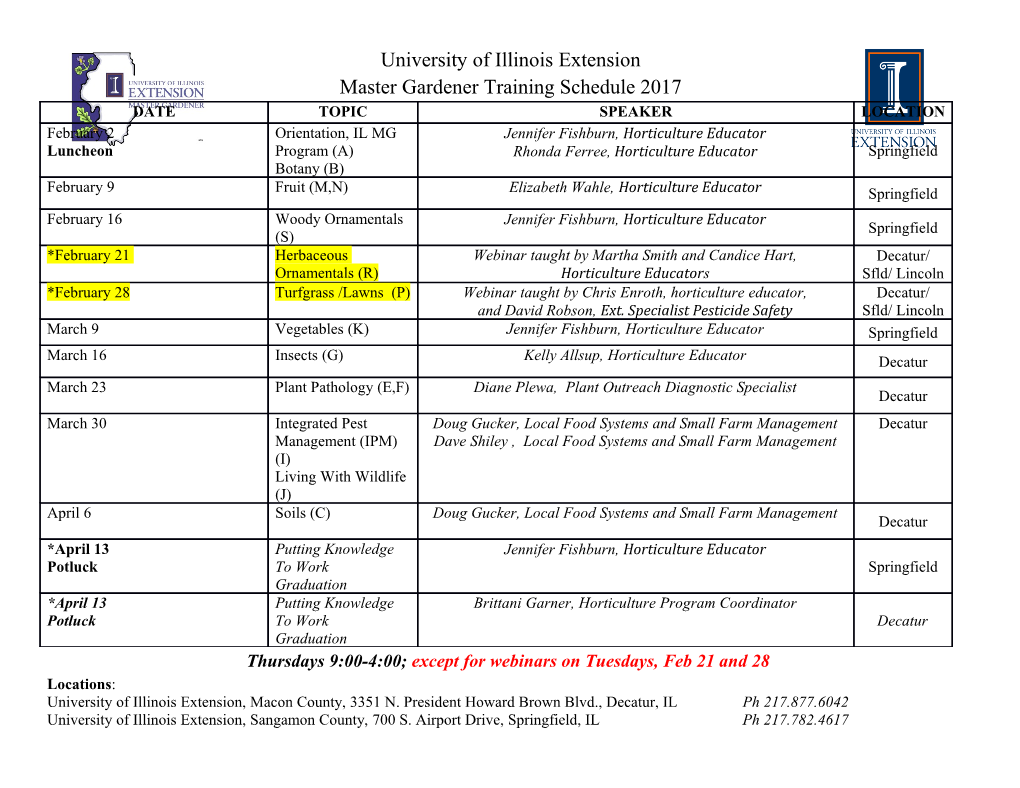
A Simple Example to Illustrate the Linkage Principle Daniel Quint∗ University of Wisconsin April 2016 Abstract. I present a numerical example illustrating the revenue-superiority of an \open" over a \closed" auction format (the linkage principle), and use it to calculate the magnitude of the effect. 1 Introduction One of the classic results of Milgrom and Weber's (1982) seminal paper is that in a symmetric, affiliated setting, an ascending auction will yield higher expected revenue if bidders are aware at each point during the auction of how many of their competitors are still bidding. This result { one of a set of related insights often collectively labeled the \linkage principle" { is well known: Loertscher, Marx and Wilkening (2015), for example, quote FCC economist Evan Kwerel (in the introduction to Milgrom (2004)) as citing it as a key consideration in the design of early U.S. spectrum auctions. However, the mechanism by which it operates is a bit subtle, and I can't recall ever seeing an explicit example illustrating it, or calculating its magnitude. Here, I offer one. 2 Model 2.1 Valuations Let N be the number of bidders. Suppose there's an underlying state θ 2 f0; 1g, equally likely, with common values vi = θ. Each bidder gets a signal si which is i:i:d: conditional on θ, with density ( αsα−1 if θ = 1 f(sjθ) = α(1 − s)α−1 if θ = 0 and therefore CDF ( sα if θ = 1 F (sjθ) = 1 − (1 − s)α if θ = 0 ∗Department of Economics, University of Wisconsin. 1180 Observatory Drive, Madison, WI 53706, USA. Tel.: (608) 263-2515. E-mail: [email protected]. Parts of this work were done while I was a visitor at eBay Research Labs in San Jose, CA, and at the European University Institute in Florence, Italy. I'm thankful to ERL and EUI for the support, and to Dominic Coey, Brad Larsen, and Kane Sweeney for inspiring this note and for helpful comments. 1 conditional on the true state, with α > 1. Note that an increase in α makes signals \more infor- mative" about the realization of θ. While it's not immediately apparent, since f(sjθ) satisfies the 1 (1) (2) (3) (N) monotone likelihood ratio property, fθ; s1; : : : ; sN g are affiliated. Let s > s > s > ··· > s denote the order statistics of the bidders' signals. 2.2 Full-information versus no-information button auctions As in Milgrom and Weber (1982), I model ascending auctions as button auctions, with either full information (every bidder knows who else is active and at what price the inactive bidders dropped out) or no information (bidders receive no feedback other than that the auction is still in progress until it is over). Milgrom and Weber refer to the former as an English auction, and the latter as a second-price auction. Symmetric, monotonic equilibria for both are established in Theorems 6 and 10 of their paper, and the revenue ranking result is Theorem 11. 2.3 Bidding in the no-information case When there is no feedback during the auction, each bidder bids the expected value the asset would have if he was exactly tied for the highest signal, or b(s) = Pr(θ = 1js(1) = s(2) = s) By Bayes' Law, this is 1 Pr(s(1) = s(2) = sjθ = 1) b(s) = 2 1 (1) (2) 1 (1) (2) 2 Pr(s = s = sjθ = 1) + 2 Pr(s = s = sjθ = 0) 1 N(N − 1)αsα−1 · αsα−1 · (sα)N−2 = 2 1 α−1 α−1 α N−2 1 α−1 α−1 α N−2 2 N(N − 1)αs · αs · (s ) + 2 N(N − 1)α(1 − s) · α(1 − s) · (1 − (1 − s) ) , ! (1 − s)α−1 (1 − s)α−1 1 − (1 − s)α N−2 = 1 1 + · · sα−1 sα−1 sα 2.4 Bidding in the full-information case In the full-information case, each losing bidder's signal is revealed by the price at which he drops out. Revenue depends on the bid function when only two active bidders remain, which depends on the revealed signals of the N − 2 bidders who have dropped out to that point. I write this bid (3) function as b(s; t), where t = (t3; t4; : : : ; tN ) are the revealed realizations of the order statistics s , 1 Thus, if we think of bidder values as being vi = E(θjfs1; : : : ; sng) instead of vi = θ, this example fits within the general affiliated/interdependent model of Milgrom and Weber (1982). 2 s(4), etc. This bid function is b(s; t) = Pr(θ = 1js(1) = s(2) = s; s(3−N) = t) , α−1 α−1 N α−1 ! (1 − s) (1 − s) Y (1 − ti) = 1 1 + · · sα−1 sα−1 tα−1 i=3 i 3 Results (2) 1 3.1 Example: expected revenue when N = 3, α = 2, and s = 2 1 For an example we can solve analytically, let N = 3, α = 2, and consider the realization 2 of the (2) 1 1 (2) 1 second-highest signal. Note that E(θjs = 2 ) = 2 , as s = 2 is equally likely under either state. (2) 1 In the no-information case, when s = 2 , the second-highest bidder drops out at a bid of , 1 ! 1 1 1 1 − 4 b( 2 ) = 1 1 + · · 1 = 0:25 1 1 4 so revenue is 0:25. In the full-information case, given the realized value of the lowest signal, the 1 bidder with type 2 bids up to , ! 1 1 1 − s(3) b( 1 ; s(3)) = 1 1 + · · = s(3) 2 1 1 s(3) (2) 1 (3) (2) 1 so expected revenue when s = 2 is E(s js = 2 ). Now, conditional on the realizations of θ (2) (3) and s , the distribution of s is just the marginal (prior) distribution of a signal si, conditional on θ, truncated above at s(2); so we can calculate expected revenue as 1 (3) (2) 1 (3) (2) 1 E b 2 ; s js = 2 = E s js = 2 (2) 1 (3) (2) 1 = Pr(θ = 1js = 2 ) · E(s js = 2 ; θ = 1) (2) 1 (3) (2) 1 + Pr(θ = 0js = 2 ) · E(s js = 2 ; θ = 0) 1 1 R 2 R 2 1 0 (2s)sds 1 0 2(1 − s)sds = · 1 + · 1 2 R 2 2 R 2 0 2sds 0 2(1 − s)ds 1 1 1 2 5 = · + · = ≈ 0:2778 2 3 2 9 18 So for this particular realization of s(2), expected revenue is 11% higher when the bidders learn the realization of s(3) from equilibrium bidding than when they do not. 3 3.2 What's going on To avoid ex post regret, the second-to-last bidder must give up before he would regret winning the item if his opponent dropped out. This therefore stops him from bidding beyond the expected (1) (2) 1 value of θ conditional on s = s = 2 , and whatever other information he has. If the signal of the lowest signal was observed, then its realization is also used to update the expected value of θ. If not, then it is not; by iterated expectations, this is the same, in expectation, as using the (3) (1) (2) 1 distribution of s that would be consistent with s = s = 2 . But since this is pessimistic (1) (2) 1 relative to the truth s > s = 2 , and since the signals are affiliated, this depresses the expected value of θ and therefore the second-highest bidder's bid. (2) 1 In numbers, as noted above, expected revenue with full information when s = 2 is (3) (2) 1 (2) 1 (3) (2) 1 E(s js = 2 ) = Pr(θ = 1js = 2 )E(s jθ = 1; s = 2 ) (2) 1 (3) (2) 1 + Pr(θ = 0js = 2 )E(s jθ = 0; s = 2 ) 1 1 1 2 5 = · + · = ; 2 3 2 9 18 while with no information, it's (1) (2) 1 (1) (2) 1 (3) E(θjs = s = ) = E (3) (1) (2) 1 E(θjs = s = ; s ) 2 s js =s = 2 2 (3) (1) (2) 1 = E(s js = s = 2 ) (1) (2) 1 (3) (1) (2) 1 = Pr(θ = 1js = s = 2 )E(s jθ = 1; s = s = 2 ) (1) (2) 1 (3) (1) (2) 1 + Pr(θ = 0js = s = 2 )E(s jθ = 0; s = s = 2 ) 1 1 3 2 1 = · + · = : 4 3 4 9 4 3.3 Ex ante expected revenue While that explains the mechanism through which this effect operates, in terms of magnitude, what we want is not revenue for a particular realization of signals, but expected revenue ex ante. For various values of α and N, I've calculated ex ante expected revenue for the two formats via numerical simulation, simulating 500,000 sets of signals and the resulting auction outcomes. Table 1 gives expected revenue (multiplied by 1; 000 for easier reading); keep in mind the expected value 1 of the asset is exactly 2 , so 500 minus revenue gives bidder surplus. Note that revenue goes to 500, regardless of N, as α approaches either 1 or 1. As α ! 1, the distributions F (sijθ = 1) and F (sijθ = 0) approach each other, so the signals become uninformative; 1 bidders then share the common belief that E(θ) = 2 and earn no information rents. When α gets large, signals are very informative, but therefore also highly correlated, so information rents once again go to 0.
Details
-
File Typepdf
-
Upload Time-
-
Content LanguagesEnglish
-
Upload UserAnonymous/Not logged-in
-
File Pages6 Page
-
File Size-