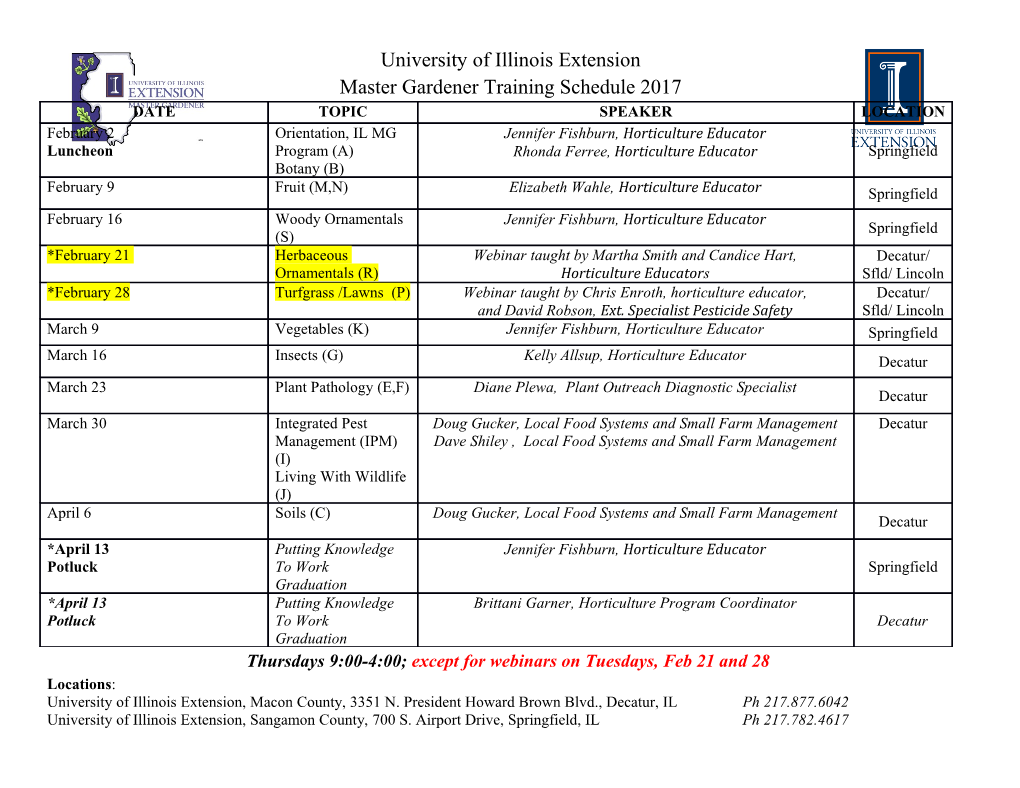
Exploratory Spatial Data Analysis ESDA Luc Anselin University of Illinois, Urbana-Champaign http://www.spacestat.com Outline ESDA Exploring Spatial Patterns Global Spatial Autocorrelation Local Spatial Autocorrelation © 1999-2001, Luc Anselin All Rights Reserved ESDA © 1999-2001, Luc Anselin All Rights Reserved 1 Classes of Spatial Data (Cressie) Point Patterns points on a map Geostatistical Data points as sample locations Lattice/Regional Data polygons or points (centroids) © 1999-2001, Luc Anselin All Rights Reserved Lattice or Regional Data Spatial Process index set D is fixed collection of countably many points in Rd finite, discrete spatial units Data fixed points or discrete locations (regions) » examples: county tax rates, state unemployment Research Question interest focuses on statistical inference patterns, estimation, specification tests © 1999-2001, Luc Anselin All Rights Reserved WV Housing Values (1990) counties, county centroids, Thiessen polygons 2 EDA and Space EDA = Discover Potentially Explicable Patterns (Good) Data Visualization (Buja) Interactive View Manipulation » focusing individual views » linking multiple views » arranging many views No Role for Explicit Treatment of Space © 1999-2001, Luc Anselin All Rights Reserved Exploratory Spatial Data Analysis EDA + Describe Spatial Distributions spatial trends, spatial means Identify Atypical Observations spatial outliers Discover Patterns of Spatial Association spatial clusters Suggest Spatial Regimes spatial non-stationarity © 1999-2001, Luc Anselin All Rights Reserved ESDA Functionality Dynamic Graphics linking and brushing statistical plots and map Visualizing Spatial Distributions spatial box map smoothing rates Visualizing Spatial Autocorrelation spatial lag pie charts and bar charts Moran scatterplot and map, LISA maps © 1999-2001, Luc Anselin All Rights Reserved 3 Exploring Spatial Patterns © 1999-2001, Luc Anselin All Rights Reserved Dynamically Linked Windows Dynamic Graphics different “views” of data: histogram, box plot, scatterplot, list views dynamically linked: click on one, corresponding points (areas) on others highlighted geographic brushing: map as a view of data © 1999-2001, Luc Anselin All Rights Reserved 4 Global Spatial Autocorrelation © 1999-2001, Luc Anselin All Rights Reserved 5 Spatial Association Null Hypothesis: No Spatial Association values observed at a location do not depend on values observed at neighboring locations observed spatial pattern of values is equally likely as any other spatial pattern the location of values may be altered without affecting the information content of the data © 1999-2001, Luc Anselin All Rights Reserved Observed (left) and randomized (right) distribution for Columbus Crime Randomization polyid 1 became 14 polyid 2 became 20 polyid 3 became 48 ... Observed (left) and randomized (right) distribution for Columbus Crime Moran’s I = 0.486 Moran’s I = -0.003 6 Alternative Hypotheses of SA Positive Spatial Association like values tend to cluster in space neighbors are similar Negative Spatial Association neighbors are dissimilar checkerboard pattern © 1999-2001, Luc Anselin All Rights Reserved Moran’s I Spatial Autocorrelation Statistic Moran’s I cross-product statistic Σ Σ Σ 2 I = (N/S0) i j wij.zi.zj / i zi µ= Σ Σ with zi = xi - and S0 = i j wij Inference normal distribution randomization permutation © 1999-2001, Luc Anselin All Rights Reserved Interpretation of Moran’s I Positive Spatial Autocorrelation I > -1/(n-1), or z > 0 spatial clustering of high and/or low values » no distinction between high or low Negative Spatial Autocorrelation I < -1/(n-1), or z < 0 checkerboard pattern, “competition” © 1999-2001, Luc Anselin All Rights Reserved 7 Spatial Lag Chart Spatial Lag Visualization value at i compared to weighted average of neighbors: xi relative to (Wx)i similar values = positive SA dissimilar values = negative SA Spatial Lag Pie Chart xi and (Wx)i as proportions of “pie” (x > 0 only) Spatial Lag Bar Chart xi and (Wx)i as bars © 1999-2001, Luc Anselin All Rights Reserved Ww_hoval Hoval 8 Moran Scatterplot Linear Spatial Association linear association between value at i and weighted average of neighbors: Σ j wij yj vs. yi , or Wy vs y four quadrants » high-high, low-low = spatial clusters » high-low, low-high = spatial outliers Moran’s I slope of linear scatterplot smoother I = z’Wz / z’z © 1999-2001, Luc Anselin All Rights Reserved 9 Use of Moran Scatterplot Classification of Spatial Association Local Nonstationarity outliers high leverage points sensitivity to boundary values Regimes nonlinear association » different slopes in subsets of the data © 1999-2001, Luc Anselin All Rights Reserved 10 Local Spatial Autocorrelation © 1999-2001, Luc Anselin All Rights Reserved LISA Definition (Anselin 1995) Local Indicators of Spatial Association LISA satisfies two requirements indicate significant spatial clustering for each location sum of LISA proportional to a global indicator of spatial association LISA Forms of Global Statistics local Moran, local Geary, local Gamma © 1999-2001, Luc Anselin All Rights Reserved Use of LISA Identify Hot Spots significant local clusters in the absence of global association significant local outliers » high surrounded by low and vice versa Indicate Local Instability local deviations from global pattern of spatial association © 1999-2001, Luc Anselin All Rights Reserved 11 Local Moran Local Moran Statistic Σ Ii = (zi/ m2) j wij.zj Σ i Ii = N.I Inference randomization assumption conditional permutation local dependence or heterogeneity? Visualization LISA map and Moran Significance Map © 1999-2001, Luc Anselin All Rights Reserved 12.
Details
-
File Typepdf
-
Upload Time-
-
Content LanguagesEnglish
-
Upload UserAnonymous/Not logged-in
-
File Pages12 Page
-
File Size-