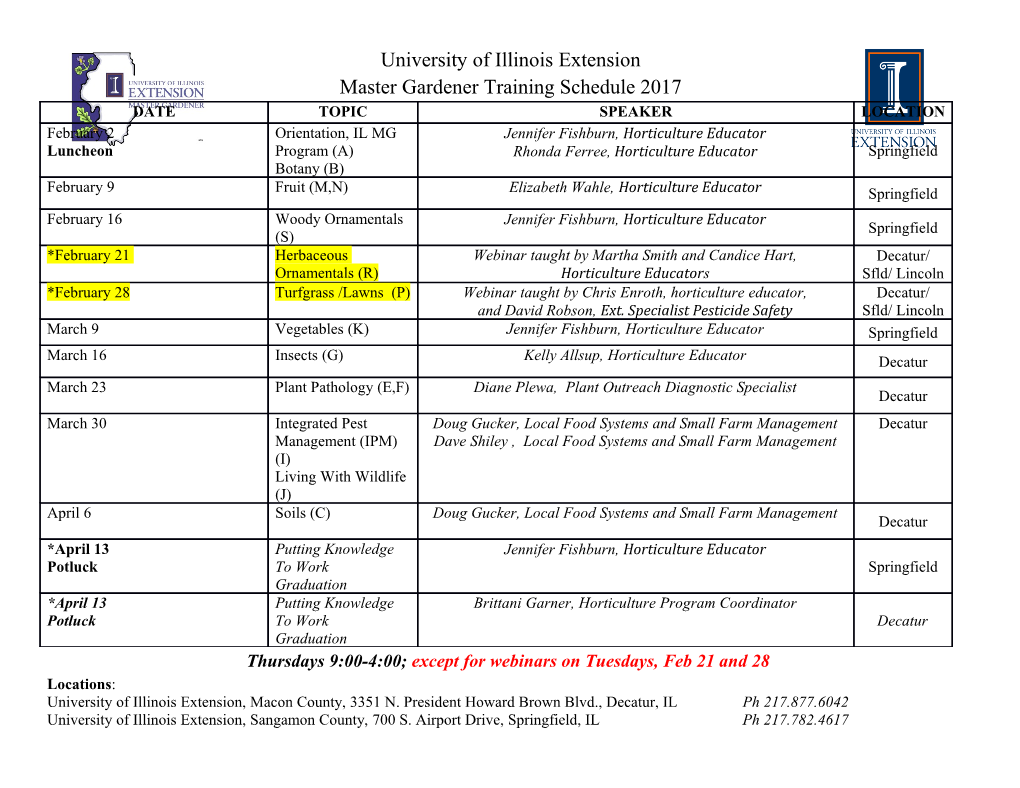
Extending Owen's integral table and a new multivariate Bernoulli distribution Marcelo Hartmann, Phd student1 1Department of Mathematics and Statistics, P.O. Box 68, 00014 University of Helsinki, Finland June 22, 2017 Corresponding author: marcelo.hartmann@helsinki.fi 1 Description The table of Owen (1980) presents a great variety of integrals involving the Gaussian density function and the Gaussian cumulative distribution function. For some of them analytical solution is presented and for some others, the solution is written in terms of the Owen's T -function (Owen, 1980). With little more algebra we can extend many of those equalities in his table (Owen, 1980) and moreover a new multivariate Bernoulli distribution could be found. This extension can be useful in many practical application where quadrature methods have been applied to solve integrals involving Gaussian function and the Owen's T -function, e.g., Riihim¨aki et al. (2013); J¨arvenp¨a¨aet al. (2017); Stathopoulos et al. (2017). 2 Gaussian integral I arXiv:1704.04736v3 [stat.ME] 21 Jun 2017 Lemma 1. Let Φ(·) be the standard-Gaussian cumulative distribution function and 2 2 N (·|µ, σ ) the Gaussian density function with parameters (µ, σ ) 2 R × R+. Then the following holds true, Z N Y x − mr Φ N (xjµ, σ2)dx = F (µ jm ;V ) (1) v N N N N r=1 r R where FN (·|c; C) is the N-dimensional Gaussian cumulative distribution function with parameters (c; C) 2 RN × R, with R the space of positive-definite matrices 1 T N N (covariance matrices). Furthermore, mN = [m1 ··· mN ] 2 R , µN = µ1N 2 R , vr > 0 8r and VN is a covariance matrix given by, 2 2 2 2 3 v1 + σ : : : σ 6 . .. 7 VN = 4 . 5 (2) 2 2 2 σ : : : vN + σ Proof. To show (1), start writing the left-hand side of the equation in full. Rewrite the integrand as the product of non-standard Gaussian density functions as well as the regions of integration, i.e., x x Z Z Z N Y 2 2 ··· N (yrjmr; vr )N (xjµ, σ )dy1 ··· dyN dx: (3) r=1 R −∞ −∞ T Rewrite again using the following transformation [x; y1; ··· ; yN ] = [w + µ, z1 + w + T m1; ··· ; zN +w +mN ] and note that j@(x; y1; ··· ; yN )=@(w; z1; ··· ; zN )j = 1. After changing variables, group the different terms in the exponentials together to have µ−m µ−m N 1 ( " N #) Z Z Z 2 1 1 X (zr+w) w2 ··· exp − 2 + 2 dz1 ··· dzN dw (4) c 2 vr σ r=1 R −∞ −∞ (N+1)=2 QN where c = σ(2π) r=1 vr. Now, the expression inside the squared bracket is a quadratic form which is written with the following matrix form, N N ! N N 2 2 X (zr+w) w 2 X 1 1 X zr X w zr 2 + 2 = w 2 + 2 + w 2 + zr 2 + 2 vr σ vr σ vr vr vr r=1 r=1 r=1 r=1 2 3T PN 1 1 PN zr w 2 + 2 + 2 2 3 r=1 vr σ r=1 vr w 6 7 6 w z1 7 6 z1 7 6 v2 + v2 7 6 7 = 6 1 1 7 6 . 7 6 . 7 6 . 7 6 . 7 4 5 4 5 w zN zN 2 + 2 vN vN 2 3T 2PN 1 1 1 1 3 2 3 w r=1 2 + 2 2 ··· 2 w vr σ v1 vN 6 7 6 1 1 7 6 7 z1 6 v2 v2 ··· 0 7 z1 6 7 6 1 1 7 6 7 = 6 . 7 . 6 . 7 (5) 6 . 7 6 . .. 7 6 . 7 4 5 6 . 7 4 5 4 1 1 5 zN 2 0 ··· 2 zN vN vN therefore (4) is the same as 8 2 3T 2PN 1 1 1 1 3 2 39 > w r=1 v2 + σ2 v2 ··· v2 w > µ−m µ−m > r 1 N > Z Z N Z 1 > 6 7 6 1 1 7 6 7> < 1 z1 6 v2 v2 ··· 0 7 z1 = ··· 1 exp − 6 7 6 1 1 7 6 7 d z dw c 6 . 7 6 . 7 6 . 7 > 2 6 . 7 6 . .. 7 6 . 7> R −∞ −∞ > 4 5 4 5> > 4 1 1 5 > :> zN 2 0 ··· 2 zN ;> vN vN (6) 2 Note that the integrand from (6) does have the full form of the multivariate Gaus- sian density with the specific precision matrix given above. To identify this we need to find the closed-form covariance matrix from the precision matrix and if the determinant of the covariance matrix is given by c2=(2π)N+1. Write the pre- PN 1 1 h 1 1 i cision matrix as block matrix such that A = r=1 2 + 2 , B = 2 ··· 2 , vr σ v1 vN T 1 1 C = B and D = diag 2 ; ··· ; 2 . Use the partitioned matrix inversion lemma v1 vN (Strang and Borre, 1997, equation 17.44) to get the blocks, (A − BD−1C)−1 = σ2,(BD−1C − A)−1BD−1 = −σ2[1 ··· 1], D−1C(BD−1C − A)−1 = −σ2[1 ··· 1]T −1 −1 −1 −1 −1 2 and D + D C(A − BD C) BD where its main diagonal equals to [v1 + 2 2 2 2 σ ; ··· ; vN + σ ] and all off-diagonal elements are given by σ . Put everything to- gether to have the covariance matrix 2 3 σ2 −σ2 · · · −σ2 6 2 2 2 2 7 6−σ v1 + σ ··· σ 7 6 . 7 (7) 6−σ2 . .. 7 4 5 2 2 2 2 −σ σ ··· vN + σ −1 2 QN 2 2 N+1 whose determinant equals to 1=[det(D) det(A−BD C)] = σ r=1 vr = c =(2π) by the partitioned matrix determinant lemma. Finally, in (6), interchange the order of integration with Fubini-Tonelli theorem (Folland, 2013) and integrate w.r.t. w to get 02 3 2 3 2 2 2 2 31 µ−mN µ−m1 z1 0 v1 + σ ··· σ Z Z B6 . 7 6.7 6 . .. 7C ··· N @4 . 5 4.5 ; 4 . 5A dz1 ··· dzN (8) −∞ −∞ 2 2 2 zN 0 σ ··· vN + σ that equals to 02 3 2 3 2 2 2 2 31 µ m1 v1 + σ ··· σ B6 . 7 6 . 7 6 . .. 7C FN @4 . 5 4 . 5 ; 4 . 5A (9) 2 2 2 µ mN σ ··· vN + σ and therefore the equality (1) holds. For N = 1 the result follows the same as in Rasmussen and Williams (2006). Lemma 2. Let Φ(·) be the standard-Gaussian cumulative distribution function and denote by N (·| µN ; Σ) the N-dimensional Gaussian density function with mean pa- rameter µN and covariance matrix Σ. Then the following holds true, Z N Y xr − mr Φ N (x j µ ; Σ)dx = F µ jm ; diag(v )2 + Σ (10) v N N N N N r=1 r RN where FN (·|c; C) is the N-dimensional Gaussian cumulative distribution function T N T with parameters (c; C). Furthermore, mN = [m1 ··· mN ] 2 R , µN = [µ1 ··· µN ] 2 N T N R , vN = [v1 ··· vN ] 2 R+ and Σ is a covariance matrix. 3 Proof. Let's rewrite the left-hand side of (10) in full and use the following transfor- T mation [x1; ··· ; xN ; y1; ··· ; yN ] = [w1 + µ1; ··· ; wN + µN ; z1 + w1 + m1; ··· ; zN + T wN + mN ] . From this we note that the Jacobian of the transformation simplifies to j@ (x1; ··· ; xN ; y1; ··· ; yN ) =@(w1; ··· ; wN ; z1; ··· ; zN )j = 1. Therefore we find that µ −m µ −m Z NZ N 1Z 1 2 ··· N (wj − z; diag(vN ) )N (wj 0; Σ)dzdw (11) RN −∞ −∞ T T where z = [z1 ··· zN ] and w = [w1 ··· wN ] . Note that the product of two mul- tivariate Gaussians is another unnormalized multivariate Gaussian (see Rasmussen and Williams, 2006, for example). Therefore we write µ −m µ −m Z NZ N 1Z 1 2 ··· N (zj 0; diag(vN ) + Σ)N (wjc; C)dzdw (12) RN −∞ −∞ 2 −1 2 −1 −1 −1 where c = −C[diag(vN ) ] z and C = ([diag(vN ) ] + Σ ) . Interchange the order of integration with Fubini-Tonelli theorem (Folland, 2013) and integrate w.r.t w to get that, µ −m µ −m NZ N 1Z 1 2 2 ··· N (zj 0; diag(vN ) + Σ)dz = FN (µN jmN ; diag(vN ) + Σ) (13) −∞ −∞ which completes the proof. Note that for N = 1 the result follows the same as in Rasmussen and Williams (2006). 3 Gaussian integral II T Theorem 1. Let f = [f1 ··· fN ] ∼ N (µ; Σ), where N (·) is the N-dimensional Gaussian density function with mean parameter µ and covariance matrix Σ. Suppose that, conditional on f, we perform N independent Bernoulli trials with probability Φ(fr), r = 1; ··· ;N, where Φ(·) is the standard-Gaussian distribution function, i.e., ind Yrjfr ∼ Bernoulli (Φ(fr)). Instead of record the values 0 or 1 we use the values −1 and 1, so that, each Bernoulli random variable Yrjfr has probability mass function π (y jf ) = Φ(y f )I (y ) (14) Yrjfr r r r r {−1;1g r where IA(·) is the indicator function of a set A. Hence the marginal distribution of T Y = [Y1 ··· YN ] is given by πY (y1; ··· ; yN ) = FN (0 j − Iy µ;IyΣIy + IN ) (15) where Iy = diag(y1; ··· ; yN ), IN is the N × N identity matrix and FN (·|c; C) is the N-dimensional Gaussian cumulative distribution function with mean parameter c and covariance matrix C.
Details
-
File Typepdf
-
Upload Time-
-
Content LanguagesEnglish
-
Upload UserAnonymous/Not logged-in
-
File Pages7 Page
-
File Size-