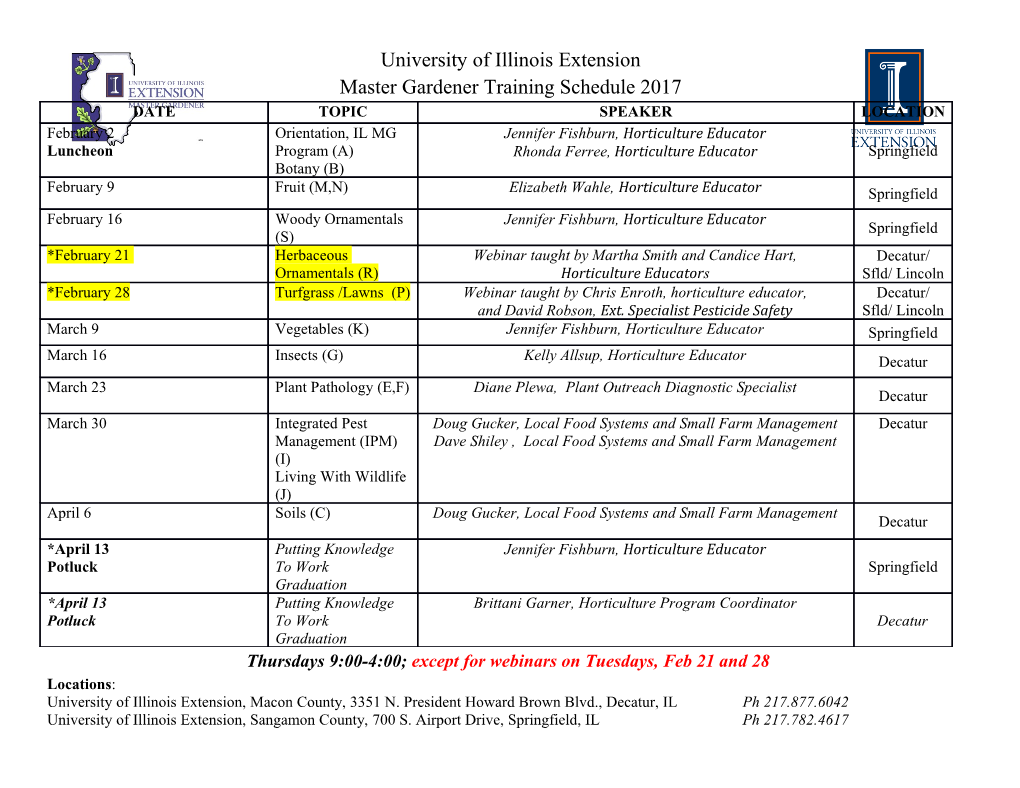
Ethics in Artificial Intelligence: the Why Case Study Analyses and Proposed Solutions Emma Beharry Hari Bhimaraju Meghna Gite Elena Lukac Anika Puri Mana Vale Ecem Yilmazhaliloglu, Students of AI4ALL Artificial Intelligence is the future. This appears again and again in the news, at our schools, during conversations, but what percentage of the general public—and especially the youth—truly understands why? We hail from a variety of schools, countries, and backgrounds, but we are all united by our passion for Artificial Intelligence. We attended nonprofit AI4ALL’s summer educational programs, where we had the opportunity to explore an introduction to AI alongside like-minded female students. Through topic-specific lectures that taught basic AI algorithms (i.e. K-Nearest Neighbors, Decision Trees, Naïve Bayes, etc.), interdisciplinary AI research introductions from distinguished professors and industry leaders, hands-on project groups, and application-oriented lab visits, we gained an incredibly thorough exposure to all facets of the field. Our goal in writing this paper is to share our experiences as young researchers to offer our perspective on this question. There are two main questions in AI as we approach a future straight from our imagination: how and why. The former is what a majority of the scientific and technological communities are focused on. How can we use AI to create autonomous vehicles? How can doctors employ AI to transform medicine from a curative field to a predictive and preventive one? How can computer vision convert our excess of big data into systems for disaster relief or humanoid robots? With all the excitement surrounding novel technologies and the seemingly infinite potential of AI, the question of why often takes the backseat. Why are we putting so many resources into AI without truly understanding its full potential? Why are there not governmental or universal regulations on AI? Why are more people not thinking about AI’s potential failures? These questions bring in ethics: what is and will be ethical in AI? Our research paper takes the format of problem-example, case study-potential solution analyses on prominent topics in AI: Natural Language Processing, Facial Recognition, Computer Vision, Data Collection, and Gun Violence. We selected key problems that we have identified in AI today and studied popular examples of these shortcoming, discussing their ethical implications. Through our paper, which is geared towards the common public, we hope to increase AI education, literacy, and youth involvement, not just in the technological aspects of AI but in all its intersecting fields: policy, education, business, and more. AI4ALL’s motto is as follows: AI will change the world. Who will change AI? As you read this paper, keep this question in mind. 100% of the authors of this paper identify as female. 12% of AI researchers around the world follow the same demographic. This is a problem. Why is dataset bias so prominent in AI? One of the main reasons is lack of diversity in researchers creating datasets. This and other issues that plague Artificial Intelligence today are caused by the homogeneity in the field. We hope that this paper will serve as a positive step towards increased diversity in AI. We hope that it will bring the why to the forefront of the AI revolution. Natural Language Processing Natural Language Processing (NLP) is the subsection of artificial intelligence technology that concerns the natural human language. NLP systems aim to process, understand, and generate human language. Applications of NLP systems are ubiquitous in daily American life, from translation apps (i.e. Google Translate) to grammatical correction technologies (i.e. auto-correct). The variety of NLP algorithms has allowed the existence and development of these applications for decades. The simplest machine learning NLP algorithm is Naïve Bayes, which calculates the conditional probability that a word or phrase has a certain meaning or is in a certain category, based upon the dataset. The Neural Networks family of machine learning algorithms has a varying range of complexity, ranging from basic Neural Networks to Deep Convolutional Neural Networks to Generative Adversarial Networks and more. Neural Networks are inspired by the human brain, and pass inputs through layers of mathematical calculations to produce an output. Lastly, the newest widely adopted NLP algorithms—word2vec, bag of words, term frequency-inverse document frequency, etc.—all involve storing words in multi-dimensional vectors, or 1xn matrices. The computer creates a mathematical understanding or definition of the word through different matrix calculations depending on the algorithm, which it then uses to create relationships between words. Despite advances in technology, NLP algorithms are susceptible to dataset biases because they are generally supervised machine learning algorithms. All machine learning algorithms require a training dataset to observe and test predictions with. Supervised machine learning means the dataset is labelled and annotated, or it has the “correct answers”—the algorithm knows what the answers should be and must figure out how to achieve those answers. If the dataset involves language that is gendered, racist, and/or generally demeaning, the algorithm will adopt that language as its default goal or “correct answer,” encoding biases. Even less explicitly malign dataset biases can reveal inequities. In the Stanford AI4ALL 2019 Camp, a group of students programmed a Naïve Bayes System that classified texts and tweets from Hurricane Sandy and the 2010 Haitian Earthquake, respectively, into 5 categories of relief: food, water, medical, energy, or none. The geographical differences between New Jersey and New York and Haiti, and the different types of damage incurred, skewed the global dataset. Figure 1 – Hurricane Sandy Dataset Composition Figure 2 – 2010 Haitian Earthquake Dataset Composition Although biases like requests for different necessities seem inherently benign, an investigation into their creation can provide proof or evidence of inequities. For example, the common demands in New York and New Jersey were for generators, hand-crank devices, and batteries. However, it was hypothesized that because such energy resources were not widely available in the less affluent Haiti, the demand for energy materials was drastically less. In addition, more immediate actions can skew the dataset. For example, the Haitian texts were translated into English to create the algorithm, meaning nuances of questions were inevitably lost. This was hypothesized to be a big contributor as to why over one-third of requests to a post-earthquake hotline were labelled as “None.” In addition, the primary training set employed was the Hurricane Sandy Dataset. Thus, when evaluating the performance of the Naïve Bayes Algorithm, performance on the Hurricane Sandy Testing Set (unlabeled data the computer had not yet seen) was higher than the Haitian Earthquake Testing Set. The computer was unable to recognize Haitian requests for energy supplies and had a lower F1 score, the metric used to evaluate the performance of the algorithm. Figure 3 – NLP Performance by Category on Hurricane Sandy Testing Set Figure 4 – NLP Performance by Category on 2010 Haitian Earthquake Testing Set The overuse of the Hurricane Sandy training set had intuitively biased the algorithm to perform better with Hurricane Sandy data. This bias towards one dataset comes at the expense of performance on the other, and creates an algorithm less capable of providing relief to an entire population. The algorithm will not be used in real-world scenarios in the near future. Yet, if it had, it would have had lower performance analyzing data from Haiti than data New England. This disparity was not intentional, yet it could have cost lives in delivering crucial disaster relief. Biases are almost always a product of humans. They can originate at every step of the creation of an NLP algorithm, from the selection of data, the preparation of data, the creation of code, and the training of the algorithm. Whether intentional or not, biased missteps create algorithms with marginalizing biases toward certain demographics that will manifest during use. As NLP develops, and algorithms become increasingly capable of generating human language, the notion of a biased algorithm generating racist, gendered, or demeaning language during use, or harboring a bias towards a demographic—without the intention of the computer scientist—is a sobering thought. When considering how to solve the issue of bias in NLP, there is a major caveat. It is impossible to claim and prove that each and every bias is malign and must be eliminated. Additionally, it is impossible to eliminate every bias from existence; there is a typically entrenched force, institution, or structure producing that bias. In large, biases are largely malign and in practice have contributed to more harm that good. Thus, computer scientists should take extreme care and caution when inevitably producing A.I. We have outlined a general guideline for computer scientists to follow when assessing or encountering bias during any stage of the algorithm development cycle. Guidelines: 1. Assess possible biases that could occur in the dataset and why 2. Determine how malign the possible biases are on a scale of 1-5. a. Scale. For clarification, being “completely” benign/malign means that the bias is inherently benign/malign and has a benign/malign impact on algorithm performance. The “3” category is for biases that are not overtly benign or malign but have an impact that skews the dataset in a distinct way. i. 1 is completely benign ii. 2 is benign impact on performance iii. 3 is skews dataset with tangible impact iv. 4 is malign impact on performance v. 5 is completely malign b. Evaluation Metrics. When giving a number on the scale to a bias, one should look at the bias’s i. Inherent malign/benign standing (reason for existence) ii. Impact on algorithm performance iii. Real-world Impact 3. Determine the desirability of minimizing biases.
Details
-
File Typepdf
-
Upload Time-
-
Content LanguagesEnglish
-
Upload UserAnonymous/Not logged-in
-
File Pages26 Page
-
File Size-