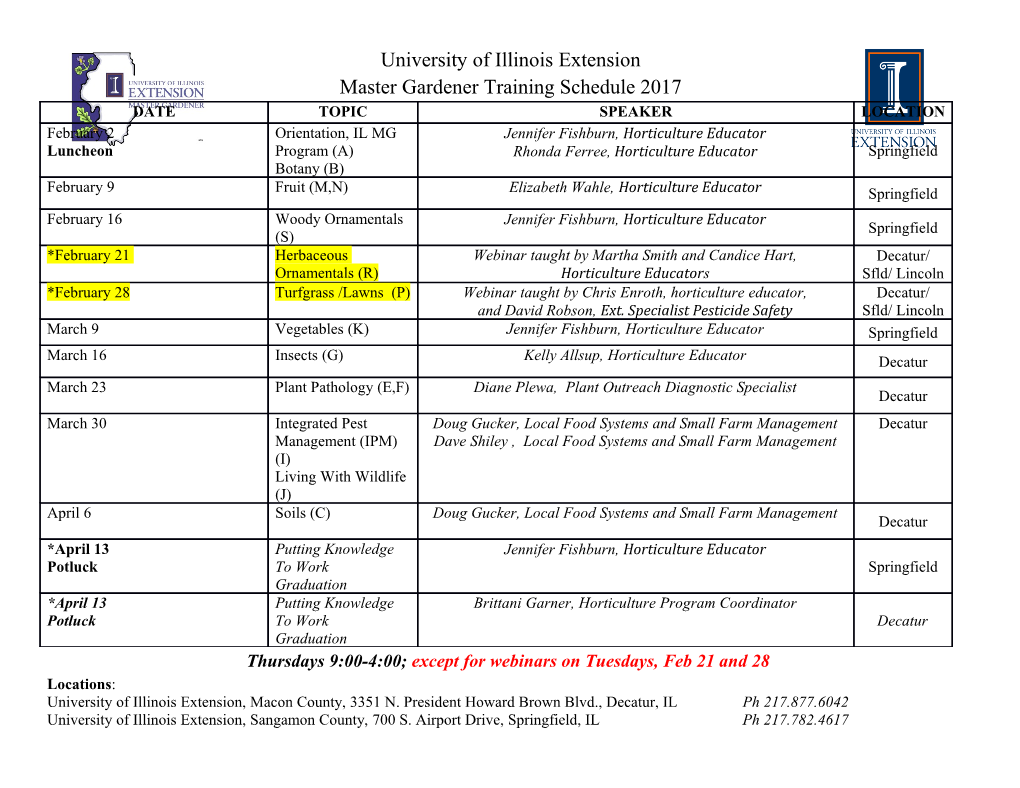
Bandit Processes and Dynamic Allocation Indices Author(s): J. C. Gittins Source: Journal of the Royal Statistical Society. Series B (Methodological), Vol. 41, No. 2 (1979), pp. 148-177 Published by: Blackwell Publishing for the Royal Statistical Society Stable URL: http://www.jstor.org/stable/2985029 Accessed: 10/12/2010 20:37 Your use of the JSTOR archive indicates your acceptance of JSTOR's Terms and Conditions of Use, available at http://www.jstor.org/page/info/about/policies/terms.jsp. JSTOR's Terms and Conditions of Use provides, in part, that unless you have obtained prior permission, you may not download an entire issue of a journal or multiple copies of articles, and you may use content in the JSTOR archive only for your personal, non-commercial use. Please contact the publisher regarding any further use of this work. Publisher contact information may be obtained at http://www.jstor.org/action/showPublisher?publisherCode=black. Each copy of any part of a JSTOR transmission must contain the same copyright notice that appears on the screen or printed page of such transmission. JSTOR is a not-for-profit service that helps scholars, researchers, and students discover, use, and build upon a wide range of content in a trusted digital archive. We use information technology and tools to increase productivity and facilitate new forms of scholarship. For more information about JSTOR, please contact [email protected]. Royal Statistical Society and Blackwell Publishing are collaborating with JSTOR to digitize, preserve and extend access to Journal of the Royal Statistical Society. Series B (Methodological). http://www.jstor.org J. R. Statist.Soc. B (1979), 41, No. 2, pp. 148-177 Bandit Processes and Dynamic Allocation Indices By J. C. GITTINS Keble College,Oxford [Read beforethe ROYAL STATISTICAL SOCIETY at a meetingorganized by theRESEARCH SECTION on Wednesday,February 14th, 1979, the Chairman Professor J. F. C. KINGMAN in theChair] SUMMARY The paperaims to givea unifiedaccount of thecentral concepts in recentwork on banditprocesses and dynamicallocation indices; to show how thesereduce some previouslyintractable problems to the problemof calculatingsuch indices;and to describehow these calculationsmay be carriedout. Applicationsto stochastic scheduling,sequential clinical trials and a class of searchproblems are discussed. Keywords:BANDIT PROCESSES;DYNAMIC ALLOCATION INDICES; TWO-ARMEDBANDIT PROBLEM; MARKOVDECISION PROCESSES; OPTIMAL RESOURCE ALLOCATION; SEQUENTIAL RANDOM SAMPLING;CHEMICAL RESEARCH; CLINICAL TRIALS; SEARCH 1. INTRODUCTION A schedulingproblem There are n jobs to be carriedout by a singlemachine. The timestaken to processthe jobs are independentinteger-valued random variables. The jobs mustbe processedone at a time. At the beginningof each timeunit any job may be selectedfor processing, whether or not the job processedduring the precedingtime unit has been completed,and thereis no penaltyor delayinvolved in switchingfrom one job to another.The probabilitythat t + 1 time unitsare requiredto completethe processingof job i, conditionalon more than t timeunits being needed, is pi(t) (i = 1,2, ..., n; te Z). The rewardfor finishingjob i at times is as Vi (O< a <1; ViJ>>0,i = 1,2, ..., n), and there are no other rewards or costs. The problem is to decide whichjob to processnext at each stage so as to maximizethe total expectedreward. A multi-armedbandit problem There are n armswhich may be pulled repeatedlyin any order. Each pull takes one time unit and only one arm may be pulled at a time. A pull may result in either a success or a failure. The sequence of successes and failures which result from pulling arm i forms a Bernoulli process with an unknown success probability 6i (i = 1,2, ..., n). A successful pull on any arm at time t yieldsa rewardat (O< a < 1), whilstan unsuccessfulpull yieldsa zero reward. At timezero O4has the probabilitydensity (o?e(O)?+f3(O)+1)! (4(0)! Pi(O)!)-l 6ii(O)(l- o i.e. a beta distribution with parameters (oci(O),/3i(0)), and these distributionsare independent forthe differentarms. The problemis to decide whicharm to pull nextat each stage so as to maximizethe total expectedreward from an infinitesequence of pulls. From Bayes' theoremit followsthat at everystage Oi has a beta distribution,but with parameterswhich change at each pull on arm i. If in the firstt pulls thereare r successes,the new values of the parameters,which we denote by (cxi(t),f3i(t)), are (U'i(O)+ r,fi(O) + t- r). If the (t + 1)st pull on arm i takes place at times, the expectedreward, conditional on the record of successes and failures up to then, is as times the expected value of a beta variate with parameters(oii(t), pi(t)), whichis (oci(t)+ l)/(oti(t)+ pi(t) + 2). Both theproblems described above involvea sequenceof decisions,each of whichis based on moreinformation than its predecessors, and thusboth problems may be tackledby dynamic 1979] GirrINS- BanditProcesses and DynamicAllocation Indices 149 programming(see Bellman,1957). This is a computationalalgorithm based on the principle that,"an optimalpolicy has the propertythat whateverthe initialstate and initialdecision, theremaining decisions must constitute an optimalpolicy with regard to thestate resulting from thefirst decision". This observationmeans thatif the optimalpolicy from a certainstage (or time)onwards is known,then it is relativelyeasy to extendthis policy so as to givean optimal policystarting one stageearlier. Repetitionof thisprocedure is the basis of an algorithmfor solvingsuch problems,which is oftendescribed as a processof backwardsinduction. A simplerprocedure than backwardsinduction is at each stage to make that decision whichmaximizes the expectedreward before the nextdecision time. This procedurewill be termeda one-steplook-aheadpolicy, following the terminology used by Ross (1970) forstopping problems. The idea is that each decisionis based on what may happen in just one further timeunit or step. The notionof a one-steplook-ahead policy may be extendedin the obvious way to form s-steplook-ahead policies. In generalsuch policiesperform better as s increasesand approach optimalityas s tendsto infinity,whilst the algorithms to whichthey lead becomeprogressively morecomplex as s increases. As a furtherextension of an s-steplook-ahead policy we may allow the numberof stepsT whichwe look ahead at each stageto dependin an arbitrarymanner on whathappens whilst those steps are takingplace, so that -ris a randomvariable. Given any rule for takingour sequenceof decisions,r may be chosenso as in some senseto maximizethe expectedrate of rewardper step forthe next r steps. A second maximizationwith respect to decisionrules selectsa decisionrule. Our extendedlook-ahead policy starts by followingthe decisionrule just describedfor the randomnumber of steps r. The processof findinga decisionrule, and a correspondingrandom number of furthersteps r', is thenrepeated with respect to the state reachedafter the firstr steps. The new ruleis followedfor the nextr' steps,and the process may be repeatedindefinitely. In thisway a rule is definedwhich specifies the decisionto be made at everystage. Such a rulewill be termedaforwards induction policy, in contrastwith the backwardsinduction of dynamicprogramming. A formaldefinition is givenin Section 3. Forwardsinduction policies are optimalfor a class of problems,which includes the two problemsdescribed above, in whicheffort is allocatedin a sequentialmanner between a number of competingcandidates for that effort,a resultwhich will be describedas theforwards inductiontheorem. These candidateswill be describedas alternativebandit processes. From the optimalityof forwardsinduction policies it followsthat a dynamicallocation index (DAI) may be definedon the statespace of each banditprocess, with the propertythat an optimal policymust at each stageallocate effortto one of thosebandit processes with the largestDAI value. This resultwill be describedas the DAI theoremand the policyas a DAI policy. The proofsof theseresults will be publishedseparately (Gittins, 1979). The existenceof a functionwith this property, and the factthat it may be writtenin the formused here,were proved in earlierpapers (Gittins and Jones,1 974a; Gittinsand Glazebrook, 1977) withoutusing the concept of a forwardsinduction policy, and the particularcases discussedin the presentpaper depend only on theseresults. The approach via the forwards inductiontheorem has the advantagethat it is intuitivelyplausible that such a resultshould hold, and it leads naturally,as we shall see, to the generalfunctional form of the dynamic allocationindex. Moreover,the forwardsinduction theorem continues to hold underappro- priateconditions, and essentiallythe same proofworks, if bandit processes arrive in a random manner,or are subjectto precedenceconstraints. This leads to resultsanalogous to the DAI theoremin thetheories of priority queues and ofmore complex stochastic scheduling situations. Some of theseapplications have been describedby Nash (1973) and Glazebrook (1976a, b), respectively.A morecomplete account, using the simplifying concept of a forwardsinduction policy,will be publishedin due course. Sometimes,too, as shownby Glazebrook (1978a), a decisionproblem may be simplifiedby expressingjust part of the problemin termsof bandit processes. 150 GrrrNS - BanditProcesses and DynamicAllocation Indices [No. 2, In the presentpaper theseextensions are mentionedonly in passing. The aims are: (i) to give a unifiedaccount, in the contextof Markov decision processesand
Details
-
File Typepdf
-
Upload Time-
-
Content LanguagesEnglish
-
Upload UserAnonymous/Not logged-in
-
File Pages31 Page
-
File Size-