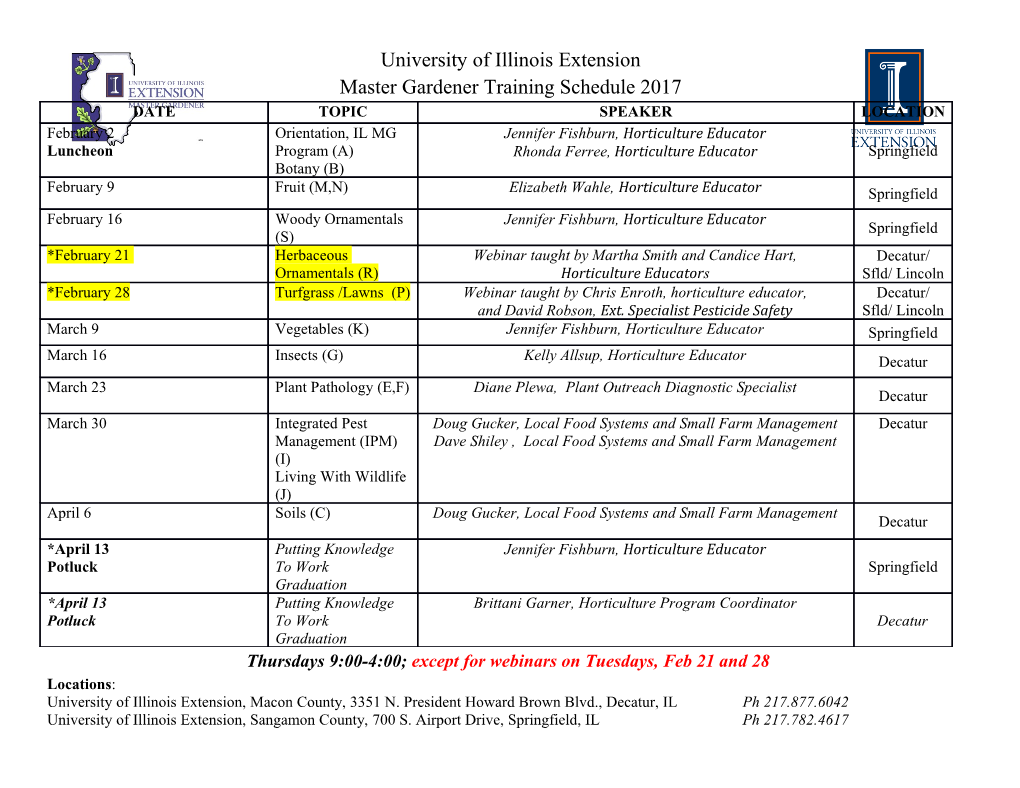
Deep Learning: Enhancing the Security of Software-Defined Networks Ahmed Dawoud M.Sc. Software Development & M.Sc. Information and Communication Technologies A thesis submitted for the degree of Doctor of Philosophy School of Computing, Engineering and Mathematics Western Sydney University June 2019 Abstract Software-defined networking (SDN) is a communication paradigm that promotes network flexibility and programmability by separating the control plane from the data plane. SDN consolidates the logic of network devices into a single entity known as the controller. SDN raises significant security challenges related to its architecture and associated characteristics such as programmability and centralisation. Notably, security flaws pose a risk to controller integrity, confidentiality and availability. The SDN model introduces separation of the forwarding and control planes. It detaches the control logic from switching and routing devices, forming a central plane or network controller that facilitates communications between applications and devices. The architecture enhances network resilience, simplifies management procedures and supports network policy enforcement. However, it is vulnerable to new attack vectors that can target the controller. Current security solutions rely on traditional measures such as firewalls or intrusion detection systems (IDS). An IDS can use two different approaches: signature-based or anomaly-based detection. The signature-based approach is incapable of detecting zero-day attacks, while anomaly-based detection has high false-positive and false-negative alarm rates. Inaccuracies related to false-positive attacks may have significant consequences, specifically from threats that target the controller. Thus, improving the accuracy of the IDS will enhance controller security and, subsequently, SDN security. A centralised network entity that controls the entire network is a primary target for intruders. The controller is located at a central point between the applications and the data plane and has two interfaces for plane communications, known as northbound and southbound, respectively. Communications between the controller, the application and data planes are prone to various types of attacks, such as eavesdropping and tampering. The controller software is vulnerable to attacks such as buffer and stack overflow, which enable remote code execution that can result in attackers taking control of the entire network. Additionally, traditional network attacks are more destructive. This thesis introduces a threat detection approach aimed at improving the accuracy and efficiency of the IDS, which is essential for controller security. To evaluate the 1 effectiveness of the proposed framework, an empirical study of SDN controller security was conducted to identify, formalise and quantify security concerns related to SDN architecture. The study explored the threats related to SDN architecture, specifically threats originating from the existence of the control plane. The framework comprises two stages, involving the use of deep learning (DL) algorithms and clustering algorithms, respectively. DL algorithms were used to reduce the dimensionality of inputs, which were forwarded to clustering algorithms in the second stage. Features were compressed to a single value, simplifying and improving the performance of the clustering algorithm. Rather than using the output of the neural network, the framework presented a unique technique for dimensionality reduction that used a single value—reconstruction error—for the entire input record. The use of a DL algorithm in the pre-training stage contributed to solving the problem of dimensionality related to k-means clustering. Using unsupervised algorithms facilitated the discovery of new attacks. Further, this study compares generative energy-based models (restricted Boltzmann machines) with non-probabilistic models (autoencoders). The study implements TensorFlow in four scenarios. Simulation results were statistically analysed using a confusion matrix, which was evaluated and compared with similar related works. The proposed framework, which was adapted from existing similar approaches, resulted in promising outcomes and may provide a robust prospect for deployment in modern threat detection systems in SDN. The framework was implemented using TensorFlow and was benchmarked to the KDD99 dataset. Simulation results showed that the use of the DL algorithm to reduce dimensionality significantly improved detection accuracy and reduced false-positive and false-negative alarm rates. Extensive simulation studies on benchmark tasks demonstrated that the proposed framework consistently outperforms all competing approaches. This improvement is a further step towards the development of a reliable IDS to enhance the security of SDN controllers. 2 Declaration of Original Work I certify that this thesis does not incorporate without acknowledgement any material previously submitted for a degree or diploma in any university and that to the best of my knowledge and belief it does not contain any material previously published or written by another person, except where due reference is made in the text. Signe _____/____/_____28 11 2019 3 Acknowledgements First and foremost I want to express my gratitude and appreciations to my supervisor Dr. Seyed Shahrestani for his guidance, support, and motivation during my prolonged PhD journey. I appreciate all his contributions of time, ideas and expertise to make this work possible. I would like to express my special appreciation and thanks to Dr. Chun Raun, for her patient listening and vital comments. Also, it is inevitable to express my thanks to graduate research school administrative staff for their continuous and prompt support. During the long and intense years of my studies and research I lost people who shared me the dreams, I would like to honour their memory in this work, to my father and my uncle Ibrahim. A special thanks to my beloved wife Sara, and my sons Hamza and Mohamed without them I would finish this work a year and half earlier. 4 Table of Contents Abstract ............................................................................................................................ 1 Declaration of Original Work ........................................................................................ 3 Acknowledgements .......................................................................................................... 4 Table of Contents ............................................................................................................ 5 List of Figures .................................................................................................................. 8 List of Tables ................................................................................................................. 10 List of Abbreviations .................................................................................................... 11 Publications .................................................................................................................... 13 Chapter 1: Introduction ............................................................................................... 15 1.1 Introduction ........................................................................................................... 15 1.2 Motivation ............................................................................................................. 17 1.3 Research Methodology .......................................................................................... 19 1.4 Research Questions and Scope .............................................................................. 23 1.5 Contributions ......................................................................................................... 23 1.6 Thesis Structure ..................................................................................................... 25 Chapter 2: Software-Defined Networks ...................................................................... 28 2.1 Introduction ........................................................................................................... 28 2.2 Software-Defined Network Architecture .............................................................. 30 2.2.1 Software-Defined Network Controller ........................................................... 31 2.2.2 Controller Anatomy: OpenDaylight ............................................................... 33 2.2.3 OpenFlow Protocol ........................................................................................ 35 2.3 Software-Defined Network Applications .............................................................. 39 2.3.1 Network Traffic Engineering ......................................................................... 39 2.3.2 Network Monitoring ...................................................................................... 40 2.3.3 Software-Defined Networks for Virtualisation .............................................. 41 2.4 Software-Defined Networds in Various Networking Environments .................... 43 2.4.1 Software-Defined Networks for the Internet of Things ................................. 43 2.4.2 Software-Defined Networks for Cloud and Data Centres.............................. 44 2.4.3 Software-Defined Networks for Wireless Networks and 5G ......................... 46 2.5 Software-Defined Network Challenges ...............................................................
Details
-
File Typepdf
-
Upload Time-
-
Content LanguagesEnglish
-
Upload UserAnonymous/Not logged-in
-
File Pages164 Page
-
File Size-