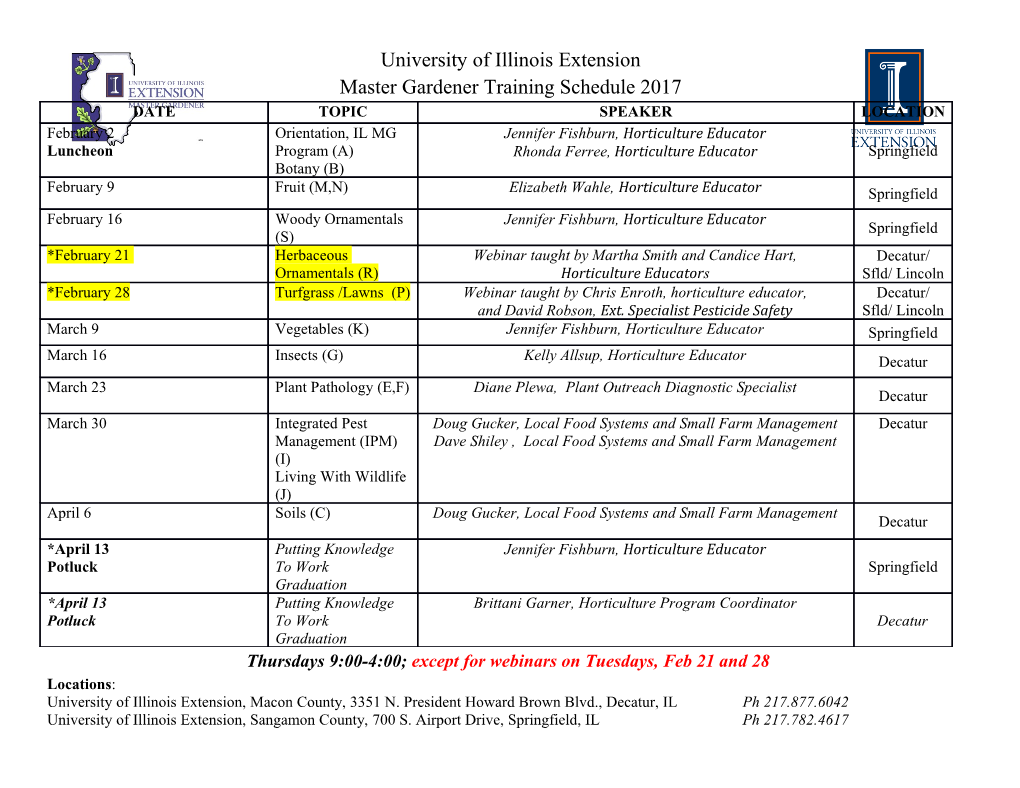
AUTO-ADAPTIVE RESONANCE EQUALIZATION USING DILATED RESIDUAL NETWORKS Maarten Grachten Emmanuel Deruty Alexandre Tanguy Contractor for Sony CSL Paris, France Sony CSL Paris, France Yascore, Paris, France ABSTRACT The use of machine learning, in particular neural net- works, to solve audio production related tasks is recent. In music and audio production, attenuation of spectral res- Automatic mixing tasks that have been addressed in this onances is an important step towards a technically correct way include automatic reverbation [6], dynamic range result. In this paper we present a two-component system compression [18], and demixing/remixing of tracks [17]. to automate the task of resonance equalization. The first To our knowledge, there is no documented example of the component is a dynamic equalizer that automatically de- use of neural networks for automatic equalization. tects resonances, to be attenuated by a user-specified fac- A specific form of equalization used both in mixing and tor. The second component is a deep neural network that mastering is the attenuation of resonating or salient fre- predicts the optimal attenuation factor based on the win- quencies, i.e. frequencies that are substantially louder than dowed audio. The network is trained and validated on em- their neighbors [2]. Salient frequencies may originate from pirical data gathered from a listening experiment. We test different phenomena, such as the acoustic resonances of a two distinct network architectures for the predictive model physical instrument or an acoustic space. They are consid- and find that an agnostic network architecture operating ered a deficiency in the sense that they may mask the con- directly on the audio signal is on a par with a network tent of other frequency regions. One particular difficulty architecture that relies on hand-designed features. Both in resonance attenuation (RA) is finding the right amount architectures significantly improve a baseline approach to of attenuation. For example, too much attenuation may un- predicting human-preferred resonance attenuation factors. mask noise that would otherwise remain unheard, or flatten the spectrum to the point of garbling the original audio. 1. INTRODUCTION AND RELATED WORK The subject of this paper is the automation of the RA process using machine learning. We limit our study to Equalization is part of the audio mixing and mastering neural networks as the state of the art machine learning process. It is a redistribution of the energy of the signal technique. Our method fully automates the RA process. It in different frequency bands. The process has been tra- includes 1) a 0.5s windowed RA process that can be con- ditionally performed by skilled sound engineers or mu- trolled with a single parameter—the resonance attenuation sicians who determine the proper equalization given the factor (RAF), 2) a deep neural network that predicts the at- characteristics of the input audio. Recently methods have tenuation factor from the input audio, making the process been developed for semi-automatic and automatic equal- auto-adaptive [21]. ization. These methods include automatic detection of fre- For the training and validation we conduct a listen- quency resonances [1], equalization derived from expert ing experiment determining optimal RAFs for a set of practices [7], and conformation to a target spectrum [15]. tracks, as chosen by sound engineers. We compare a neu- Equalization profiles may also be derived from semantic ral network architecture that operates directly on the au- descriptors [5]. Appropriate equalization settings can be dio signal to a more traditional approach that includes a found through different means, for example by compar- feature-extraction stage yielding a set of features com- ing the input source to previously equalized content [20], monly used in MIR. Results show that both approaches or by formulating equalization as an optimization problem perform equally well, and significantly outperform a base- where inter-track masking is used as the cost function [10]. line. Some automated equalization functionalities are featured The paper is organized as follows. Section 2 describes in commercial products 1 2 . the RA process. The listening experiment is described in Section 3. The design, training, and evaluation of the pre- 1 www.izotope.com/en/products/mix/neutron 2 www.soundtheory.com/home dictive models is presented in Section 4, and conclusions are presented in Section 5. c Maarten Grachten, Emmanuel Deruty, Alexandre Tan- guy. Licensed under a Creative Commons Attribution 4.0 International 2. RESONANCE EQUALIZATION License (CC BY 4.0). Attribution: Maarten Grachten, Emmanuel Deruty, Alexandre Tanguy. “Auto-adaptive Resonance Equalization us- Traditionally RA has been a manual task where a sound ing Dilated Residual Networks”, 20th International Society for Music In- engineer determines the resonating frequencies by ear or formation Retrieval Conference, Delft, The Netherlands, 2019. using a graphical tool, in order to reduce the energy of the 405 Proceedings of the 20th ISMIR Conference, Delft, Netherlands, November 4-8, 2019 s Corrected PCM Signal a PCM Signal the experimental design of the listening test it proved un- practical to ask subjects to set a varying RAF. Therefore we r inverse DFT b DFT chose relatively homogeneous music fragments (excluding transitions between different sections of songs) and asked q Corrected c Power Spectrum p Power Spectrum subjects for a single attenuation factor for the whole frag- ment, ensuring relatively homogeneous sound fragments. n d Equal Loud- o Spectrum Log-to-linear/freq- ness Weighting Correction interpolation 3.1 Participants e ELC-weighted m Power Spec- l Attenuation A group of 15 subjects was recruited for the experiment, Power Spectrum trum Correction Factor around Paris (France). All subjects are recognized pro- k ∗ fessionals in the industry. Nine subjects specialize in stu- f Smoothing j − dio recording (Classic/Jazz/Pop/Rock/Movie Music, audio g post-production), three are experts in live music, and three Smoothed h Positive i Resonance Power Spectrum Difference are composer/music producers. The average age was 32 (min: 24, max: 42). The subjects were recruited and paid Figure 1. Resonance equalization block diagram; White as if they were working on a commercial project. and gray blocks represent data and processes respectively; The green block depicts the single user-controlled param- 3.2 Data eter; The symbols , ∗ and − represent elementwise vec- A set of 150 audio tracks was used for the listening exper- tor/vector multiplication, elementwise scalar/vector multi- iment. The tracks are excerpts from longer pieces, with a plication, and unary negation respectively. mean duration of 46 seconds and a standard deviation of 16 seconds. All tracks were processed using Nugen AMB R128 4 so that they were aligned to the same median loud- signal in those frequencies by an appropriate amount [16]. ness. The set comprised contemporary pop and rock mu- In this section, we describe a procedure that identifies res- sic, as well as film scores. Of this set, 131 tracks were onating frequencies autonomously, and reduces the energy unique recordings, while the remaining 19 tracks were in those frequencies by a factor that is controlled by the variants of some of the unique 131 recordings, with dif- user. The procedure works on overlapping audio windows ferences in mixing. None of the tracks were previously that must be large enough to allow for spectral analysis at mastered. a high frequency resolution. Figure 1 displays a block diagram of the RA process, 3.3 Procedure where each element is denoted by a letter. We will use these letters to refer to the corresponding elements in the The listening experiment took place in a recording studio, diagram. First the audio signal is used to compute a where participants listened to the audio tracks individually, power spectrum weighted by Equal-Loudness Contours using studio monitors, at a measured loudness of 80 dBC– (ELC) [12] at a fixed monitoring level of 80 phon (Fig- a typical listening loudness during audio production. The ure 1, element d) to reflect the perceptual salience of the participants were presented with a web interface in which signal energy at different frequency bands. The value of they could listen to each track with different degrees of 80 phon is chosen in relation to the procedure detailed in RA, ranging from 0 (no attenuation) to 1 in 17 steps. They Section 3.3. The ELC-weighted power spectrum (e) con- could select their preferred degree of RA, or alternatively sists of 400 log-scaled frequency bands. decline to select any version, indicating that none of the Resonances (i) are determined by smoothing ELC- versions sounded acceptable. The tracks were separated weighted power spectrum 3 (e) to obtain (g) and comput- from each other by 10 seconds of pink noise surrounded ing the elementwise differences (e) minus (g), setting nega- by a short silence to give the participants a fixed reference. tive elements to zero (h). The negative of the resonances is Sessions of 50 tracks were alternated with breaks. then scaled by the user defined RAF (l), transformed back to a linear scale and converted back to the shape of the 3.4 Results and discussion original spectrum using interpolation (h). The result (o) is a vector of scaling factors (one per DFT bin). Multiplying Basic statistics of the results per subject are given in Ta- the original spectrum (c) with the scaling factors gives the ble 1. Subject 13 stands out because of the number of corrected spectrum (q) which—by the inverse DFT (r)— missing ratings (21 versus a median of 1 over all subjects). yields the corrected audio signal (s). Subjects 1 and 15 have abnormally high rates of 0.0 rat- ings (72 and 58 respectively, versus a median of 16 over all subjects). Finally, Subject 7 stands out in terms of median 3.
Details
-
File Typepdf
-
Upload Time-
-
Content LanguagesEnglish
-
Upload UserAnonymous/Not logged-in
-
File Pages7 Page
-
File Size-