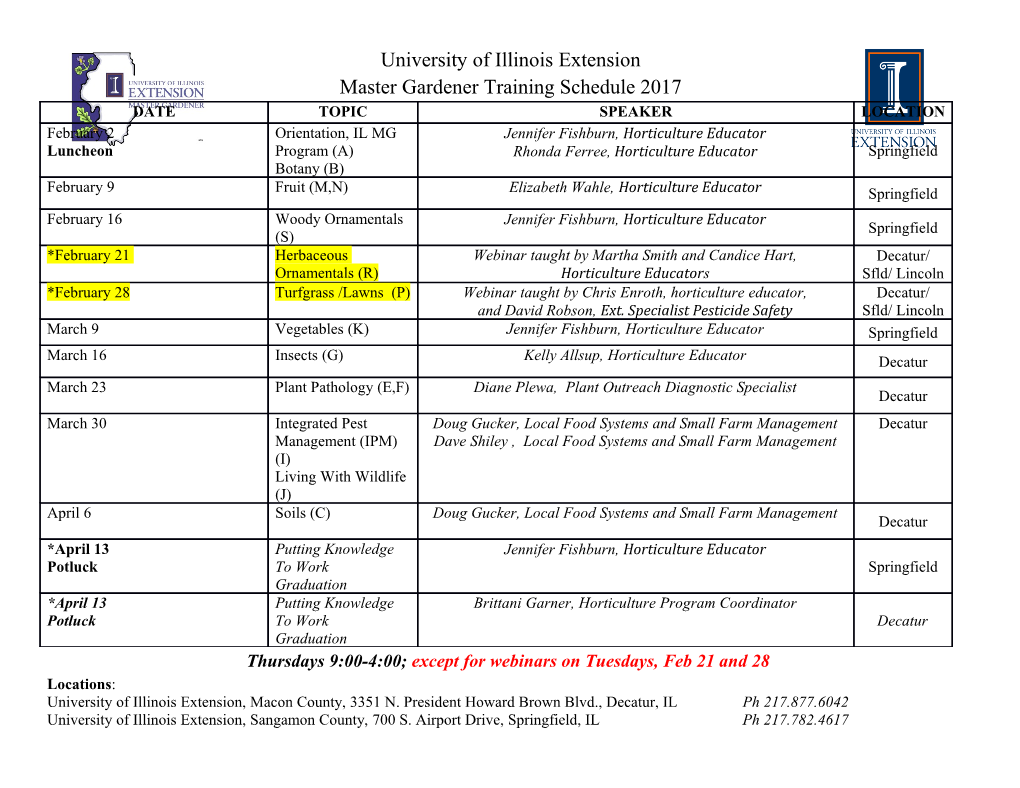
IC 1407 ACCREDIT STOCHASTIC SIGNAL PROCESSING Prof., Dr. Yury Kuznetsov Moscow Aviation Institute (National Research University) Russian Federation COST Training School: EMC for Emerging Technologies Department of Physics - Faculty of Science University of Malta MOSCOW 19.04.2018 AVIATION INSTITUTE Contents Motivation and the theory of statistical signal processing (SP) Non-stationary Stationary Cyclostationary Characterization of measurement data and localization of the spatial distribution Example: Intel Galileo Board Separation of two distinct cyclostationary sources and localization of their spatial distributions Example: Artix Training Board MOSCOW AVIATION INSTITUTE 2 Motivation of statistical SP Electromagnetic field (EMF) emitted by the printed circuit board (PCB) contains a stochastic component sensing by the near-field scanning probe and registering by the real-time digital oscilloscope Statistical signal processing can be used for the characterization of the received stochastic EMF and for the localization and identification of the radiating sources The measured stochastic signals contain the superposition of different radiating sources located in the environment of the DUT: emissions from transmission lines transferring the data sequences between distinct blocks of the DUT; thermal noise and interference from surrounding radiations MOSCOW AVIATION INSTITUTE 3 Classification of random processes Random process Time sequence of random The set of random signals variables = , : Ω , = , = 1, is an elementary ∈ event ∈ ℝ Ω is a sample space is a random variable at = is a set of real numbers The stochastic phenomena can be mathematically characterized by the model of random ℝ process constructed from the measured random signals The random variable is fully described by the probability density function for each time t = ti Random signal is a time domain realization of the stochastic phenomena for each event Ω MOSCOW ∈ AVIATION INSTITUTE 4 Cumulative Distribution Function The cumulative distribution function of the stochastic process is: , = , = : , d = : , Ω ≤ ≤ ≤1, : �, indicator function = : , 0, : , > ≤ ≤ � is the operator of statistical expectation or ensemble averaging1 using Lebesgue integral � The expected value of the stochastic process is the statistical mean = , = , d = d , Ω � �ℝ 1 MOSCOW A. Napolitano, Generalizations of Cyclostationary Signal Processing: Spectral Analysis and AVIATION Applications INSTITUTE . John Wiley & Sons Ltd - IEEE Press, 2012.5 Second-Order Characterization The second-order joint cumulative distribution function of the stochastic process is: , ; , = , , , = : , : , 1 2 1 2 1 ≤ 1 2 ≤ 2 1 ≤1 2 ≤2 The autocorrelation function of the stochastic process is: , = , + , = d , ; , + 2 1 2 1 2 ℛ �ℝ The autocovariance of the stochastic process is its autocorrelation function of the zero-mean process: , = , + , + − − MOSCOW AVIATION INSTITUTE 6 Spectral Characterization The second order stochastic process is harmonizable and can be characterized in the frequency domain if its autocorrelation function can be expressed by the Fourier-Stieltjes integral: , , = d , 2 11+22 where , is a spectral1 correlation2 function2 with bounded variation. 1 2 It is also known that a �ℝ stochastic process is harmonizable if and only if its covariance function is harmonizable 1 2 The Fourier transform of the realization , of the harmonizable stochastic process can be expressed as: , = , d −2 , ̂ where can contain Dirac delta functions�ℝ ̂ MOSCOW AVIATION INSTITUTE 7 Time-Frequency Relation The spectral correlation function (Loève bifrequency spectrum) of the harmonizable stochastic process is defined as: , = , , 1 2 ̂ 1 ̂ 2 The relation between the autocorrelation function and the spectral correlation function is defined by two-dimensional Fourier transform: = , d d 2 11+22 1 2 � 2 1 2 1 2 , = ℝ d d −2 11+22 1 2 2 1 2 1 2 �ℝ MOSCOW AVIATION INSTITUTE 8 Time-Variant Spectrum The time-variant spectrum of the stochastic process is the Fourier transform of the autocorrelation function with respect to the lag parameter = : 2 1 , = , d − −2 �ℝ ℛ By introducing the variables = + /2 and = /2 it can be obtained a time- frequency representation in terms of Wigner-Ville spectrum for stochastic processes: 1 2 − , = + /2 /2 d = + /2 /2 d −2 −2 ̂ ̂ �ℝ − �ℝ − MOSCOW AVIATION INSTITUTE 9 Characteristics of RP Distribution of the probability over RVs of the random process Ensemble averaging of the random process by using expectation operator Statistical mean and 2D-autocorrelation function in time domain Spectral correlation function in bi-frequency domain Time-variant and Wigner-Ville spectra in time-frequency domain Probabilistic approach is more theoretical then practical MOSCOW AVIATION INSTITUTE 10 Stationary process Second-order wide-sense stationary process (WSS) can be characterized by an autocorrelation function (ACF) and a power spectral density linked by the Wiener-Khinchin relation , = = d 2 ℛ � , = = ℝ d −2 �ℝ Due to the dependency of the ACF for the WSS process only from = , the spectral correlation function , can be non-zero only for = 2 − 1 1 2 1 2 = , d d =− , d d 2 11+2 1− 21 1 1 2 1 2 1 2 2 1 1 1 2 − �ℝ �ℝ − MOSCOW AVIATION INSTITUTE 11 Stationary process Random process realization 0 Autocorrelation function 1/ 0 1/ − Power spectral density 0 MOSCOW AVIATION INSTITUTE 12 − 2 2 Discrete-time random process Discrete time process can be analyzed as the sampled continuous time realizations of the stochastic processes: = = = ∞ ∆ � − where is a sample interval. The Discrete Time Fourier=− ∞Transform (DTFT) of the realization of the harmonizable discrete stochastic process can be expressed as: ∆ = ∞ −2 = ̂ � where is a normalizes frequency. The inverse=−∞ DTFT gives the initial realization of the discretized stochastic process: ∆ / = 1 2 / 2 MOSCOW � ̂ AVIATION −1 2 INSTITUTE 13 Discrete-time stationary process Discrete time process Discrete autocorrelation function [ ] 1 0 1 Periodical −power spectral density MOSCOW 2 1 0 1 2 AVIATION INSTITUTE 14 − − Characteristics of Discrete random process The second order discretized stochastic process is harmonizable and can be characterized in the frequency domain if its autocorrelation function can be expressed by the Fourier-Stieltjes integral: = d , / , / 2 11+22 1 2 2 1 2 The spectral correlation function (Loève� bifrequency−1 2 1 2 spectrum) of the harmonizable discretized stochastic process is defined as: , = The relation between the autocorrelation 1 function2 and̂ the1 spectral̂ 2 correlation function is defined by two-dimensional DTFT: = , d d / , / 2 11+22 1 2 2 1 2 1 2 �−1 2 1 2 , = ∞ ∞ −2 11+22 MOSCOW 1 2 1 2 AVIATION � � 15 INSTITUTE 1=−∞ 2=−∞ Characteristics of stationary RP Distribution of the probability over RVs of the random process doesn’t depend of time Ensemble averaging of the random process by using expectation operator Statistical mean and autocorrelation function doesn’t depend of time Spectral correlation function in a Fourier transform of the ACF Discretization of the stationary RP gives the periodic PSD and discrete ACF MOSCOW AVIATION INSTITUTE 16 Cyclostationary random process The cyclostationary random process is a non-stationary stochastic process whose statistical properties are periodically vary with respect to time. The period is called a cycle, and its inverse = 1/ is a cyclic frequency. More generally, the process is almost-cyclostationary (ACS) if its statistical properties can 0 0 be represented by a superposition of periodic functions with distinct cyclic frequencies . The autocorrelation function of the ACS stochastic process posses the periodicity in time and∈ can be expressed by the Fourier series expansion1: , = + = , � where is the operatorℛ of ensemble averaging: � ∈ 1 � + = lim + + + + 1 0 0 0 The Fourier coefficients of , are called→∞ cyclic autocorrelation� functions and can be defined by a 2 =− time domain cyclic averaging for each known cyclic frequency: ℛ 1 / , = lim , d 2 / −� 1 W. A. Gardner, Introduction to Random→∞ Processes� ℛwith Applications to Signals and Systems. MOSCOW − 2 AVIATION INSTITUTE Macmillan, New York, 1985 (2nd Edition McGraw17 -Hill, New York, 1990). Pulse Amplitude Modulation Pulse amplitude modulated (PAM) signal / 0 1 2 3 4 5 6 7 8 9 ∆ Autocorrelation function Cyclic autocorrelation function , , / 1 0 1 / 2 5 ∆ 4 − ∆ − 1 − 3 − 2 − 0 − 1 − 0 1 − 1 2 2 3 4 3 MOSCOW 5 AVIATION 1 0 1 INSTITUTE / 18 − ⋅ ∆ ∆ Cyclic correlation function The magnitude and phase of , represent amplitude and phase of the additive complex harmonic component at frequency for time lag contained in the autocorrelation function of the ACS stochastic process , . For = 0 the cyclic autocorrelation function reduces to the autocorrelation function of the stationary random process . ℛ For a zero-mean stochastic process = = 0 the magnitude of the cyclic autocorrelation functions , 0 as . If the mean function of the stochastic process = , 0, then some , contain additive sinusoidal functions of , which arise from the products of sinusoidal terms in . Such → ACS processes → ∞ are called unpure and need to be processed accounting
Details
-
File Typepdf
-
Upload Time-
-
Content LanguagesEnglish
-
Upload UserAnonymous/Not logged-in
-
File Pages52 Page
-
File Size-