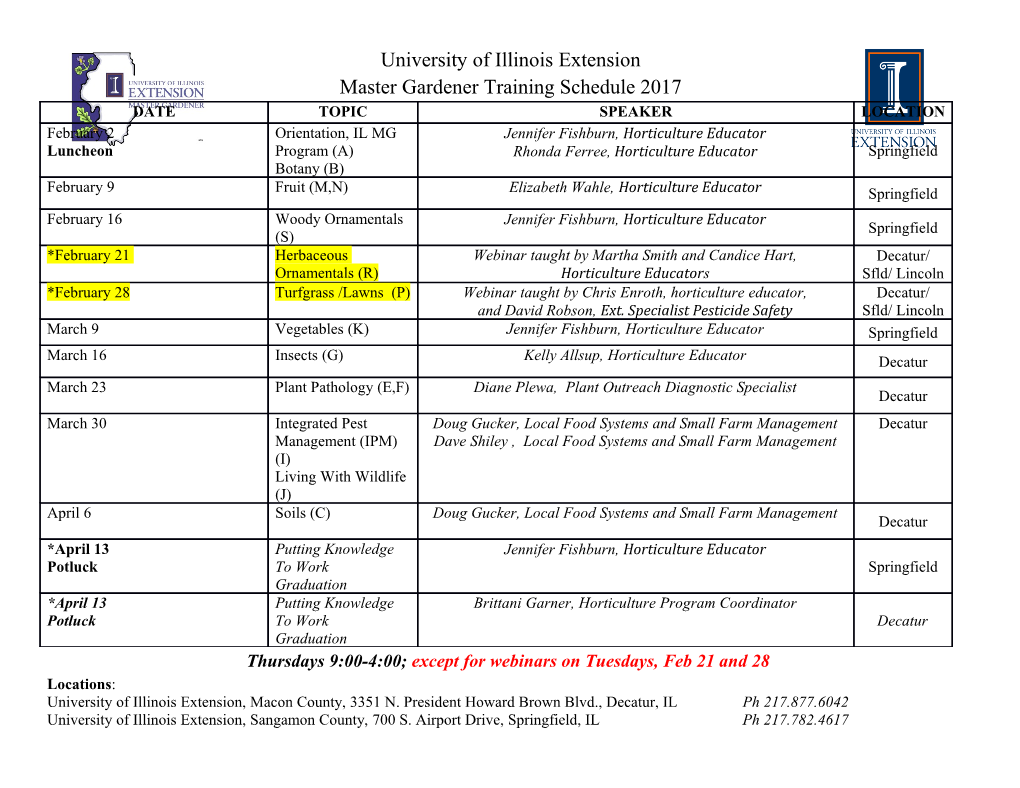
Finding Heavily-Weighted Features with the Weight-Median Sketch Extended Abstract Kai Sheng Tai, Vatsal Sharan, Peter Bailis, Gregory Valiant Stanford University ABSTRACT update “foo” 2.5 We introduce the Weight-Median Sketch, a sub-linear space data “bar” -1.9 (xt ;yt ) rLˆt structure that captures the most heavily weighted features in linear “baz” 1.8 classifiers trained over data streams. This enables memory-limited query execution of several statistical analyses over streams, including on- streaming gradient sketched estimates of line feature selection, streaming data explanation, relative deltoid data estimates classifier largest weights detection, and streaming estimation of pointwise mutual informa- Figure 1: Streaming updates to a sketched classifier with ap- tion. On a standard binary classification benchmark, the Weight- proximations of the most heavily-weighted features. Median Sketch enables estimation of the top-100 weights with 10% relative error using two orders of magnitude less space than an We introduce a new sketching algorithm—the Weight-Median uncompressed classifier. Sketch (WM-Sketch)—for binary linear classifiers trained on stream- ing data.1 As each new labeled example, (x;y) with x 2 Rd , y 2 1 INTRODUCTION f−1;+1g, is observed in the stream, we update a fixed-size data struc- ture in memory with online gradient descent. This data structure Memory-efficient sketching algorithms are a well-established tool represents a compressed version of the full d-dimensional classifier in stream processing tasks such as frequent item identification [4, trained on the same data stream. This structure can be efficiently 5, 16], quantile estimation [10], and approximate counting of dis- queried for estimates of the top-K highest-magnitude weights in the tinct items [9]. Sketching algorithms compute approximations of classifier. Importantly, we show that it suffices that this compressed these quantities in exchange for significant reductions in memory data structure is of size polylogarithmic in the feature dimension utilization. Therefore, they are a good choice when highly-accurate d. Therefore, the WM-Sketch can be used to realize substantial estimation is not essential and practitioners wish to trade off be- memory savings in high dimensional classification problems where tween memory usage and approximation accuracy [3, 24]. the user is interested in obtaining a K-sparse approximation to the Simultaneously, a wide range of streaming analytics workloads classifier, where K ≪ d. can be formulated as learning problems over streaming data. In streaming data explanation [1, 15], analyses seek to explain the 2 THE WEIGHT-MEDIAN SKETCH difference between subpopulations in the data (e.g., between an The main data structure in the WM-Sketch is identical to that used inlier class and an outlier class) using a small set of discriminative in the Count-Sketch [4]. The sketch is parameterized by size k, features. In network monitoring, analyses seek to identify sets of depth s, and width k=s. We initialize with a size-k array set to zero. features (e.g., source/destination IP addresses) that exhibit the most We view this array z as being arranged in s rows, each of width k=s. significant relative differences in occurrence between streams of The high-level idea is that each row of the sketch is a compressed network traffic [6]. In natural language processing on text streams, version of the model weight vector w 2 Rd , where each index several applications require the identification of strongly-associated i 2 [d] is mapped to some assigned bucket j 2 [k=s]. Since k=s ≪ d, groups of tokens [8]; this pertains more broadly to the problem of there will be many collisions between these weights; therefore, we identifying groups of events which tend to co-occur. maintain s rows—each with different assignments of features to These tasks can all be framed as instances of streaming classi- buckets—in order to disambiguate weights. fication between two or more classes of interest, followed by the interpretation of the learned model in order to identify the features Updates. For each feature i, we assign a uniformly random index that are the most discriminative between the classes. Further, as hj (i) in each row j 2 [s] and a uniformly random sign σj (i). Given is the case with classical tasks like identifying frequent items or an update ∆i 2 R to weight wi , we apply the update by adding counting distinct items, these use cases typically allow for some σj (i)∆i index hj (i) of each row j. Denoting this random projection degree of approximation error in the returned results. We are there- by the matrix A 2 f−1;+1gk×d , we can then write the update ∆˜ to fore interested in algorithms that offer similar memory-accuracy z as ∆˜ = A∆. In practice, this assignment is done via hashing. tradeoffs to classical sketching algorithms, but in the context of Instead of being provided the updates ∆, we must compute them online learning for linear classifiers. as a function of the input example (x;y) and the sketch state z. Given the convex and differentiable loss function `, we define the update to z as the online gradient descent update for the sketched example SysML’18, February 2018, Stanford, CA (Ax;y). In particular, we first make a prediction τ = (1=s)zT Ax, 2018. ACM ISBN 978-x-xxxx-xxxx-x/YY/MM...$15.00 https://doi.org/10.1145/nnnnnnn.nnnnnnn 1The full version of this paper is available at [19]. SysML’18, February 2018, Stanford, CA Tai et al. RCV1 ( = 10 6) URL ( = 10 5) Algorithm 1: Weight-Median (WM) Sketch 1.25 1.25 input: size k, depth s, loss function `, `2-regularization 1.20 1.20 Trun parameter λ, learning rate schedule η PTrun t 1.15 1.15 SS initialization 1.10 1.10 Hash z s × k=s array of zeroes WM relative error 1.05 1.05 AWM Sample Count-Sketch matrix A 1.00 1.00 t 0 0 50 100 0 50 100 function Update(x, y) K K 1 T Figure 2: Relative ` error of estimated top-K weights vs. true τ s z Ax . Prediction for x 2 z (1 − ληt )z − ηtyr` (yτ ) Ax . Update with `2 reg. top-K weights for `2-regularized logistic regression under an t t + 1 8KB memory budget. The AWM-Sketch achieves lower re- function Query(i) covery error across both datasets. return output of Count-Sketch retrieval on z URL identification [13], and the KDD Algebra dataset [18, 23] (see Fig. 2 for results on RCV1 and URL). We compared against several and then compute the gradient update ∆˜ = −ηyr` (yτ ) Ax with memory-budgeted baselines: hard thresholding and a probabilistic learning rate η. variant (Trun, PTrun), tracking frequent features with the Space For intuition, we can compare this update to the Count-Sketch Saving algorithm (SS), and feature hashing (Hash). We found that update rule [4]. In the frequent-items setting, the input x is a one- the AWM-Sketch achieved lower recovery error across all three hot encoding for the observed item. The update to the Count-Sketch baselines. On RCV1, the AWM-Sketch achieved 4× lower error ˜ = state zcs is simply ∆cs Ax, where A is defined identically as above. relative to the baseline of only learning weights for frequently- Therefore, our update rule is simply the Count-Sketch update scaled occurring features. Compared to an uncompressed classifier, the − r` T = by the constant ηy (yz Ax s). However, an important detail to AWM-Sketch uses two orders of magnitude less space at the cost note is that the Count-Sketch update is independent of the sketch of 10% relative error in the recovered weights. In terms of classifi- state zcs, whereas the WM-Sketch update does depend on z. This cation accuracy, the AWM-Sketch with an 8KB budget achieved 1% cyclical dependency between the state and state updates is the main higher error rate than an uncompressed classifier on RCV1, while challenge in our analysis of the WM-Sketch. outperforming all the baseline methods by at least 1%. Queries. To obtain an estimate wˆi of the ith weight, we return the Application: Network Monitoring. IP network monitoring is median of the values σ (i) · z for j 2 [s]. This is identical to j j;hj (i) one of the primary application domains for sketches and other the query procedure for the Count-Sketch. small-space summary methods [2, 20, 24]. Here, we focus on finding Analysis. We show that for feature dimension d and with success source/destination IP addresses that differ significantly in relative probability 1 − δ, we can learn a compressed model of dimension frequency between a pair of network links [6]. The AWM-Sketch O ϵ−4 log3(d=δ ) that supports approximate recovery of the opti- significantly outperformed a baseline using a pair of Count-Min mal weight vector w∗ over all the examples seen so far, where the sketches by a factor of over 4× in recall while using the same absolute error of each weight estimate is bounded above by ϵ kw∗ k1. memory budget (32KB). These results indicate that linear classifiers For a given input vector x, this structure can be updated in time can be used effectively to identify significant relative differences O ϵ−2 log2(d=δ ) · nnz(x) . For formal statements of our theorems, over pairs of data streams. proofs, and additional discussion of the conditions under which 4 DISCUSSION AND RELATED WORK this result holds, see the full version of the paper [19]. Sparsity-Inducing Regularization. ` -regularization is a stan- Active-Set Weight-Median Sketch (AWM-Sketch).
Details
-
File Typepdf
-
Upload Time-
-
Content LanguagesEnglish
-
Upload UserAnonymous/Not logged-in
-
File Pages3 Page
-
File Size-