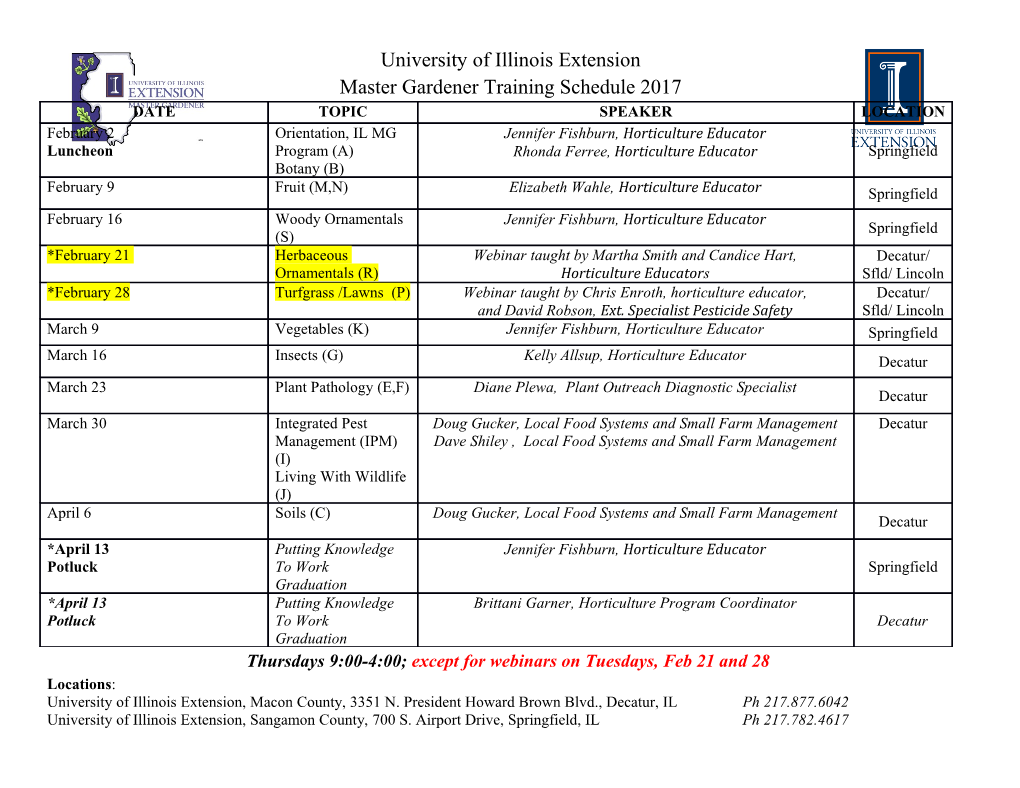
Capturing Covertly Toxic Speech via Crowdsourcing Jorge Nario Alyssa Lees Google Jigsaw [email protected] [email protected] Ian D. Kivlichan Daniel Borkan Nitesh Goyal Jigsaw Jigsaw Jigsaw [email protected] [email protected] [email protected] Abstract Previous works have published baseline toxic- ity detection datasets (Borkan et al., 2019; Wul- We study the task of labeling covert or veiled toxicity in online conversations. Prior re- czyn et al., 2016) along with models for detecting search has highlighted the difficulty in creat- such content (Jigsaw, 2017; Zampieri et al., 2019; ing language models that recognize nuanced Schmidt and Wiegand, 2017; Pavlopoulos et al., toxicity such as microaggressions. Our in- 2020; Georgakopoulos et al., 2018). vestigations further underscore the difficulty However, (Han and Tsvetkov, 2020) recently in parsing such labels reliably from raters via crowdsourcing. We introduce an initial established that covert or veiled toxicity is over- dataset, COVERTTOXICITY, which aims to whelmingly misclassified by leading models. Per- identify and categorize such comments from spective, a free API that uses machine learning to a refined rater template. Finally, we fine- score comments for toxicity, is widely used to aid tune a comment-domain BERT model to clas- content moderators on online platforms (Jigsaw, sify covertly offensive comments and compare 2017). As example, the deeply offensive comment against existing baselines. masked as humor “What’s the difference between 1 Introduction boy scouts and Jews? Boy scouts come back from camp.” is assigned a toxicity probability score Warning: this paper contains content that may be of 0.31 by Perspective. Such comments can be offensive or upsetting. as harmful to participants as more overt toxicity Tools identifying toxic content are essential for (Nadal et al., 2014). moderation of problematic online conversations Covert toxicity is an umbrella term which in- (Jigsaw, 2018). Generally, “toxic” is an umbrella cludes types of toxicity that may not be imme- label for various types of disrespectful comments, diately obvious. Covertly toxic comments may including but not limited to identity attacks, pro- use obfuscation, code words, suggestive emojis, fanity and threats, that could encourage a user to dark humor, or sarcasm. It also includes subtle ag- leave a conversation. Besides, ”toxicity”, other gression, such as the user comment “‘slurp slurp”, terms like ”hate-speech”, and ”online violence” says Chang’, which contains a stereotype regard- have also been used to refer to similar problems ing Asian individuals. Microaggressions may be (Chandrasekharan et al., 2017) - yet no single con- unintentional but can have profound psychological clusive definition exists. In this work, we chose to repercussions in aggregate (Sue et al., 2007a; Jack- use ”toxicity” because our research suggests that son, 2011; Sue et al., 2007b; Stryker and Burke, ”toxicity” is an easy concept for annotators to un- 2000). derstand, meaning we can gather opinions from a diverse range of people, allowing us to capture the In this work, we seek to close the gap in toxicity inherent subjectivity of the concept. In other words, models. Our two main contributions include: it was easier for more people to agree on what con- • A custom crowd-sourcing instruction template stituted “toxic” speech—comments likely to make to identify covert toxicity someone leave a conversation—than it was for peo- • A benchmark dataset for identifying covert ple to agree on other terms to describe problematic toxicity. comments.1 • An enhanced toxicity model with improved 1https://jigsaw.google.com/the-current/toxicity/ performance in covert/veiled toxicity. 14 Proceedings of the First Workshop on Bridging Human–Computer Interaction and Natural Language Processing, pages 14–20 April 20, 2021. ©2021 Association for Computational Linguistics 2 Related Work • Sarcasm/Humor Offensive content in the context of a joke Ogbonnaya-Ogburu et al.(2020) provides a call for • Masked Harm Implied harm or threats race-conscious efforts within the human-computer masked by seemingly inoffensive language interaction community. For example, Dosono and Semaan(2019) argues that Asian American Pacific 3.1 Crowdsourcing Data Collection Islander online community members need support. Further Aroyo et al.(2019) points out that the per- We iterated on versions of an instruction templates ceived toxicity of a comment is influenced by a va- to assist raters in identifying covertly toxic lan- riety of factors not limited to cultural background, guage with high precision. Previous work by Hube rater bias, context and cognitive bias. (2020) on biases and crowdworker annotations, il- In particular, some of these efforts include explo- luminated relevant findings for the templates: rations of microaggressions in social media posts • Ask crowdworkers to reflect and put them- (Breitfeller et al., 2019; Dosono and Semaan, 2019) selves in the shoes of participant with disadvantaged groups, surfacing contextual • Encourage crowdworkers to critically think hate speech or words (Taylor et al., 2017) that about the task prior to engagement. have alternate meanings or veiled toxicity like code- words, adversarially generated or novel forms of As such, two priming questions, see Table 3.1, were offense (Jain et al., 2018), and exploring humor added in the final template. Note to improve rater used as a tool for propagating hate (Billig, 2001). comprehension we refer to covert and overt toxicity Small datasets exist (Breitfeller et al., 2019) as implicit and explicit, respectively.See Figure1 specifically for exploring microaggressions in on- for an example. line conversations. The Social Bias Inference Overtly Offensive Rule Corpus (SBIC), a dataset of social media posts Overt offenses occur when text has some with structured annotations of thousands of demo- words that are clearly toxic and requires no graphic groups (Sap et al., 2019) is another exam- hidden meaning interpretation. ple. To filter such datasets for veiled toxicity, Han Can you think of a word/phrase that is and Tsvetkov(2020) proposed a procedure using clearly spelled or mis-spelled using toxic probing examples. vocabulary, or is threatening? Our paper builds on previous work with a strat- egy for consistently rating covertly toxic content, Covertly Offensive Rule a shared dataset for training, and a baseline covert Covert offenses occur when text has some toxicity model. words that might have hidden meanings or relevance to a community. 3 Covert Toxicity Can you think of a community or members In order to formulate a covertly toxic dataset, we of a community that might be targeted by first extracted a set of candidate comments from the use of words/phrases or might find text CivilCommentsIdentities dataset (Borkan et al., derogatory without explicitly calling out 2019) as described in Section 4.1. We manually la- on them? beled a random sample of these comments, seeking those which could have a negative impact on online The addition of priming questions significantly conversations, but were frequently missed by raters. improved rater performance. In an initial iteration, From this sample, we defined broad categories of a test question of the overtly toxic comment “Peter covert toxicity types. See Figure1 for an example. is an idiot”, had a 43% miss rate by raters. The • Microaggression Subtle discrimination to- miss rate dropped to 5% with priming questions. wards an identity group However, raters still frequently missed com- • Obfuscation Hidden toxicity via intentional ments containing stereotypes, identity attacks and misspellings, coded words, or implied refer- veiled threats. As such, we reconfigured the tem- ences plate instructions (see Figure1) to include a table • Emoticons/Emojis Toxic usage of non-text outlining different types of toxicity with examples. symbols Desired rater accuracy again improved. 15 (b) B. Template for labeling covert toxicity (a) A. Instructions for identifying implicitly (covertly) toxic comments versus explicit toxicity Figure 1: Rater Instructions and Template Rater Classification Count % cific identity terms (e.g. gender, religion, or sexual Not Sure 4457 1.4 orientation). Overtly Offensive 34094 10.8 A limitation of using this dataset is that the com- Covertly Offensive 120314 38.1 ments are directly targeted towards news related Not Offensive 157259 49.7 content. As such, this work should not be gener- alized for other types of online forums, such as Table 1: COVERTTOXICITY rater scores. 4Chan, which may contain vastly different content and context. 4 Datasets For the COVERTTOXICITY dataset, we applied The COVERTTOXICITY2 dataset consists of the methodology of (Han and Tsvetkov, 2020) to covertly offensive rater labels using the described the CivilCommentsIdentities set: comments with template on a subset of CivilCommentsIdentities identity attack annotations and low Perspective API data (Borkan et al., 2019). toxicity scores (Jigsaw, 2017) were marked as can- didates for covert toxicity. 4.1 Training Data The CivilCommentsIdentities toxicity label is The CivilComments dataset is a publicly available the fraction of raters who voted for the label. Han corpus of ∼1.8 million crowd rated comments and Tsvetkov(2020) noted that comments with labeled for toxicity (Borkan et al., 2019). The veiled toxicity were more likely to have dissent CivilComments dataset is derived from the Civil amongst crowd raters and empirically we observed Comments platform plugin,
Details
-
File Typepdf
-
Upload Time-
-
Content LanguagesEnglish
-
Upload UserAnonymous/Not logged-in
-
File Pages7 Page
-
File Size-