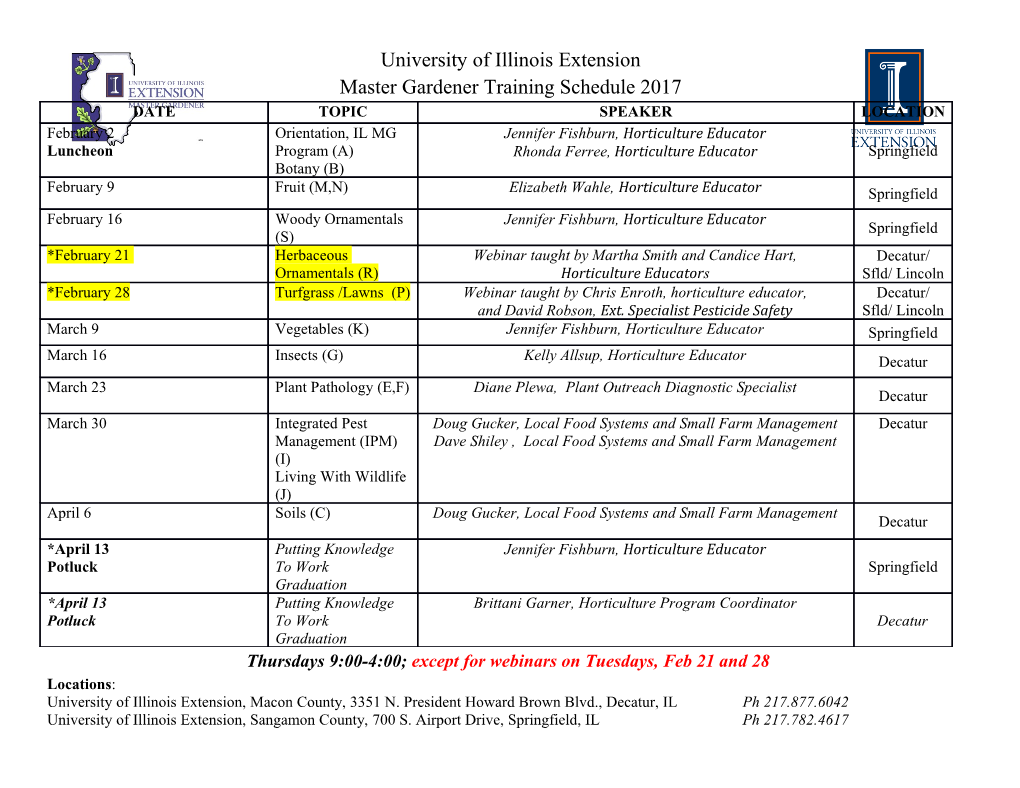
UCAM-CL-TR-721 Technical Report ISSN 1476-2986 Number 721 Computer Laboratory Investigating classification for natural language processing tasks Ben W. Medlock June 2008 15 JJ Thomson Avenue Cambridge CB3 0FD United Kingdom phone +44 1223 763500 http://www.cl.cam.ac.uk/ c 2008 Ben W. Medlock This technical report is based on a dissertation submitted September 2007 by the author for the degree of Doctor of Philosophy to the University of Cambridge, Fitzwilliam College. Technical reports published by the University of Cambridge Computer Laboratory are freely available via the Internet: http://www.cl.cam.ac.uk/techreports/ ISSN 1476-2986 Abstract This report investigates the application of classification techniques to four natural lan- guage processing (NLP) tasks. The classification paradigm falls within the family of statistical and machine learning (ML) methods and consists of a framework within which a mechanical `learner' induces a functional mapping between elements drawn from a par- ticular sample space and a set of designated target classes. It is applicable to a wide range of NLP problems and has met with a great deal of success due to its flexibility and firm theoretical foundations. The first task we investigate, topic classification, is firmly established within the NLP/ML communities as a benchmark application for classification research. Our aim is to arrive at a deeper understanding of how class granularity affects classification accuracy and to assess the impact of representational issues on different classification models. Our second task, content-based spam filtering, is a highly topical application for classification techniques due to the ever-worsening problem of unsolicited email. We assemble a new corpus and formulate a state-of-the-art classifier based on structured language model com- ponents. Thirdly, we introduce the problem of anonymisation, which has received little attention to date within the NLP community. We define the task in terms of obfuscating potentially sensitive references to real world entities and present a new publicly-available benchmark corpus. We explore the implications of the subjective nature of the problem and present an interactive model for anonymising large quantities of data based on syn- tactic analysis and active learning. Finally, we investigate the task of hedge classification, a relatively new application which is currently of growing interest due to the expansion of research into the application of NLP techniques to scientific literature for information extraction. A high level of annotation agreement is obtained using new guidelines and a new benchmark corpus is made publicly available. As part of our investigation, we de- velop a probabilistic model for training data acquisition within a semi-supervised learning framework which is explored both theoretically and experimentally. Throughout the report, many common themes of fundamental importance to classifi- cation for NLP are addressed, including sample representation, performance evaluation, learning model selection, linguistically-motivated feature engineering, corpus construction and real-world application. 3 4 Acknowledgements Firstly I would like to thank my supervisor Ted Briscoe for overseeing the progress of this doctorate; for his relentless belief in the quality of my work in the face of what often seemed to me highly convincing evidence to the contrary. I would also like to thank my colleagues here in the Lab for their companionship, and for fruitful discussion and advice; in particular, Andreas Vlachos and Mark Craven who both spent time reading my work and offering invaluable technical guidance, and Nikiforos Karamanis who generously helped with annotation and discussion. At different times and places, the following people have all contributed to this work by offering me the benefit of their expertise, for which I am very grateful: Karen Sp¨arck Jones, Ann Copestake, Simone Teufel, Joshua Goodman, Zoubin Ghahramani, Sean Holden, Paul Callaghan, Vidura Seneviratne, Mark Gales, Thomas Hain, Bill Hollingsworth and Ruth Seal. This research was made possible by a University of Cambridge Millennium Scholarship. On a personal note, I would like to acknowledge my sirenical office partners Anna and Rebecca; it has been a pleasure to begin and end my postgraduate journey with you. I would also like to thank my PhD buddies Wayne Coppins and Ed Zaayman for making the ride so much more entertaining, and in fact all my friends, both in and out of Cambridge; you're all part of this. Special acknowledgement goes to my grandfather George who kept me on my toes with his persistent enquiry into my conference attendance and publication record, and to Lizzie for proofreading and patiently allowing me to defend the split infinitive. Final and profound thanks goes to my family: Paul, Jane, Matt and Abi, for everything you mean to me, and to my Creator who fills all in all. 5 6 For George, who did it all on a typewriter... 8 Contents 1 Introduction 13 1.1 Project Overview . 13 1.1.1 Topic Classification . 14 1.1.2 Spam Filtering . 14 1.1.3 Anonymisation . 14 1.1.4 Hedge Classification . 14 1.2 Research Questions . 15 1.3 Tools . 16 1.3.1 RASP . 16 1.3.2 SVMlight ................................. 17 1.4 Report Structure . 17 2 Background and Literature Overview 19 2.1 The Classification Model . 19 2.1.1 Classification by Machine Learning . 20 2.1.2 Classification versus Clustering . 20 2.1.3 Supervision . 21 2.1.4 Approaches to Classification . 21 2.1.5 Generalisation . 23 2.1.6 Representation . 23 2.2 Classification in NLP { Literature Overview . 24 3 Topic Classification 29 3.1 Introduction and Motivation . 29 3.2 Methodology . 30 3.2.1 Data . 30 3.2.2 Evaluation Measures . 31 3.2.3 Classifiers . 32 3.3 Investigating Granularity . 33 3.4 Classifier Comparison . 37 3.5 Document Representation . 39 3.5.1 Feature Selection . 40 3.5.2 Salient Region Selection . 41 3.5.3 Balancing the Training Distributions . 42 3.5.4 Introducing More Informative Features . 44 3.6 Complexity . 48 9 10 CONTENTS 3.7 Conclusions and Future Work . 48 4 Spam Filtering 51 4.1 Introduction . 51 4.2 Related Work . 51 4.3 A New Email Corpus . 52 4.4 Classification Model . 54 4.4.1 Introduction . 54 4.4.2 Formal Classification Model . 55 4.4.3 LM Construction . 57 4.4.4 Adaptivity . 58 4.4.5 Interpolation . 59 4.5 Experimental Analysis { LingSpam ...................... 60 4.5.1 Data . 60 4.5.2 Experimental Method . 60 4.5.3 Evaluation Measures . 61 4.5.4 Results . 61 4.6 Experimental Analysis { GenSpam ...................... 62 4.6.1 Classifier Comparison . 62 4.6.2 Implementation . 63 4.6.3 Experimental Method . 63 4.6.4 Data . 63 4.6.5 Evaluation Measures . 64 4.6.6 Hyperparameter Tuning . 64 4.6.7 Results and Analysis . 65 4.7 Discussion . 67 4.8 Conclusions . 68 5 Anonymisation 71 5.1 Introduction . 71 5.2 Related Work . 72 5.3 The Anonymisation Task . 72 5.4 Corpus . 74 5.4.1 Corpus Construction . 74 5.4.2 Pseudonymisation . 75 5.4.3 Annotation . 77 5.4.4 Format . 77 5.5 Classification . 78 5.5.1 Lingpipe . 78 5.5.2 An Interactive Approach . 78 5.6 Experimental Method . 83 5.6.1 Evaluation Measures . 83 5.6.2 Syntactic Analysis . 84 5.6.3 Experimental Procedure . 84 5.7 Results and Analysis . 84 5.7.1 Results . 84 5.7.2 Discussion . 86 CONTENTS 11 5.7.3 Error Analysis . 87 5.8 Conclusions and Future Work . 88 6 Hedge Classification 89 6.1 Introduction . 89 6.2 Related Work . 90 6.2.1 Hedge Classification . 90 6.2.2 Semi-Supervised Learning . 90 6.3 The Task . 91 6.4 Data . 94 6.5 Annotation and Agreement . 94 6.6 Discussion . 95 6.7 A Probabilistic Model for Training Data Acquisition . 97 6.8 Hedge Classification . 98 6.9 Classification . 99 6.10 Experimental Evaluation . ..
Details
-
File Typepdf
-
Upload Time-
-
Content LanguagesEnglish
-
Upload UserAnonymous/Not logged-in
-
File Pages138 Page
-
File Size-