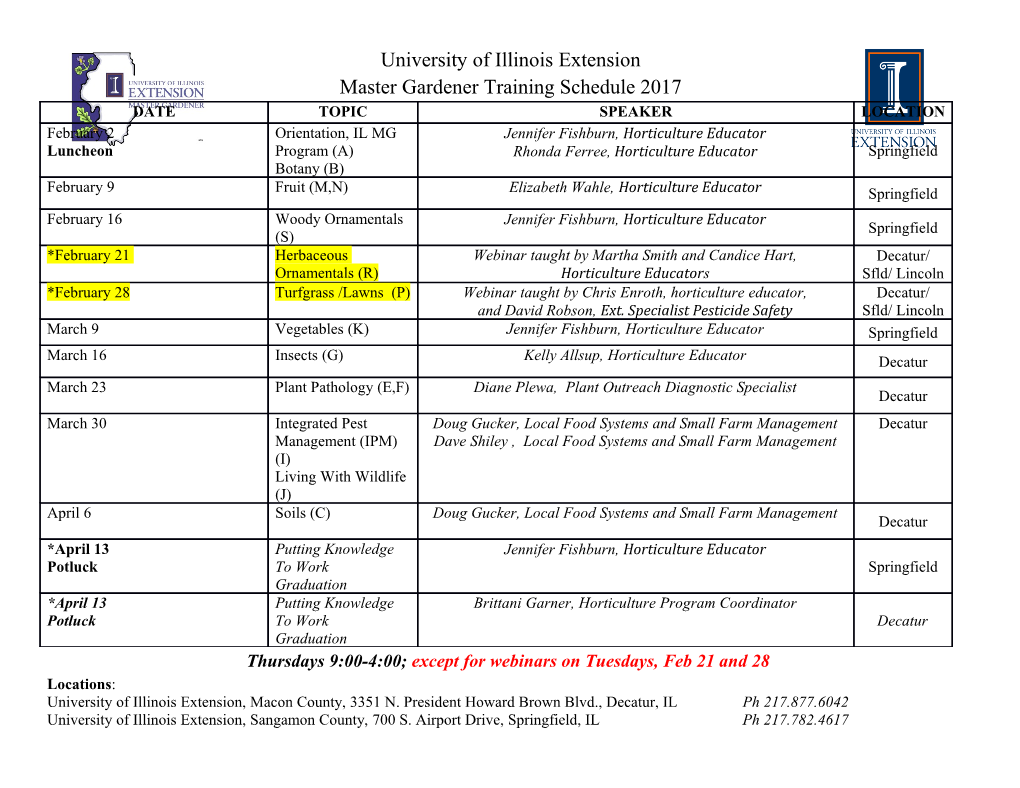
Digital Communication Systems ECS 452 Asst. Prof. Dr. Prapun Suksompong [email protected] 4. Mutual Information and Channel Capacity Office Hours: Check Google Calendar on the course website. Dr.Prapun’s Office: 6th floor of Sirindhralai building, 1 BKD Digital Communication Systems ECS 452 Asst. Prof. Dr. Prapun Suksompong [email protected] Operational Channel Capacity 14 Channel Capacity [Section 4.2] “Operational”: max rate at which reliable communication is possible Channel Capacity Arbitrarily small error probability can be achieved. “Information”: [bpcu] [Section 4.3] Shannon [1948] shows that these two quantities are actually the same. 15 System Model for Section 3.4 Message Transmitter Information Source Channel Digital Source Encoder Encoder Modulator Transmitted Remove Add X: channelSignal input redundancy systematic redundancy EquivalentChannel Channel Recovered Decoded Message value Received Y: channelSignal output Source Channel Digital Destination Decoder Decoder (Detector)Demodulator Receiver In Chapter 3, we studied how to find the optimal decoder. Some results from Section 3.3-3.4 17 Under appropriate assumptions, minimum distance decoder is optimal. System Model for Section 3.5 Message Transmitter Information Source Channel Source Encoder Encoder Remove Add systematic X: channel input redundancy redundancy Equivalent Channel Recovered Decoded Message value Y: channel output Source Channel Destination Decoder Decoder (Detector) Receiver We then introduced the channel encoder box. [3.62] Block Encoding Channel X Encoder k bits k bits k bits n bits n bits n bits , code Code rate = [3.62] Block Encoding Channel X Encoder k bits k bits k bits n bits n bits n bits , code Code rate = Example: Repetition Code Channel X 1 0 1 111110000011111 Encoder 1bit 1bit 1bit 5 bits 5 bits 5 bits , code Code rate = [3.62] Block Encoding Channel X Encoder k bits n bits 2 possibilities Choose 2 from 2 possibilities to be [Figure 13] used as codewords. Codebook Repetition Code 22 Review: Channel Encoder and Decoder 2 possibilities Choose 2 from 2 possibilities to be used as codewords. Message (Data block) Channel Digital Encoder Modulator Transmitted k bits k bits k bits Signal Add n bits n bits n bits systematic redundancy 0 1-p 0 p Channel Binary Symmetric p 1 1-p 1 Channel with p < 0.5 Received Noise & Interference Signal Channel Digital Recovered Message Decoder Demodulator 23 minimum distance decoder Example: Repetition Code [Figure 14] Original Equivalent Channel: 0 1-p 0 p p 1 1-p 1 BSC with crossover probability p = 0.01 New (and Better) Equivalent Channel: 1-p Repetition 0 0 Majority 0 0 Code with p p Vote 1 1 n = 5 1 1-p 1 Use repetition code with n = 5 at the transmitter Use majority vote at the receiver 5 5 5 1 1 1 New BSC with 3 4 5 10 24 [From ECS315] 25 MATLAB close all; clear all; % ECS315 Example 6.58 % ECS452 Example 3.66 C = [0 0 0 0 0; 1 1 1 1 1]; % repetition code p = (1/100); PE_minDist(C,p) Code C is defined by putting all its (valid) codewords as its rows. For repetition >> PE_minDist_demo1 code, there are two codewords: 00..0 and 11..1. ans = 9.8506e-06 Crossover probability of the binary symmetric channel. 26 function PE = PE_minDist(C,p) MATLAB % Function PE_minDist_3 computes the error probability P(E) when code C % is used for transmission over BSC with crossover probability p. % Code C is defined by putting all its (valid) codewords as its rows. M = size(C,1); k = log2(M); n = size(C,2); % Generate all possible received vectors Y = dec2bin(0:2^n-1)-'0'; % Normally, we need to construct an extended Q matrix. However, because % each conditional probability in there is a decreasing function of the % Hamming distance, we can work with the distances instead of the % conditional probability. In particular, instead of selecting the max in % each column of the Q matrix, we consider min distance in each column. dmin = zeros(1,2^n); for j = 1:(2^n) % for each received vector y, y = Y(j,:); % find the minimum distance (the distance from y to the closest % codeword) d = sum(mod(bsxfun(@plus,y,C),2),2); dmin(j) = min(d); end % From the distances, calculate the conditional probabilities. % Note that we compute only the values that are to be selected (instead of % calculating the whole Q first). n1 = dmin; n0 = n-dmin; Qmax = (p.^n1).*((1-p).^n0); % Scale the conditional probabilities by the input probabilities and add % the values. Note that we assume equally likely input. PC = sum((1/M)*Qmax); PE = 1-PC; end 27 MATLAB 28 Example: Repetition Code Original Equivalent Channel: 0 1-p 0 p p 1 1-p 1 BSC with crossover probability p New (and Better) Equivalent Channel: 1-p Repetition 0 0 Majority 0 0 Code with p p Vote 1 1 n = 5 1 1-p 1 Use repetition code at the transmitter Use majority vote at the receiver New BSC with new crossover probability 29 MATLAB close all; clear all; 0.5 % ECS315 Example 6.58 0.45 % ECS452 Example 3.66 0.4 C = [0 0 0 0 0; 1 1 1 1 1]; 0.35 0.3 0.25 syms p; P(E) PE = PE_minDist(C,p) 0.2 pp = linspace(0,0.5,100); 0.15 0.1 PE = subs(PE,p,pp); 0.05 plot(pp,PE,'LineWidth',1.5) 0 0 0.05 0.1 0.15 0.2 0.25 0.3 0.35 0.4 0.45 0.5 xlabel('p') p ylabel('P(E)') grid on >> PE_minDist_demo2 PE = (p - 1)^5 + 10*p^2*(p - 1)^3 - 5*p*(p - 1)^4 + 1 30 Searching for the best encoder Now that we have MATLAB function PE_minDist, for specific values of n, k, we can try to search for the encoder that minimizes the error probability. Recall that, from Example 3.64, there are reasonable encoders. Even for small n and k, this is a large space to look at every possible cases. 31 Example: Repetition Code 0 1-p 0 0 0 Repetition p Majority p Vote Code 1 1 1 1-p 1 0.1 1 0.1 3 3 3 1 0.0280 2 3 5 5 5 5 1 1 0.0086 3 4 5 7 0.0027 9 8.9092 10 11 2.9571 10 32 Channel Capacity [Section 4.2] “Operational”: max rate at which reliable communication is possible Channel Capacity Arbitrarily small error probability can be achieved. “Information”: [bpcu] [Section 4.3] Shannon [1948] shows that these two quantities are actually the same. 33 Digital Communication Systems ECS 452 Asst. Prof. Dr. Prapun Suksompong [email protected] 5. Channel Coding Office Hours: Check Google Calendar on the course website. Dr.Prapun’s Office: 6th floor of Sirindhralai building, 1 BKD Review: Channel Encoder and Decoder Message Transmitter S Information Source Channel Digital Source Encoder Encoder Modulator Transmitted Add X: channelSignal input systematic redundancy 0 1-p 0 p Channel p 1 1-p 1 Noise & Interference Recovered Message Receiver Received Y: channelSignal output Source Channel Digital Destination Decoder Decoder Demodulator 2 System Model for Chapter 5 Transmitter s Channel Digital Message Encoder Modulator Transmitted Add x: channelSignal input systematic redundancy 0 1-p 0 p Channel p 1 1-p 1 Received Noise & Interference Receiver y: channel output Signal Channel Digital Recovered Message Decoder Demodulator 3 Vector Notation 0,0: the zero vector ⋮ (the all-zero vector) : column vector ⋮ 1,1: the one vector (the all-one vector) : row vector ,,…,,… Subscripts represent element indices inside individual vectors. th and refer to the i elements inside the vectors and , respectively. When we have a list of vectors, we use superscripts in parentheses as indices of vectors. is a list of M column vectors is a list of M row vectors and refer to the ith vectors in the corresponding lists. 4 Harpoon a long, heavy spear attached to a rope, used for killing large fish or whales 5 Review: Channel Decoding Recall 1. The MAP decoder is the optimal decoder. 2. When the codewords are equally-likely, the ML decoder the same as the MAP decoder; hence it is also optimal. 3. When the crossover probability of the BSC p is < 0.5, ML decoder is the same as the minimum distance decoder. In this chapter, we assume the use of minimum distance decoder. arg min , Also, in this chapter, we will focus less on probabilistic analysis, but more on explicit codes. 6 Digital Communication Systems ECS 452 Asst. Prof. Dr. Prapun Suksompong [email protected] 5.1 Binary Linear Block Codes Office Hours: Check Google Calendar on the course website. Dr.Prapun’s Office: 6th floor of Sirindhralai building, 7 BKD Review: Block Encoding We mentioned the general form of channel coding over BSC. In particular, we looked at the general form of block codes. Block Encoder k bits k bits k bits n bits n bits n bits Code length “Dimension” of the code (n,k) codes: n-bit blocks are used to conveys k-info-bit blocks Assume n > k codewords “messages” Max. achievable rate Rate: . Recall that the capacity of BSC is 1 . For ∈ 0,1 , we also have ∈ 0,1 . Achievable rate is < 1. 8 System Model for Section 5.1 Transmitter s Channel Digital Message Encoder Modulator n bits Transmitted k bits Add x: channelSignal input systematic redundancy 0 1-p 0 p Channel p 1 1-p 1 Received Noise & Interference Receiver y: channel output Signal Recovered Message Channel Digital Decoder Demodulator k bits n bits 9 = the collection of all codewords for the code considered Each n-bit block is selected from .
Details
-
File Typepdf
-
Upload Time-
-
Content LanguagesEnglish
-
Upload UserAnonymous/Not logged-in
-
File Pages249 Page
-
File Size-