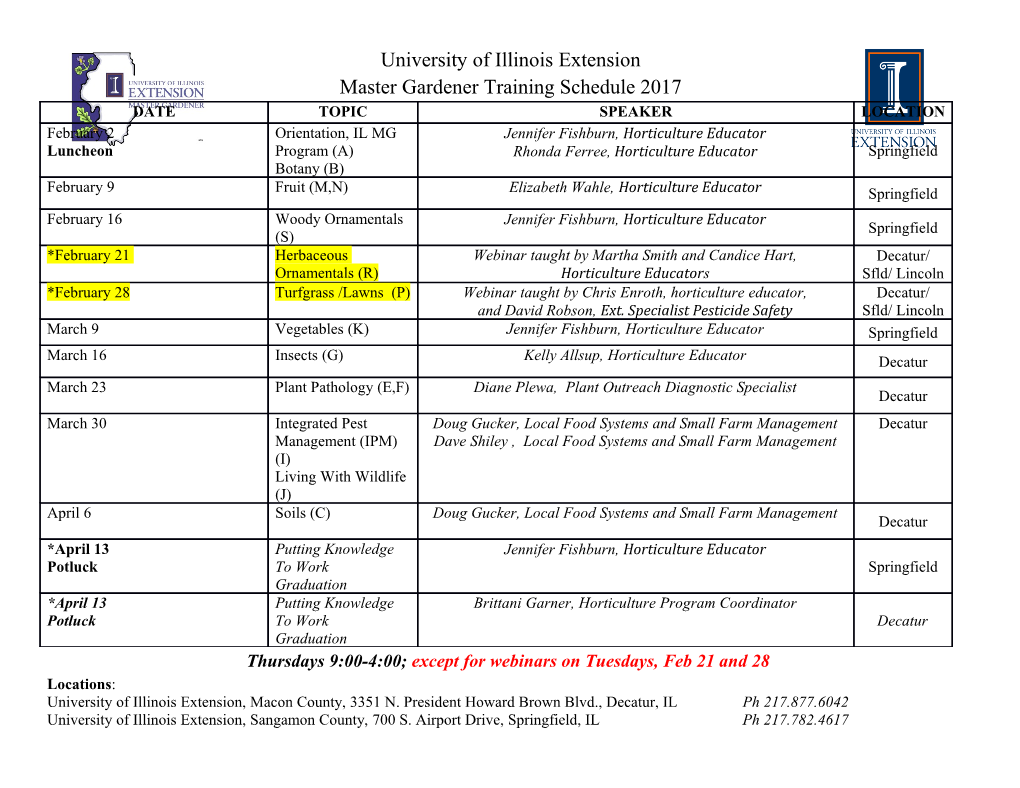
www.nature.com/scientificreports OPEN Using mechanistic models for the clinical interpretation of complex genomic variation María Peña-Chilet1,2, Marina Esteban-Medina1, Matias M. Falco1,2, Kinza Rian1, Marta R. Hidalgo3, Carlos Loucera 1 & Joaquín Dopazo 1,2,4* The sustained generation of genomic data in the last decade has increased the knowledge on the causal mutations of a large number of diseases, especially for highly penetrant Mendelian diseases, typically caused by a unique or a few genes. However, the discovery of causal genes in complex diseases has been far less successful. Many complex diseases are actually a consequence of the failure of complex biological modules, composed by interrelated proteins, which can happen in many diferent ways, which conferring a multigenic nature to the condition that can hardly be attributed to one or a few genes. We present a mechanistic model, Hipathia, implemented in a web server that allows estimating the efect that mutations, or changes in the expression of genes, have over the whole system of human signaling and the corresponding functional consequences. We show several use cases where we demonstrate how diferent the ultimate impact of mutations with similar loss-of-function potential can be and how the potential pathological role of a damaged gene can be inferred within the context of a signaling network. The use of systems biology-based approaches, such as mechanistic models, allows estimating the potential impact of loss-of-function mutations occurring in proteins that are part of complex biological interaction networks, such as signaling pathways. This holistic approach provides an elegant alternative to gene-centric approaches that can open new avenues in the interpretation of the genomic variability in complex diseases. Te extraordinarily fast increase in throughput of sequencing technologies in the last decade1,2 has fostered dif- ferent international collaborative projects3–5 that resulted in an unprecedented increase in our knowledge of the mutational spectrum of diseases, especially thosewith signifcant morbidity and mortality and caused by highly penetrant (typically protein-coding) variants6,7. However, in addition to the expected pathogenic variation, these projects have revealed an unanticipated amount of variation at genome level in apparently normal, healthy individuals. Actually, putative loss-of-function (pLoF) variants, with a potential severe efect on the function of human protein-coding genes8 seems to be surprisingly pervasive, according to reports from diferent genome sequencing projects3,4,9. Conservative estimates suggest that there are more than 250 pLoF variants predicted to be highly damaging10 per sequenced genome, in protein coding regions8, as well as other non-coding regions, such as miRNAs11, transcription factor binding sites12 and others13. Terefore, a better understanding on the contribution of pLoF to disease is critical for clinical applications of genomic data14. From a historical perspective, the application of sequential heuristic flters, in a process called prioritization, has demonstrated to be a useful tool for the clinical interpretation of genomic variation in rare Mendelian dis- eases6,7. Tus, extensively used fltering criteria are: (i) the potential impact of the variant in the resulting gene product, estimated by diferent indexes that predict the potential pathologic efect of an amino acid substitution (e.g. Polyphen15, SIFT16, SNPefect17, PMUT18, etc.) that can be combined with allelic frequency, conservation, etc. (e.g. PROVEAN19, PupaSNP20, CONDEL21, VAAST22, MutationTaster23, etc.); (ii) variant population frequen- cies, given that variants with a relatively high frequency in the population are unlikely to be causative of many hereditary disorders (obtained from diferent repositories such as the 1000 genomes3, the Exome Aggregation Consortium24, the gnomAD25, or also from local population repositories, which have demonstrated to be useful 1Clinical Bioinformatics Area. Fundación Progreso y Salud (FPS). CDCA, Hospital Virgen del Rocío, 41013, Sevilla, Spain. 2Bioinformatics in RareDiseases (BiER). Centro de Investigación Biomédica en Red de Enfermedades Raras (CIBERER), FPS, Hospital Virgen del Rocío, 41013, Sevilla, Spain. 3Bioinformatics and Biostatistics Unit, Centro de Investigación Príncipe Felipe (CIPF), 46012, Valencia, Spain. 4INB-ELIXIR-es, FPS, Hospital Virgen del Rocío, Sevilla, 42013, Spain. *email: [email protected] SCIENTIFIC REPORTS | (2019) 9:18937 | https://doi.org/10.1038/s41598-019-55454-7 1 www.nature.com/scientificreports/ www.nature.com/scientificreports for this purpose26); (iii) evolutionary conservation (e.g. PhyloP27, GERP28, etc.); (iv) compendiums of diferent cri- teria, such as CADD29 or, more recently, based on artifcial intelligence30. Tese flters can also be used in combi- nation with knowledge on functional labels31, syndromes and phenotypes32, or diseases33–35, previously associated to the most likely candidate genes, as implemented in tools such as Phen-Gen36, eXtasy37, PhenIX38, Exomiser39, etc. Diferent computer applications, such as Annovar40, the Variant Efect Predictor41 or the CellBase42, collect all this information that is subsequently used by diferent web interfaces that allow carry out this prioritization interactively, likeOVA43, BiERapp44, QueryOR45 or Mutation Distiller46, etc. Despite success of gene centric clinical interpretation of variation in fnding disease genes in single gene Mendelian disorders7,47,48, the application of these concepts to complex diseases has produced more modest results49. Contrarily to the case of RDs, complex diseases are characterized by phenotypic heterogeneity, that is, patients with similar presentations ofen have diferent underlying disease mechanisms, and incomplete pene- trance, as a consequence of its multigenic nature and the signifcant role of the environment50–52. However, the most widely used tools for the interpretation of genetic variation all focus on monogenic genetic models or, on oligogenic ones as much. As a matter of fact, complex, multigenic diseases can be better understood as failures of functional mod- ules caused by diferent combinations of perturbed gene activities rather than by the failure of a unique gene53. Actually, the idea of cell functionality as a result of the complex interactions between their molecular components is not new54 and was proposed almost two decades ago in the context of systems biology55. Tese interacting components defne operational entities or modules to which diferent elementary functions can be attributed. Tis modularity, extensively described in numerous reports56,57, suggests that causative genes for the same disease ofen reside in the same biological module, which can be a protein complex or any type of biological network58,59. Currently, a detailed recapitulation of the knowledge on biological networks that account for cell functionality, metabolism and other cell processes is available in diferent pathway repositories such as the Kyoto Encyclopedia of Genes and Genomes (KEGG)60, Reactome61, Pathway Commons62, Wikipathways63 and others, including path- ways with specifc, curated descriptions of disease mechanisms64. Since pathways describe how proteins interact among them to trigger cell functionalities or to generate diferent molecules and metabolites, mathematical mod- els of the activity of such pathways constitute ultimately mechanistic descriptors of the behavior of the cell. In fact, recent reports demonstrate that mechanistic models of the activity of metabolic or signaling pathways, render highly precise predictions of complex phenotypes, such as patient survival65,66, drug response67, etc. An interesting property of mechanistic models is that, in addition to study molecular mechanisms of disease in a particular condition, they can be used to predict the potential consequences that perturbations (mutations or changes in the expression) of the proteins that compose the pathway can have over the individual circuits that trigger cell actions or the production of metabolites68,69. Tis mechanistic view of the efects of a change in the integrity or the activity of one or several proteins within the context of signaling66 or metabolic70 pathways can be used to understand the functional consequences of pLoF mutations and/or gene expression perturbations, thus providing a clinical interpretation for these variations in complex scenarios that takes into account the whole context of the impact of the perturbation from a functional angle. Here we present a web server that implements a version of the Hipathia66, an algorithm that has recently been shown that outperforms other current competing algorithms71, and demonstrate the advantages of mechanis- tic models in the interpretation of complex variability in two scenarios of diferent complexity: a rare disease, Fanconi anemia (FA) and a common disease, diabetes. Implementation Overview. Te general idea for the interpretation of complex variability is based on the use of an algorithm that uses gene expression (as a proxy of the presence of the corresponding protein within a pathway) to model the activity of signaling circuits defned within signaling pathways, which ultimately provide a hint on functional cell activity. A functional assessment of the diferences in functional cell behavior between two conditions can be achieved by com- paring the corresponding signaling circuit activity profles to detect what circuits behave
Details
-
File Typepdf
-
Upload Time-
-
Content LanguagesEnglish
-
Upload UserAnonymous/Not logged-in
-
File Pages12 Page
-
File Size-