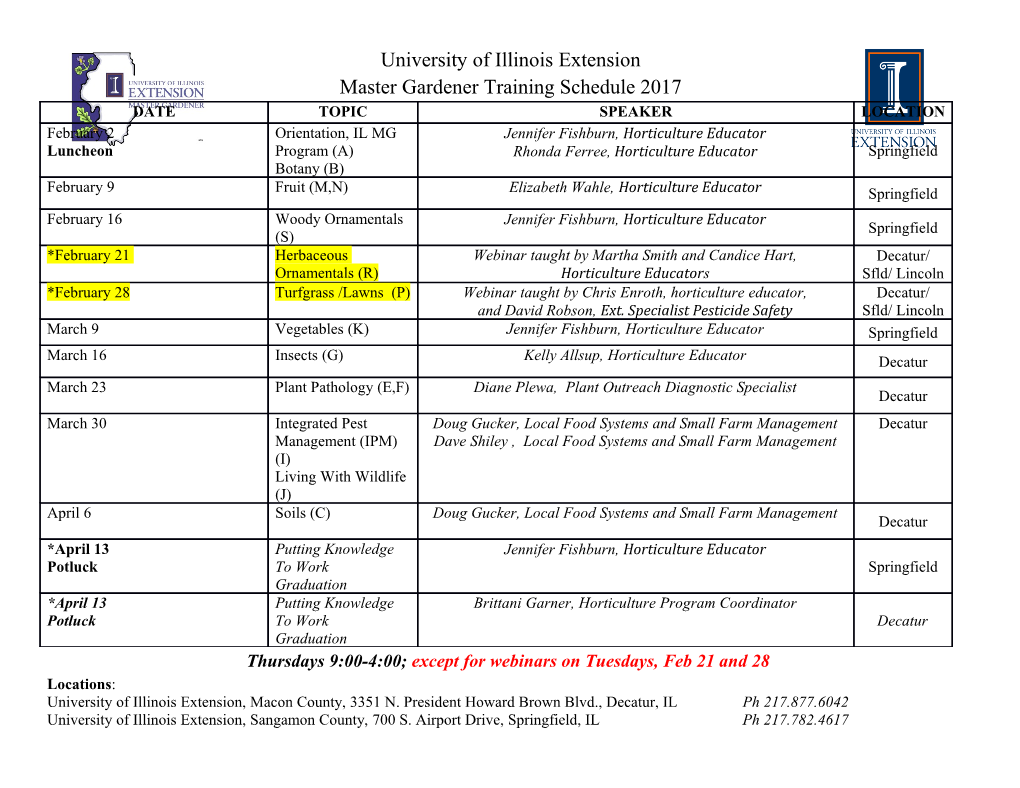
Bayes factors P values Generalized additive model selection References Bayes factors P values Generalized additive model selection References Preface Introducing Bayes Factors There's no theorem like Bayes' theorem Like no theorem we know Leonhard Held Everything about it is appealing Division of Biostatistics Everything about it is a wow University of Zurich Let out all that a priori feeling You've been concealing right up to now! G.E.P. Box 25 November 2011 Music: Irving Berlin (\There's no Business like Show Business") Bayes factors P values Generalized additive model selection References Bayes factors P values Generalized additive model selection References Outline Bayes factors Consider two hypotheses H0 and H1 and some data x. Bayes's theorem implies P(H j x) p(x j H ) P(H ) Bayes factors 0 = 0 · 0 P(H1 j x) p(x j H1) P(H1) | {z } | {z } | {z } Posterior odds Bayes factor Prior odds P values The Bayes factor (BF) quantifies the evidence of data x for H0 vs. H1. BF is the ratio of the marginal likelihoods Generalized additive model selection Z p(x j Hi ) = p(x j θ; Hi ) p(θ j Hi ) dθ | {z } | {z } Likelihood Prior of the two hypotheses H , i = 0; 1. i Bayes factors P values Generalized additive model selection References Bayes factors P values Generalized additive model selection References Properties of Bayes factors Scaling of Bayes factors Bayes factors 1. need proper priors p(θ j Hi ). BF Strength of evidence < 1:1 Negative (supports H ) 2. reduce to likelihood ratios for simple hypotheses. 1 1:1 to 3:1 Barely worth mentioning 3. have an automatic penalty for model complexity. 3:1 to 20:1 Substantial 4. work also for non-nested models. 20:1 to 150:1 Strong 5. are symmetric measures of evidence. >150:1 Very strong 6. are related to the Bayesian Information Criterion (BIC). Bayes factors P values Generalized additive model selection References Bayes factors P values Generalized additive model selection References Jeffreys{Lindley\paradox" About P values Harold Jeffreys (1891-1989) \What the use of P implies [:::] When comparing models with different numbers of is that a hypothesis that may parameters and using diffuse priors, the simpler model be true may be rejected because is always favoured over the more complex one. it has not predicted observable results that have not occurred. ! Priors matter. This seems a remarkable proce- ! The evidence against the simpler model is bounded. dure." Bayes factors P values Generalized additive model selection References Bayes factors P values Generalized additive model selection References About Jeffreys' book\Theory of Probability" Ronald Fisher (1890-1962) \He makes a logical mistake on the first page which invali- dates all the 395 formulae in his book." Bayes factors P values Generalized additive model selection References Bayes factors P values Generalized additive model selection References Steve Goodman's conclusion The Edwards et al. (1963) approach 2 Consider a Gauss test for H0 : µ = µ0 where x ∼ N(µ, σ ). This scenario reflects, at least approximately, many of the statistical procedures found in scientific journals. With T value t = (x − µ0)/σ we obtain p(x j H0) = '(t)/σ. For the alternative hypothesis H1 we allow any prior \In fact, the P value is almost nothing sensible you distribution p(µ) for µ, it then follows that can think of. I tell students to give up trying." Z 1 x − µ p(x j H ) = ' p(µ)dµ ≤ '(0)/σ: 1 σ σ Q: What is the relationship between P values and Bayes factors? This corresponds to a prior density p(µ) concentrated at x. The Bayes factor BF for H0 vs. H1 is therefore bounded: p(x j H ) BF = 0 ≥ exp(−0:5t2) =: BF p(x j H1) Universal lower bound on BF for any prior on µ! Bayes factors P values Generalized additive model selection References Bayes factors P values Generalized additive model selection References The Edwards et al. (1963) approach cont. The Edwards et al. (1963) approach cont. Assuming equal prior probability for H0 and H1 we obtain: Assuming equal prior probability for H0 and H1 we obtain: P value P value 0.05 0.01 0.001 0.05 0.01 0.001 two-sided t 1.96 2.58 3.29 one-sided t 1.64 2.33 3.09 BF 0.15 0.04 0.004 BF 0.26 0.07 0.008 min P(H0 j x) 12.8% 3.5% 0.4% min P(H0 j x) 20.5% 6.3% 0.8% Bayes factors P values Generalized additive model selection References Bayes factors P values Generalized additive model selection References Some refinements by Berger and Sellke (1987) The Sellke et al. (2001) approach Idea: Work directly with the P value p Under H0: p ∼ U(0; 1) Consider two-sided tests with Under H1: p ∼ Be(ξ; 1) with 0 < ξ < 1 1. Symmetric prior distributions, centered at µ0 The Bayes factor of H0 vs. H1 is then 2. Unimodal, symmetric prior distributions, centered at µ0 Z . ξ−1 3. Normal prior distributions, centered at µ0 BF = 1 ξp p(ξ)dξ P value for some prior p(ξ) under H1. Prior 5% 1% 0.1% Calculus shows that a lower limit on BF is Symmetric min P(H0 j x) 22.7% 6.8% 0.9% ( −e · p log(p) for p < e−1 + Unimodal min P(H0 j x) 29.0% 10.9% 1.8% BF = 1 else Normal min P(H0 j x) 32.1% 13.3% 2.4% P value 5% 1% 0.1% min P(H0 j x) 28.9% 11.1% 1.8% Bayes factors P values Generalized additive model selection References Bayes factors P values Generalized additive model selection References Summary A Nomogram for P Values 50 0.5 40 Using minimum Bayes factors, P values can be transformed to 30 1 20 lower bounds on the posterior probability of the null 15 2 hypothesis. 10 5 0.37 5 0.05 It turns out that: 10 0.01 2 20 \Remarkably, this smallest possible bound is by no 1 30 0.001 means always very small in those cases when the 40 0.5 % 50 1e−04 % datum would lead to a high classical significance 60 0.2 70 1e−05 0.1 level. 80 Even the utmost generosity to the alternative hy- 0.05 90 1e−06 0.02 pothesis cannot make the evidence in favor of it as 95 strong as classical significance levels might suggest." 0.01 98 0.005 Edwards et al. (1963), Psychological Review 99 0.002 99.5 0.001 Prior P value Min. Posterior Probability Probability Bayes factors P values Generalized additive model selection References Bayes factors P values Generalized additive model selection References Example: p = 0:03 Q: What prior do I need to achieve p = P(H0 j x)? 50 50 0.5 40 0.5 40 30 30 1 1 20 20 15 15 2 2 10 10 5 0.37 5 5 0.37 5 0.05 0.05 10 10 0.01 2 0.01 2 20 20 1 1 30 0.001 30 0.001 40 0.5 40 0.5 % 50 1e−04 % % 50 1e−04 % 60 0.2 60 0.2 70 70 1e−05 0.1 1e−05 0.1 80 80 0.05 0.05 90 1e−06 90 1e−06 0.02 0.02 95 95 0.01 0.01 98 98 0.005 0.005 99 99 0.002 0.002 99.5 99.5 0.001 0.001 Prior P value Min. Posterior Prior P value Min. Posterior Probability Probability Probability Probability Bayes factors P values Generalized additive model selection References Bayes factors P values Generalized additive model selection References Maximum difference between P(H0) and p = P(H0 j x) Bayesian regression Edwards et al. (two−sided) Consider linear regression model Edwards et al. (one−sided) Berger & Sellke 1 25 2 Berger & Sellke 2 y ∼ N(1β0 + Xβ; σ I) Berger & Sellke 3 Sellke et al. 20 Zellner's (1986) g-prior on the coefficients β 2 2 T −1 15 β j g; σ ∼ N 0; gσ (X X) Jeffreys' prior on intercept: p(β ) / 1 10 0 Jeffreys' prior on variance: p(σ2) / (σ2)−1 5 max difference (in percentage points) max difference The factor g can be interpreted as inverse relative prior sample size. 0 ! Fixed shrinkage factor g=(g + 1). 0.0001 0.0003 0.001 0.003 0.01 0.03 0.1 P value Bayes factors P values Generalized additive model selection References Bayes factors P values Generalized additive model selection References Hyper-g prior in the linear model Model selection in generalized additive regression The problem of model selection in regression is pervasive in statistical practice. Prior with fixed g has unattractive asymptotic properties. Complexity increases dramatically if non-linear covariate ! Hyperprior on g: g=(g + 1) ∼ U(0; 1) effects are allowed for. Parametric approaches: ) Model selection consistency Fractional polynomials (FPs) (Sauerbrei and Royston, 1999) Bayesian FPs (Saban´es Bov´eand Held, 2011a) ) Marginal likelihood p(y) has closed form. Semiparametric approaches: Generalized additive model selection Here we describe a Bayesian approach using penalized splines (joint work with Daniel Saban´es Bov´eand G¨oran Kauermann) Bayes factors P values Generalized additive model selection References Bayes factors P values Generalized additive model selection References Additive semiparametric models Penalised splines in mixed model layout If dj > 1 then Xp T iid 2 f (x );:::; f (x ) = x β + Z u yi = β0 + fj (xij )+ "i ;"i s N(0; σ ); i = 1;:::; n j 1j j nj j j j j j=1 T xj n × 1 covariate vector, zero-centred (1 xj = 0) Effect of xj Functional form Degrees of freedom T T Zj n × K spline basis matrix (1 Zj = xj Zj = 0) 2 not included fj (xij ) ≡ 0 dj = 0 uj K × 1 spline coefficients vector, uj ∼ N(0; σ ρj I) linear fj (xij )= xij βj dj = 1 T Variance factor ρj corresponds to degrees of freedom smooth fj (xij )= xij βj + zj (xij ) uj dj > 1 T −1 −1 T dj = trf(Z Zj + ρj I) Z Zj g + 1 Degrees of freedom vector d = (d1;:::; dp) determines the model.
Details
-
File Typepdf
-
Upload Time-
-
Content LanguagesEnglish
-
Upload UserAnonymous/Not logged-in
-
File Pages10 Page
-
File Size-