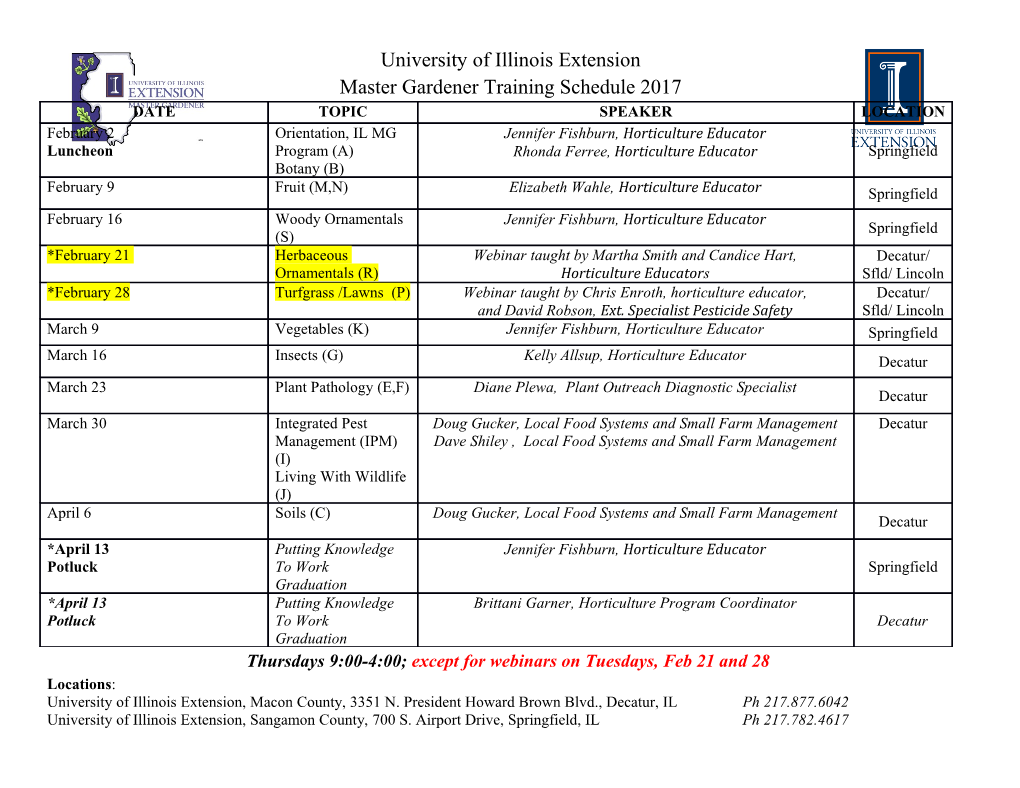
Information Theory for the Analysis of Physiological Time Series - ITS Toolbox v 2.0 ITS Toolbox: A Matlab toolbox for the practical computation of Information Dynamics Version 2.0 – July 2017 Luca Faes BIOtech, University of Trento, Italy www.lucafaes.net [email protected] 1 INTRODUCTION • Network of dynamic processes Observed dynamical system S Yn X S n Y1 Y2 M dynamic processes Y1, Y2,…, YM … YM Z Measured time series: Y1,n,Y2,n,...,YM,n n Y1,1 YM ,1 • Realization: N×M data matrix Y Y1,N YM ,N • Estimation of Information Dynamics: (A) Definition of Measures: univariate, bivariate, multivariate Can be expressed in terms of conditional entropies (B) Approximation of the past history of the processes Embedding procedures: uniform, non-uniform (C) Computation of conditional entropy Entropy estimators: Linear (Gaussian), Binning, Kernel, Nearest Neighbors 2 UNIVARIATE SYSTEM ANALYSIS j TARGET SYSTEM: Yj Time series: Yn Y1 j-th column of the data matrix Y YN • Information generated by Yj: Entropy its_Elin.m its_Ebin.m functions for Entropy: HY H(Yn) p(yn)logp(yn) its_Eker.m its_Eknn.m functions for Self Entropy: • Information Storage in Yj : Mutual Information its_SElin.m SY I(Yn;Yn ) H(Yn) H(Yn |Yn ) its_SEbin.m its_SEker.m New Information: Conditional Entropy its_SEknn.m NY H(Yn |Yn ) S Y HY SY NY NY 3 BIVARIATE SYSTEM ANALYSIS i j TARGET SYSTEM: Yj Time series: Yn j-th column of the data matrix X1 Y1 Y DRIVER SYSTEM: Yi Time series: X n i-th column of the data matrix X N YN • Information Transfer from X to Y : functions for Transfer Entropy: Conditional Mutual Information its_BTElin.m T I(Y ; X |Y ) H(Y |Y ) H(Y | X ,Y ) its_BTEbin.m X Y n n n n n n n n its_BTEker.m its_BTEknn.m New Information: Conditional Entropy NY|X H(Yn | Xn ,Yn ) TX Y HY SY TX Y NY|X SY NY|X H(Yn | Xn ,Yn ) 4 MULTIVARIATE SYSTEM ANALYSIS i j TARGET SYSTEM: Yj Time series: Yn j-th column of the data matrix DRIVER SYSTEM: Yi Time series: Xn i-th column of the data matrix X1 Y1 Y OTHER SYSTEMS: Y\{Yi,Yj} Time series: Zn k-th columns of the data matrix X N YN k Joint Transfer Entropy: • Joint Information Transfer from X,Z to Y : its_BTElin.m T I(Y ; X ,Z |Y ) H(Y |Y ) H(Y | X ,Y ,Z ) its_BTEbin.m XZ Y n n n n n n n n n n its_BTEker.m its_BTEknn.m Partial Transfer Entropy: • Conditional Information Transfer from X to Y given Z: its_PTElin.m T I(Y ; X |Y ,Z ) H(Y |Y ,Z ) H(Y | X ,Y ,Z ) its_PTEbin.m X Y|Z n n n n n n n n n n n its_PTEker.m its_PTEknn.m New Information: NY|X H(Yn | Xn ,Yn ,Zn ) HY SY TXZ Y NY|X ,Z TX Y|Z I XZY TZY|X TXZ Y TX Y|Z TZ Y|X I XZ Y TX Y TZ Y I XZ Y SY TX Y N H(Y | X ,Y ,Z ) I XZ Y TX Y TX Y|Z Y|X ,Z n n n n 5 ESTIMATION: APPROXIMATION OF THE SYSTEM PAST • Uniform embedding (UE): Covers the past of each system with predetermined lagged components, uniformly spaced in time mX mX mX LX=3, mX=5 X X [X X ] n nmX nLX mX mY mY mY LY=3, mY=4 L = embedding dimension m = embedding lag Y Y [Y Y ] n nmY nLY mY Example: L=3, m=1 Vn [Xn1,Xn2,Xn3,Yn1,Yn2,Yn3] • Non-Uniform embedding (NUE): Approximates the system past through a sequential procedure that selects progressively the lagged components according to a criterion for maximum relevance and minimum redundancy lags 1,2 lag 1 Vn [Xn1,Yn1,Yn2] Example: X Y X n Yn 6 ESTIMATION: COMPUTATION OF CONDITIONAL ENTROPY Functions for embedding (common to all estimators): its_SetLag.m Sets the indices for embedding its_buildvectors.m Given the embedding indices, forms the observation matrix B Example: M=2 time series Vn [Xn1,Xn2,Yn1,Yn2] Uniform embedding with L=2, m=1 Data matrix Embedding indices Observation matrix Y3 X 2 X1 Y2 Y1 1 1 X1 Y1 1 2 Vi 2 1 B Yn X n1 X n2 Yn1 Yn2 X Y N M 2 2 YN X N 1 X N 2 YN 1 YN 2 Functions for computing conditional entropy (estimator-specific): its_CElin.m Conditional Entropy from the Observation Matrix • Linear (Uniform Embedding) its_CElinVAR.m Conditional Entropy from the VAR parameters • Binning its_CEbin.m Conditional Entropy from the Observation Matrix its_NUEbin.m Conditional Entropy from the Observation Matrix, Non-Uniform Embedding • Kernel its_CEker.m Conditional Entropy from the Observation Matrix its_NUEker.m Conditional Entropy from the Observation Matrix, Non-Uniform Embedding • Nearest its_CMIknn.m Conditional Mutual Information from the Observation Matrix neighbors its_NUEknn.m Conditional Mutual Information from the Observation Matrix, Non-Uniform Embedding 7 ESTIMATORS: LINEAR-MODEL BASED ESTIMATOR L 1 • Computation based on linear prediction models • Example: L=1 Yn Yn Yn1 Exploits the analytic relation between (conditional) entropy and (prediction error) variance valid for Gaussian processes, and performs linear regression to find the prediction error variance Yn a1Yn1 aLYnL Wn 1 (Y ) 1 2 S ln n ln Y Y L 2 2 (Yn |Yn ) 2 W L L • The estimator uses Uniform Embedding Xn Xn [Xn1XnL], Yn Yn [Yn1YnL] • Analysis parameters: Regression order: L (either imposed or selected with optimization criteria) Main functions: • its_Elin.m System Information, univariate system its_SetLag.m • its_SElin.m Information Storage, univariate system its_buildvectors.m • its_BTElin.m Information Transfer, bivariate system its_CElin.m • its_PTElin.m Conditional Information Transfer, multivariate system its_CElinVAR.m Other functions: • its_LinReg_Ftest.m Statistical significance of Information Dynamics, based on Fisher F-test • its_FindOrderLin.m Selection of regression order, based on Akaike or Bayesian information criteria 8 ESTIMATORS: MODEL-FREE ESTIMATOR BASED ON BINNING • Example: L=1 L 1 Yn Yn Yn1 • Computation based on time series quantization Discretize the values of each variable using quantization levels, then estimate the probability as the relative frequency of visitation of the hypercubes in the multidimensional space spanned by the variables • Non-Uniform Embedding is highly recommended to limit dimensionality • Analysis parameters: Number of quantization levels: c Embedding parameters: L, m (for non-uniform embedding, also parameters for procedure termination) Main functions: • its_Ebin.m System Information, univariate system its_SetLag.m • its_SEbin.m Information Storage, univariate system its_buildvectors.m • its_BTEbin.m Information Transfer, bivariate system its_CEbin.m • its_PTEbin.m Conditional Information Transfer, multivariate system its_NUEbin.m Other functions: • its_quantization.m Uniform quantization of the time series 9 ESTIMATORS: MODEL-FREE ESTIMATOR BASED ON KERNELS L 1 • Example: L=1 Yn Yn Yn1 • Computation based on kernel density estimation Approximate the probability density at each data point by using kernel functions to weight the distance from the reference point to any other point in the time series; If the Heaviside kernel with parameter r is used, the method counts the relative number of points having distance less than r from the reference point, then averages across all points • Non-Uniform Embedding is highly recommended to limit dimensionality • Analysis parameters: Threshold distance: r (usually a fraction of the SD of the time series) Embedding parameters: L, m (for non-uniform embedding, also parameters for procedure termination) Main functions: • its_Eker.m System Information, univariate system its_SetLag.m • its_SEker.m Information Storage, univariate system its_buildvectors.m • its_BTker.m Information Transfer, bivariate system its_CEker.m • its_PTEker.m Conditional Information Transfer, multivariate system its_NUEker.m 10 ESTIMATORS: MODEL-FREE ESTIMATOR BASED ON NEAREST NEIGHBORS L 1 • Computation based on nearest neighbor statistics • Example: L=1 Yn Yn Yn1 Study the probability distribution for the distance between an observation and its k-th neighbor • Compensation of the estimation bias in a sum of entropies by looking for neighbors in the higher dimensional spaces, and counting within ranges in the lower dimensional spaces • Non-Uniform Embedding is highly recommended to limit dimensionality • Analysis parameters: Number of neighbors: k Embedding parameters: L, m (for non-uniform embedding, also parameters for procedure termination) Main functions: • its_Eknn.m System Information, univariate system its_SetLag.m • its_SEknn.m Information Storage, univariate system its_buildvectors.m • its_BTEknn.m Information Transfer, bivariate system its_CMIknn.m • its_PTEknn.m Conditional Information Transfer, multivariate system its_NUEknn.m Other functions: • nn_prepare, nn_search, range_search functions for neighbor search and range search Built-in functions, compiled from C++ and imported from the TSTOOL Toolbox [http://www.physik3.gwdg.de/tstool/] 11 COMPUTATION OF INFORMATION DYNAMICS General procedure for the computation of Information Dynamics Set indices of time series Y Load data matrix Y Compute Entropy Hy Univariate: index of Y Elin,Ebin,Eker,Eknn (normalize time series) Bivariate: indices of X,Y Multivariate: indices of X,Y,Z X,Y,Z Compute Information Dynamics: b) Compute the desired measure a) Set embedding Indices V • Univariate analysis: Information storage Uniform Embedding: L SElin, SEbin, Seker, SEknn SetLag Non-Uniform Embedding: • Bivariate analysis: Information Transfer SetLag + NUEbin BTElin, BTEbin, BTEker, BTEknn SetLag + NUEker • Multivariate analysis: Conditional Information Transfer TX Y|Z SetLag + NUEknn TX Y PTElin, PTEbin, PTEker, PTEknn SY 12 REFERENCES • Theory and generalities about estimation • L Faes, A Porta, 'Conditional entropy-based evaluation of information dynamics in physiological systems', in Directed Information Measures in Neuroscience, R Vicente, M Wibral, J Lizier (eds), Springer-Verlag; 2014, pp. 61-86 • Theory and linear-model based estimation • L Faes, A Porta, G Nollo, 'Information decomposition in bivariate systems: theory and application to cardiorespiratory dynamics', Entropy, special issue on “Entropy and Cardiac Physics”, 2015, 17:277-303.
Details
-
File Typepdf
-
Upload Time-
-
Content LanguagesEnglish
-
Upload UserAnonymous/Not logged-in
-
File Pages19 Page
-
File Size-