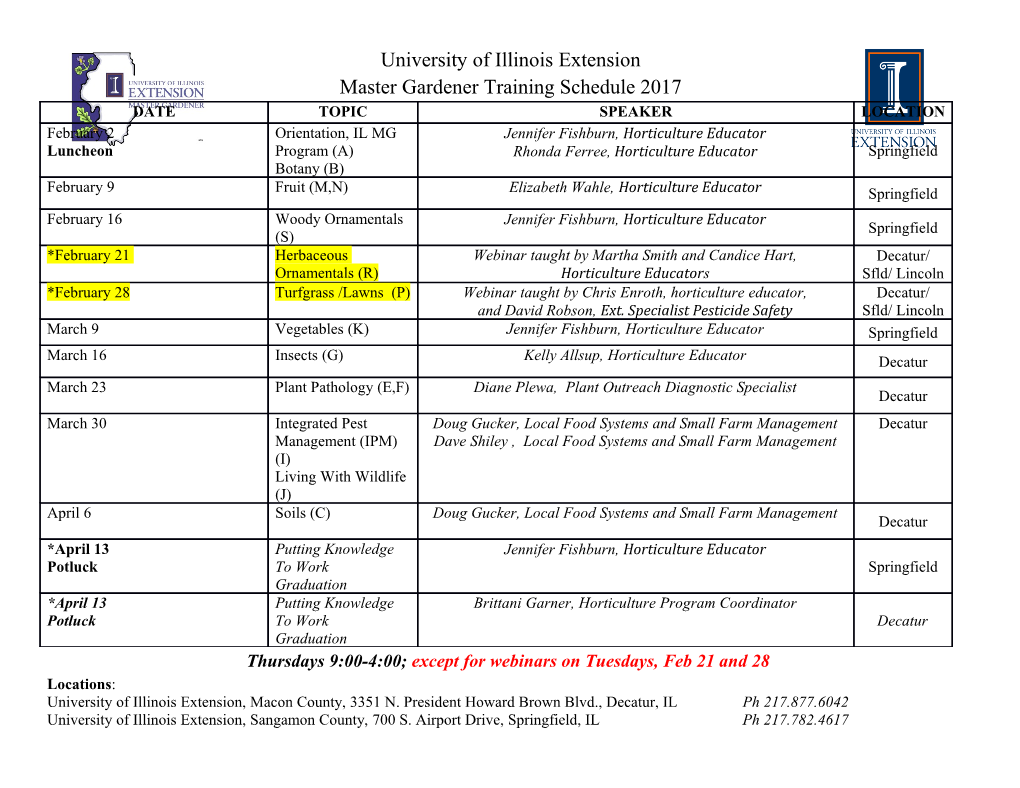
On the EXISTENCE OF HOPFIELD NEURAL NETWORKS: SYNTHESIS OF HOPFIELD TYPE ASSOCIATIVE MEMORIES: Garimella Rama Murthy* and Moncef Gabbouj** *International Institute of Information Technology, Hyderabad, India **Tampere University of Technology, Tampere, Finland ABSTRACT In this research paper, the problem of existence of the associative memory synthesized by Hopfield is addressed and solved. Using Hadamard matrix of suitable dimension, an algorithm to synthesize real valued Hopfield neural network is discussed. The problem of existence and synthesis of a certain complex Hopfield neural network is addressed and solved. Also, synthesis of real and complex Hopfield type associative memories is discussed. Novel Complex Hadamard matrices are defined and studied. Using such matrices Novel Complex Hadamard transform is proposed. Also complex Walsh functions are proposed. Certain interesting distance measures are defined. 1. INTRODUCTION : In the progress of Science and Technology, homosapiens attempted mathematical model of natural ( physical / chemical / biological ) systems. Specifically, there were attempts to model the biological neural network. McCulloch and Pitts proposed a model of artificial neuron. Using that model of neuron and its variations, researchers comprehensively built the theory of Artificial Neural Networks (ANNs). In this theory, some important milestones were Single Layer Perceptron (SLP) and Multi Layer Perceptron (MLP). Multi Layer Perceptron is an example of feedforward neural network that received considerable attention and many applications continue to utilize such a neural network. Researchers were naturally motivated to investigate feedback neural networks. Hopfield effectively succeeded in proposing a feedback neural network model that acts as an associative memory. Hopfield neural network model led to intense research activity on various types of associative memories such as the Bi-directional Associative Memory (BAM ). Researchers were also motivated to investigate neural networks in which the input, synaptic weights, outputs are allowed to be complex numbers. Thus, these research efforts led to the comprehensive research area of Complex Valued Neural Networks (CVNNs) [2, MGZ], [6]. The authors proposed a complex valued generalization of Hopfield Neural Network (HNN) [3, RaP] . It is a different model from other types of Complex Hopfield Neural Network investigated by other researchers. In this research paper, the authors investigate the question of existence and synthesis ( algorithmic procedure ) of real valued associative memory proposed by Hopfield. The results are also generalized to a Complex Valued Hopfield neural network. This research paper is organized in the following manner. In Section 2, detailed explanation of real valued Hopfield neural network is provided. Also, an interesting Complex Valued Hopfield Neural Network proposed by the authors is discussed. In Section 3, existence of real valued Hopfield neural network is discussed. In Section 4, existence of a complex valued Hopfield neural network is discussed. In Section 5, synthesis of Hopfield type Associative Memories is discussed. The research paper concludes in Section 6. 2. Hopfield Neural Network: Review of Research Literature : Hopfield neural network is an Artificial Neural Network model. It is a nonlinear dynamical system represented by a weighted, undirected graph. The nodes of the graph represent artificial neurons and the edge weights correspond to synaptic weights. At each neuron / node, there is a threshold value. Thus, in summary, a Hopfield neural network can be represented by a synaptic weight matrix, M and a threshold vector, T. The order of the network corresponds to the number of neurons [1, Hop]. Every neuron is in one of the two possible states +1 or -1. Thus, the state space of Nth order Hopfield Neural Network ( HNN ) is the N- dimensional unit hypercube. Let the state of ith neuron at time ‘t’ be denoted by ͐$ ʚͨʛ ) . Thus, the state of the non-linear dynamical system is ʚ ͐$ ʚͨʛ Ǩ ʜ ƍ1 ͣͦ Ǝ 1 ʝ represented by the N x 1 vector, ͐Ŭ ʚͨʛ. The state updation at the ith node is governed by the following equation = Sign { ….(2.1) , ͐$ ʚ ͨ ƍ 1 ʛ ∑%Ͱͥ ͇$% ͐% ʚͨʛ Ǝ ͎$ ʝ where Sign (. ) is the Signum function. In other words, is +1 if the term in ͐$ ʚͨ ƍ 1ʛ the brackets is non-negative, otherwise is -1. Depending on the set of nodes at ͐$ ʚͨ ƍ 1ʛ which the state updation (2.1) is performed at any time t, the Hopfield Neural Network ( HNN ) operation is classified into the following modes. • Serial Mode: The state updation in equation (2.1) is performed exactly at one of the nodes / neurons at time ‘t’ • Fully Parallel Mode: The state updation in equation (2.1) is performed simultaneously at all the “N” nodes / neurons at time ‘t’. In the state space of Hopfield Neural Network, a non-linear dynamical system, there are certain distinguished states, called the “stable states”. • Definition : A state ͐Ŭ ʚͨʛ is called a stable state if and only if ͐Ŭ ʚͨʛ = Sign { M ͐Ŭ ʚͨʛ - T } ….(2.2) Thus, once the Hopfield Neural Network ( HNN ) reaches the stable state, irrespective of the mode of operation of the network, the HNN will remain in that state for ever. Thus, there is no further change of the state of HNN once a stable state is reached. The following convergence Theorem summarizes the dynamics of Hopfield Neural Network ( HNN ). It characterizes the operation of neural network as an associative memory. Theorem 1: Let the pair N = ( M,T ) specify a Hopfield neural network. Then the following hold true: [1] Hopfield : If N is operating in a serial mode and the elements of the diagonal of M are non-negative, the network will always converge to a stable state ( i.e. there are no cycles in the state space ). [2] Goles: If N is operating in the fully parallel mode, the network will always converge to a stable state or to a cycle of length 2 ( i.e the cycles in the state space are of length ƙ 2 ). Remark 1: It should be noted that in [Rama1], it is shown that the synaptic weight matrix can be assumed to be an arbitrary symmetric matrix. • NOVEL COMPLEX HOPFIELD NEURAL NETWORK In research literature on artificial neural networks, there were attempts by many researchers to propose Complex Valued Neural Networks ( CVNNs) in which the synaptic weights, inputs are necessarily complex numbers [2, MGZ]. In our research efforts on CVNNs, we proposed and studied one possible Complex Hopfield neural network first discussed in [3, RaP], [7-12]. The detailed descriptions of such an artificial neural network requires the following concepts. A. Complex Hypercube: Consider a vector of dimension “N” whose components assume values in the following set { 1+ j1 , 1 –j 1, -1 + j 1, -1 – j 1 }.Thus there are 4N points as the corners of a set called the ‘Complex Hypercube”. B. Complex Signum Function: Consider a complex number “a + j b”. The “Complex Signum Function” is defined as follows: Csign ( a + j b ) = Sign ( a ) + j Sign ( b ). Now we briefly summarize the Complex Hopfield Neural Network first proposed in [3,RaP ]. • The synaptic weight matrix of such an artificial neural network is a Hermitian matrix. The activation function in such a complex valued neural network is the complex signum function. The convergence Theorem associated with such a neural network is provided below. Theorem 2: Let N=( W, T) be a neural network, with W being a synaptic weight matrix which is a Hermitian matrix with nonnegative diagonal elements and T being the threshold vector; then the following hold. 1) If N is operating in a serial mode and the consequent state computation of any arbitrary node ( i) is as follows: where ͐$ʚͨ ƍ 1ʛ Ɣ ̽ͧ͛͢Ƴ͂$ʚͨʛƷ, ) , ͂$ʚͨʛ Ɣ ∑%Ͱͥ ͑$,% ͐%ʚͨʛ Ǝ ͎$ then the network will always converge to an stable state. 2) If N is operating in a fully parallel mode and the consequent state computation of any arbitrary node (i) is similar to that of the serial mode, then the network will always converge to a stable state or to a cycle of length 2. Remark 2 : It has been shown in [RaN], the diagonal elements of W can be arbitrary real numbers and need not be necessarily non-negative. 3. Existence of Real Valued Hopfield Neural Network: • Linear Algebraic Structure of Hopfield Neural Network: Hopfield synthesized a real valued synaptic weight matrix from the patterns to be stored in such a way that the network so obtained has these patterns as stable states. The weight matrix given by Hopfield is as follows: ....(3.1) ͑ Ɣ ∑%Ͱͥʚ͒%͒% Ǝ ̓ʛ where S is the number of patterns to be stored ( S < N ), I is the identity matrix and are ͒ͥ, ͒ͦ … ͒ the orthogonal real patterns (lying on the real hypercube) to be stored. Now, we would like to understand the linear algebraic structure of the symmetric synaptic weight matrix, W. Some results related to W are as follows • Trace (W) is zero. Thus, the sum of eigenvalues of W are zero. • It is easy to see that ͑ ͒& Ɣ ʚ ͈ Ǝ ͍ʛ ͒& ͫͨ͜͝ ͍ Ɨ ͈. Now, we need the following definition in the succeeding discussion. Definition : The local minimum vectors of the energy function associated with W are called anti-stable states . Thus, if ͩ is an anti-stable state of matrix W, then it satisfies the condition ͩ Ɣ Ǝ͍͛͢͝ ʚ ͑ ͩ ʛ. Using the definitions, we have the following general result Lemma 1: If a corner of unit hypercube is an eigenvector of W corresponding to positive / negative eigenvalue, then it is also a stable / anti-stable state. Proof: Follows from the utilization of definitions of eigenvectors, stable / anti-stable states. Q.E.D. Now we arrive at the spectral representation of W using the above results.
Details
-
File Typepdf
-
Upload Time-
-
Content LanguagesEnglish
-
Upload UserAnonymous/Not logged-in
-
File Pages17 Page
-
File Size-