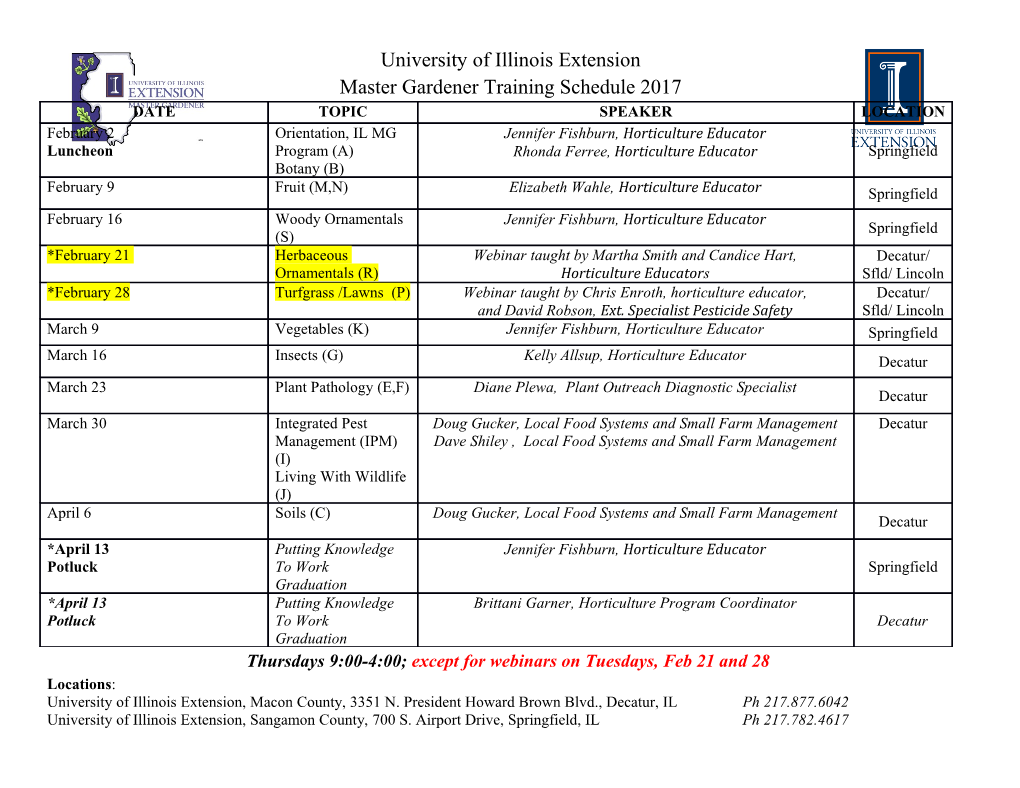
Computational Approaches To Predict Effect Of Epigenetic Modifications On Transcriptional Regulation Of Gene Expression Sharmi Banerjee Dissertation submitted to the Faculty of the Virginia Polytechnic Institute and State University in partial fulfillment of the requirements for the degree of Doctor of Philosophy in Electrical Engineering Pratap Tokekar, Co-chair Xiaowei Wu, Co-chair William Baumann Inyoung Kim Anil Vullikanti September 05, 2019 Blacksburg, Virginia Keywords: Epigenetic factors, gene expression, transcription factors, histone marks, DNA methylation Copyright 2019, Sharmi Banerjee Computational Approaches To Predict Effect Of Epigenetic Modifications On Transcriptional Regulation Of Gene Expression Sharmi Banerjee (ABSTRACT) This dissertation presents applications of machine learning and statistical approaches to infer protein-DNA bindings in the presence of epigenetic modifications. Epigenetic modifications are alterations to the DNA resulting in gene expression regulation where the structure of the DNA remains unaltered. It is a heritable and reversible modification and often involves addition or deletion of certain chemical compounds to the DNA. Histone modification is an epigenetic change that involves alteration of the histone proteins { thus changing the chromatin (DNA wound around histone proteins) structure { or addition of methyl-groups to the Cytosine base adjacent to a Guanine base. Epigenetic factors often interfere in gene expression regulation by promoting or inhibiting protein-DNA bindings. Such proteins are known as transcription factors. Transcription is the first step of gene expression where a particular segment of DNA is copied into the messenger-RNA (mRNA). Transcription factors orchestrate gene activity and are crucial for normal cell function in any organism. For example, deletion/mutation of certain transcription factors such as MEF2 have been associated with neurological disorders such as autism and schizophrenia. In this dissertation, different computational pipelines are described that use mathematical models to explain how the protein-DNA bindings are mediated by histone modifications and DNA-methylation affecting different regions of the brain at different stages of development. Computational Approaches To Predict Effect Of Epigenetic Modifications On Transcriptional Regulation Of Gene Expression Sharmi Banerjee (GENERAL AUDIENCE ABSTRACT) A cell is the basic unit of any living organism. Cells contain nucleus that contains DNA, self- replicating material often called the blueprint of life. For sustenance of life, cells must respond to changes in our environment. Gene expression regulation, a process where specific regions of the DNA (genes) are copied into messenger RNA (mRNA) molecules and then translated into proteins, determines the fate of a cell. It is known that various environmental (such as diet, stress, social interaction) and biological factors often indirectly affect gene expression regulation. In this dissertation, we use machine learning approaches to predict how certain biological factors interfere indirectly with gene expression by changing specific properties of DNA. We expect our findings will help in understanding the interplay of these factors on gene expression. Acknowledgments The dissertation owes so much to so many. Foremost among them, Dr.David Xie, for his constant guidance and encouragement, and giving me enough freedom to pursue my goals for personal development. It has been a privilege and an honor. I would like to thank Dr. Xiaowei Wu for being my mentor throughout my PhD and guiding me more than I could have expected. I would also like to thank the rest of my committee: Dr. Pratap Tokekar, Dr. William Baumann, Dr. Inyoung Kim and Dr. Anil Vullikanti for their valuable feedback, insightful discussions and hard questions. My sincere thanks also goes to Dr.Jason Xuan and Dr.Hamed Sari-Sarraf for getting me excited about research and helping me find my feet during the initial phase of the process. I would like to express my deepest gratitude to Dr. Michel Pleimling for providing me with assistantship in the last two years of my PhD. It would have be impossible for me to continue my doctoral work without his help. I could not have endured this journey without the support of my friends Sajal, Jiyoung, Meghna, Kwang, Jianlin and Xiaoran who have become my family in Blacksburg. I would like to thank my parents and sister for keeping my spirits up at times of failure. I thank my sister-in-law who shared with me her valuable academic experience and helped in critically reviewing this dissertation. Last but not the least I would like to thank my husband who bolstered me through the peaks and valleys of this journey. This dissertation belongs to him as much as it belongs to me. iv Contents List of Figures ix List of Tables xvi 1 Introduction1 2 Identification of transcriptional regulatory modules in distinct chromatin states in mouse neural stem cells7 2.1 Overview......................................7 2.2 Key challenges.................................. 12 2.2.1 Integrating histone and transcription factors.............. 12 2.2.2 Finding the optimum clustering algorithm............... 13 2.2.3 Resolution of the TRMs within chromatin states............ 13 2.3 Building blocks of the computational pipeline................. 17 2.3.1 Data-sets................................. 17 2.3.2 Chromatin state identification through genome segmentation..... 17 2.3.3 TF binding pattern clustering via Dirichlet Process Mixture of Log Gaussian Cox Processes (DPM-LGCP)................. 18 2.4 Case studies.................................... 21 v 2.4.1 Simulated data.............................. 21 2.4.2 Real data................................. 23 2.4.3 Functional enrichment of transcription factors within chromatin states 23 2.4.4 Gene expression and motif analysis................... 25 2.5 Results....................................... 25 2.5.1 Genome segmentation and chromatin state identification....... 25 2.5.2 Genome annotation enrichment..................... 26 2.5.3 Relative enrichment around TSS.................... 26 2.5.4 State sizes................................. 30 2.5.5 Functional annotation of nucleosome and domain level states..... 30 2.5.6 Chromatin state preference of individual TF binding and gene expres- sion regulation.............................. 32 2.5.7 Chromatin state and preferential TF clustering............ 34 2.5.8 Estimated clusters in chromatin states................. 37 2.5.9 Protein-DNA binding motif preferences in chromatin states...... 41 2.6 Known protein-protein interactions predicted by the algorithm........ 43 2.7 Robustness of the proposed clustering technique................ 44 2.7.1 Effect of varying domain level state sizes................ 44 2.7.2 Effect of varying number of initial clusters............... 44 2.7.3 Effect of permutation of peaks..................... 46 vi 2.8 Comparison of clustering results to other methods............... 46 2.9 Time complexity analysis............................. 47 2.10 Discussion..................................... 47 3 Recursive motif analyses identify brain epigenetic regulatory modules 53 3.1 Overview...................................... 53 3.2 Key challenges.................................. 54 3.2.1 Motif enrichment prediction in DMS.................. 54 3.3 Building blocks of the computational pipeline................. 56 3.3.1 Merging nearby DMS........................... 56 3.3.2 Clustering DMRs for motif enrichment................. 57 3.3.3 Data analysis of Whole Genome Bisulphite Sequencing (WGBS-seq). 58 3.3.4 Recursive identification of TRMs from motifs............. 59 3.3.5 Preparing motif libraries......................... 61 3.3.6 Analysis of single cell RNA-seq..................... 62 3.4 Results....................................... 64 3.4.1 A Comprehensive motif database compiled for epigenetic regulatory module identification........................... 64 3.4.2 Genome distribution of hypermethylated CpG sites identified in TET1KO and TET2KO frontal cortices...................... 65 3.4.3 TRMs identified in differentially methylated clusters......... 69 vii 3.4.4 Brain ChIP-seq data support the TRMs predicted........... 74 3.4.5 Transcription factors under the influence of DNA methylation.... 76 3.5 Discussion..................................... 78 4 Discussion and future work 81 4.1 TRM analysis with brain single cell methylomes................ 83 4.2 Cell type specific PPI obtained through ETRM prediction.......... 84 4.3 Co-expression of ETRMs in single cell and bulk RNA-seq........... 86 4.4 Summary..................................... 88 4.5 Contribution of the dissertation......................... 90 Bibliography 92 Appendices 111 Appendix A First Appendix 112 A.1 Dirichlet Process mixture of log Gaussian Cox processes............ 112 A.1.1 INLA approximation of the LGCP model................ 112 A.1.2 Algorithm for posterior inference.................... 115 viii List of Figures 2.1 A two-step process to identify chromatin-state-specific transcriptional regula- tory modules. diHMM is used to segment the genome into multiple chromatin states followed by application of the proposed clustering method to identify transcriptional regulatory modules in distinct states. Downstream analyses include gene expression comparison and de-novo motif comparison across dif- ferent chromatin states............................... 11 2.2 TF peak enrichment in different chromatin states based on a threshold re- quirement
Details
-
File Typepdf
-
Upload Time-
-
Content LanguagesEnglish
-
Upload UserAnonymous/Not logged-in
-
File Pages132 Page
-
File Size-