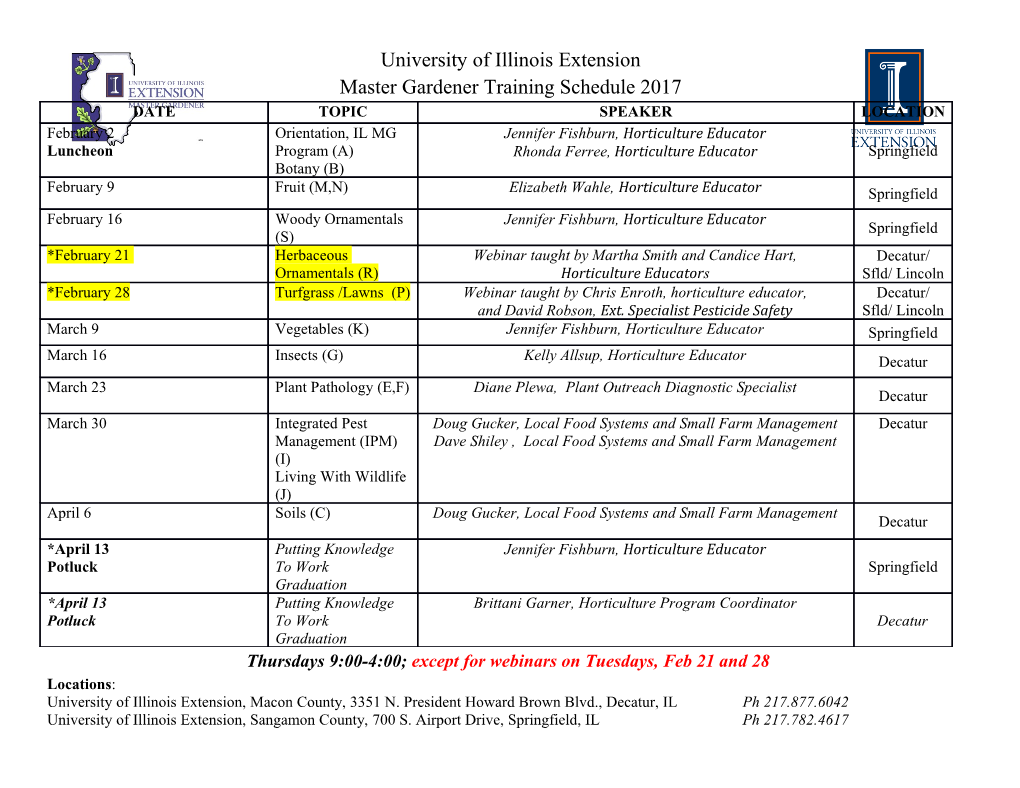
1 Genetic Algorithms in Wireless Networking: Techniques, Applications, and Issues Usama Mehboob, Junaid Qadir, Salman Ali, and Athanasios Vasilakos Abstract—In recent times, wireless access technology is be- his landmark 1950 paper on machine intelligence [5]. Holland coming increasingly commonplace due to the ease of operation used the biological metaphor of chromosomes to refer to and installation of untethered wireless media. The design of strings of binary symbols encoding a candidate solution to wireless networking is challenging due to the highly dynamic environmental condition that makes parameter optimization a the given problem. Holland proposed using the computational complex task. Due to the dynamic, and often unknown, operating analogues of the biological evolutionary processes of random conditions, modern wireless networking standards increasingly mutation, crossover, and natural selection [6] to enable popu- rely on machine learning and artificial intelligence algorithms. lations of chromosomes to get increasingly better at problem Genetic algorithms (GAs) provide a well-established framework solving. The underlying premise is that given enough time, the for implementing artificial intelligence tasks such as classifica- tion, learning, and optimization. GAs are well-known for their process would converge towards a population that contains a remarkable generality and versatility, and have been applied in chromosome (or chromosomes) that solves the given problem. a wide variety of settings in wireless networks. In this paper, Apart from the mutation and crossover operators, The design we provide a comprehensive survey of the applications of GAs in of a GA framework also involves other design issues such wireless networks. We provide both an exposition of common GA as genetic representation (encoding), population initialization, models and configuration and provide a broad ranging survey of GA techniques in wireless networks. We also point out open fitness function formulation, and a selection mechanism. More research issues and define potential future work. While various recently, researchers have also proposed the use of altruistic surveys on GAs exist in literature, our paper is the first paper, techniques in an evolutionary framework that involve cooper- to the best of our knowledge, which focuses on their application ation as a fundamental primitive [7]. in wireless networks. A. Motivations for using GA I. INTRODUCTION 1) Generality and Versatility: GAs apply in a wide variety The study of nature is a rich source of inspiration for of settings and can be easily molded to particular problems. researchers working in the area of artificial intelligence and GAs constitute a very general meta-heuristic technique which machine learning. In particular, the human brain and the can be thought of as the sledgehammer of the craft of algo- biological process of evolution have helped develop and guide rithms, much like the technique of artificial neural networks research in the neural network, and the evolutionary algorithms (ANNs). In particular, GAs can be readily invoked in areas communities. A genetic algorithm (GA) is a metaheuristic that do not yield readily to standard approaches, or when computational method [1], inspired from biological evolution more specialized techniques fail. GAs are capable of solving [2], that aims to imitate the robust procedures used by various extremely large problems that have large search spaces. GAs biological organisms to adapt as part of their natural evolution. are very good at navigating through huge search spaces to GAs have been successfully used in fields as diverse as air- heuristically find near optimal solutions in quick time. In ad- craft industry, chip design, computer animation, drug design, dition, GAs can work even when the objective function is not arXiv:1411.5323v1 [cs.NI] 19 Nov 2014 telecommunications, software creation, and financial markets exactly known since GAs rely only on an objective function’s [3]. evaluation (without necessarily knowing the objective function The field of genetic algorithms (GA) was established by explicitly). Although GAs do not guarantee optimality, GAs John Holland who investigated the evolution of complex are generally useful in practice. Bhandar et al. [8] showed that adaptive systems (CAS) comprising interacting genes, starting although GAs do not guarantee convergence to an optimal in the early 1960s, and culminating in the publication in solution, GAs avoid local optimas with a high probability 1975 of his seminal book on this topic [4]. The idea of through the use of mutation and crossover operators. embedding computers with learning inspired from evolution goes back even further as Alan Turing proposed a “learning 2) Adaptiveness and Online Problem Solving: To under- machine” which would parallel the principles of evolution in stand adaptivity in changing conditions, we will initially develop the idea of a ‘landscape’ both as a metaphor (in which Usama Mehboob ([email protected]), Junaid Qadir (ju- we visualize ourselves as climbing a landscape in pursuit of [email protected]), and Salman Ali ([email protected]) are with the Electrical Engineering Department at the School of Electrical the highest peak) and as a mathematical object (in which the Engineering and Computer Science (SEECS), at the National University of value of the function maps to the elevation of the landscape). Sciences and Technology (NUST), Pakistan. Junaid Qadir is an Assistant Pro- Building upon the metaphor of fitness landscapes, and the fessor at SEECS, NUST. Athanasios Vasilakos ([email protected]) is a Professor of Computer Engineering at the University of Western Macedonia, insight of GAs as stochastic search algorithms, two landscape Greece. models of relevance to optimization through GAs have been 2 proposed by Page [9]. Rugged landscapes are landscapes in the high performing basic building blocks. which there are many peaks, valleys, and troughs (and not 4) Parallel Nature and Scalability: Traditional theory of a single peak). Rugged landscapes assume that the fitness GAs presumes that GAs accomplish the culmination by dis- levels do not change; in evolutionary systems, however, the covering, emphasizing, and recombining the good traits of fitness function is also dependent on context, and on the chromosomes in a vastly parallel manner. GAs incorporate behavior of other competitors. This can be captured by the multiple solutions together in a ‘population’ of solutions. GAs metaphor of dancing landscapes—which are adapted from use evolutionary techniques to test and improve the solutions rugged landscapes. When a landscape ‘dances’, local peaks by using techniques such as mutation, crossover, selection, may change, making a solution that was earlier optimal no and recombination. The parallel nature of GAs renders it as a longer a peak. suitable optimization framework for scalable problem solving Traditional techniques from optimization theory assume in a wide range of applications. static and well-known topologies and are not suited to dynamic environments. In many problems of practical interest, our 5) Support For Multiobjective Optimization: Many prac- focus is more on satisficing (i.e., finding a sufficiently good tical problems in wireless networks require optimizing for solution that satisfies one’s purposes) rather than on optimizing multiple parameters (that may potentially conflict with each (i.e., finding the best possible solution). A key benefit of other). An important characteristic of GAs is that it can easily GAs is that they are well suited to the optimization task in support joint optimization of multiple objectives. Support for the dancing landscapes that characterize wireless networks (in multi-objective optimization—in which the considered objec- which there is interaction between the various adaptive wire- tives may potentially conflict with each other—is of significant less nodes and interdependency between the various node’s practical interest in wireless networks. Issues related to multi- decisions). GAs are well suited for building metaheuristic objective optimization are introduced in [10]. adaptive algorithms that can provide satisfactory performance 6) Support for Global Optimization: Unlike network mod- in changing network conditions. els such as the multi-layered perceptrons (which make local Another important aspect of GAs is that it is an online changes so as to find the local minima), GAs are suited to adaptive algorithm that that can operate in unknown environ- finding the global optima due to a number of properties: ments in an online fashion. Such an ability is crucial in various • They search by means of a population of individuals. control settings in wireless networks in which decisions have • They work with an encoding of the multiple parameters. to be made automatically in run-time to cater to dynamic chan- • They use a fitness function that does not require the nel parameters. In dynamic channel conditions that typically calculation of derivatives. exist in most wireless networking configurations, optimization • They search probabilistically. is an elusive moving target since the optimal solution keeps changing as the conditions change. In online adaptive opti- 7) Easy Implementation: GAs are computationally sim- mization algorithms, there is a fundamental tradeoff between pler compared to other complementary AI techniques such exploration—which involves looking for potentially better as neural networks
Details
-
File Typepdf
-
Upload Time-
-
Content LanguagesEnglish
-
Upload UserAnonymous/Not logged-in
-
File Pages27 Page
-
File Size-