEnt restatement Given: D I input space: S 2 R D I base measure π : R −! R I features T (x) = T1(x); :::; Tk (x) I constraints ET (x) = b If there’s a distribution of the form p∗(x) = eη·T (x)−G(η)π(x) satisfying the constraints, it is the unique minimizer of KL(p; π) subject to these constraints. 13 of 21 Proof consider any other distribution p satisfying EpT (x) = b X p(x) X p∗(x) KL(p; π) − KL(p∗; π) = p(x)log − p∗(x)log π(x) π(x) x x X p(x) X = p(x)log − p∗(x)(η · T (x) − G(η)) π(x) x x X p(x) X = p(x)log − p(x)(η · T (x) − G(η)) π(x) x x X p(x) X p∗(x) = p(x)log − p(x)log π(x) π(x) x x X p(x) = p(x)log = KL(p; p∗) > 0 p∗(x) x (1) KL(p; π) = KL(p; p∗) + KL(p∗; π) 14 of 21 Geometry http://videolectures.net/mlss05us_dasgupta_ig/ 15 of 21 More geometry http://videolectures.net/mlss05us_dasgupta_ig/ 16 of 21 Fisher Information Metric Given pθ(x), Fisher information matrix Iθ is defined as: T _ _T Iθ := Eθ[rθlog pθ(x)rθlog pθ(x) ] = Eθ[`θ`θ ] Fisher score function: `_θ = rθlog pθ(x) _ Eθ[`θ] = 0 Relation to Hessian of log pθ(x): r2p (x) rp (x)rp (x)T r2p (x) 2 θ θ θ θ _ _T r log pθ(x) = − 2 = − `θ` pθ(x) pθ(x) pθ(x) θ R 2 R 2 2 Iθ = − pθ(x)r log pθ(x)dx + r pθ(x)dx = −Eθ[r log pθ(x)] Relation to KL Divergence: 2 0 2 0 Iθ = rθ0 KL(θ; θ )jθ0=θ = rθ0 KL(θ ; θ)jθ0=θ Fisher information depends on parametrization θ! 17 of 21 Fisher Informaton and KL For any appropriately smooth family of distributions fpθgθ2Θ: 1 KL(pθ1 ; pθ2 ) ≈ 2 < θ1 − θ2; Iθ1 (θ1 − θ2) > if θ1 ≈ θ2 Proof:By Taylor expansion of log pθ2 (x) around θ1: 1 log pθ2 (x) = log pθ1 (x)+ < rlog pθ1 (x); θ1 − θ2 > + 2 < 2 θ1 − θ2; r log pθ(x)(θ1 − θ2) > +R(θ1; θ2; x) 3 R(θ1; θ2; x) = O(kθ1 − θ2k ) is the remainder term. Then: 1 Eθ1 [log pθ2 (x)] ≈ Eθ1 [log pθ1 (x)] − 2 < θ1 − θ2; Iθ1 (θ1 − θ2) > 1 T KL(pθ1 ; pθ2 ) ≈ 2 (θ1 − θ2) Iθ1 (θ1 − θ2) 1 2 I η − η0 = Iθ (θ − θ0) 1 2 I KL(pη; pη0 ) ≈ KL(pη0 ; pη) ≈ 2 kη − η0k I local properties no longer depend on parametrization 18 of 21 Natural gradient gradient: I the gradient = the direction with the highest increase in the objective per step length rf (θ) I arg max f (θ + δθ) = krf (θ)k2 δθ:kδθk2= I the state after the gradient step depends on parametrization natural gradient I the gradient = the direction with the highest increase in the objective per change in KL I T arg max f (θ + δθ) = arg max f (θ) + rθf (θ) δθ δθ:KL(θ,θ+δθ)= 1 T δθ: 2 δθ Iθδθ= −1 / Iθ rθf (θ) (2) I does not depend on parametrization 19 of 21 I; KL, and exponential families η·T (x)−G(η) Given pη(x) = e π(x) _ 0 `η = rη(η ·T (x)−G(η)+logπ(x)) = T (x)−G (η) = T (x)−Eη[T (x)] 00 Iη = Varη[T (x)] = G (η) @θ Let θ = EηT (x), we know Iη = @η : @θ X @pη(x) i = T (x) @η @η i j x j X = pη(x)(Tj (x) − Eη[Tj (x)])Ti (x) (3) x = Eη[Ti (x)Tj (x)] − Eη[Ti (x)]Eη[Tj (x)] = Iη;ij Since natural gradient for η; θ are respectively: −1 @f −1 @θ @f @f @f g(η) = Iη @η = Iη @η @θ = @θ and similarly, g(θ) = @η −! gradient in one parameter space gives natural gradient in the other [Hensman et al., 2012] 20 of 21 Reference (1) http://videolectures.net/mlss05us_dasgupta_ig/ https://hips.seas.harvard.edu/blog/2013/04/08/ fisher-information/ https://web.stanford.edu/class/stats311/Lectures/ lec-09.pdf http://www.cs.berkeley.edu/˜pabbeel/cs287-fa09/ lecture-notes/lecture20-6pp.pdf 21 of 21 Aczel,´ J., Forte, B., and Ng, C.
Details
-
File Typepdf
-
Upload Time-
-
Content LanguagesEnglish
-
Upload UserAnonymous/Not logged-in
-
File Pages22 Page
-
File Size-